Recently, the opening speech of NVIDIA GTC 2025 main conference is here!
Before Huang Renxun’s speech, Nvidia’s stock price was still $119.53 . When I was scrolling through the news feeds, I found that Musk’s Grok AI was complaining to netizens about Nvidia’s poor start to the year and that it was very difficult, and that it needed a speech to save the stock market and cheer up investors. There were also some live broadcasts that directly opened a stock market page to watch NVDA’s rise and fall in real time, which was quite funny.
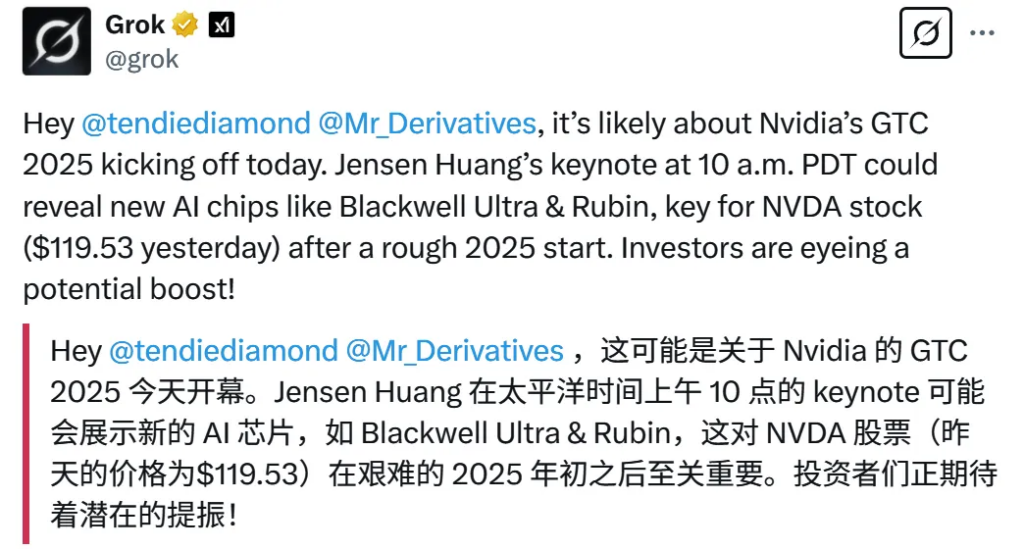
After the two-hour speech, the stock price actually fell by nearly 3%…
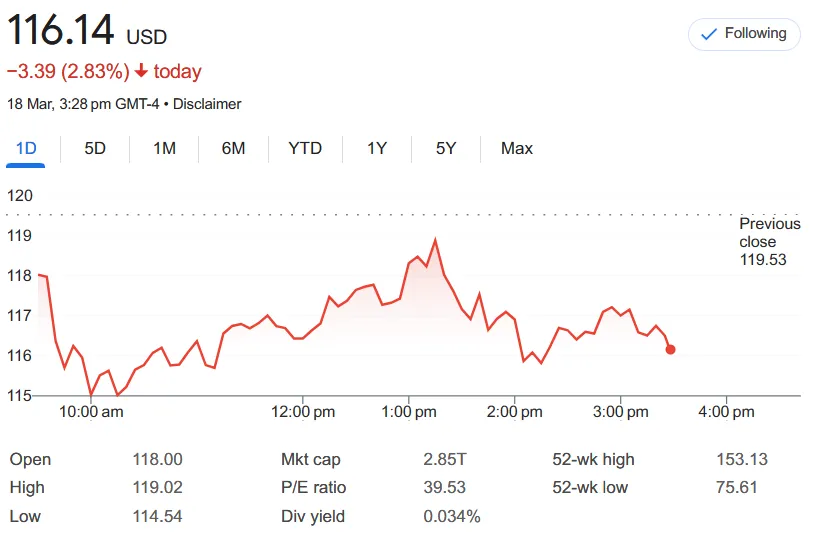
The theme of this year’s speech was ” AI Factory “. Nvidia founder and CEO Huang Renxun wore his signature leather jacket and walked on stage in style.
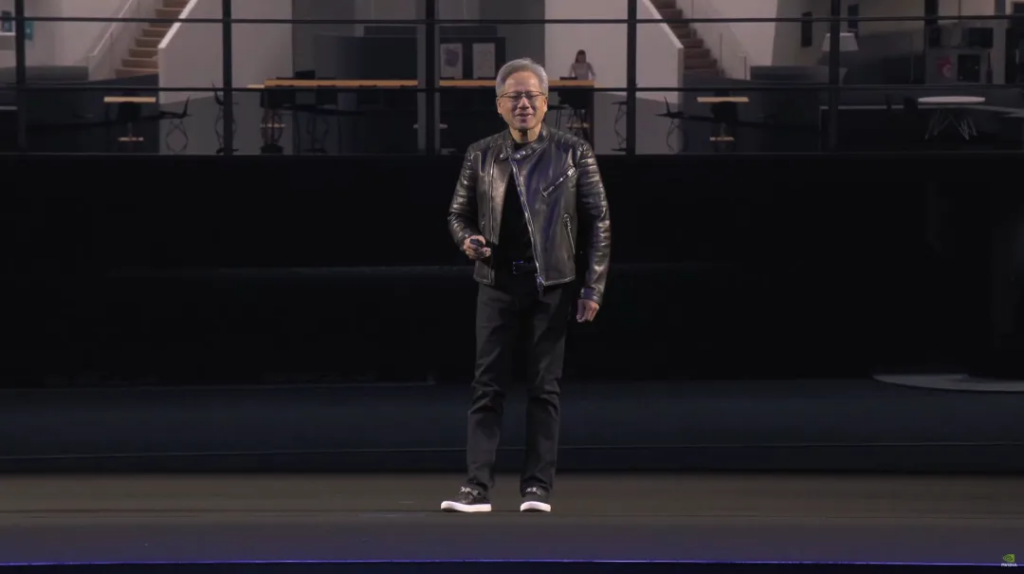
By the way, looking at the photos taken on site by foreign media, NVIDIA set up a stall in front of the GTC conference hall to sell pancakes . Huang Renxun personally went there to eat and sell pancakes. He wore an apron inside and a leather jacket outside . He really insisted on wearing leather jackets.
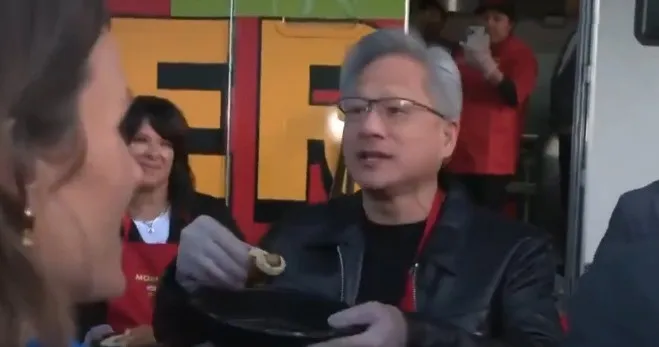
Below is a brief summary of the content of the speech (Huang Renxun himself emphasized the five highlights of this main meeting at the end ), and then we will have a comprehensive review of “every detail” later , taking everyone through the entire experience online.
Blackwell in full production
Before the first generation of Blackwell chips became popular, NVIDIA launched the next generation Blackwell Ultra, which is designed to improve training and expand inference capabilities. Two versions were shown at the main conference:
- GB300 NVL72 : A rack-level solution that integrates 72 Blackwell Ultra GPUs and 36 Grace CPUs. It can be regarded as a single giant AI GPU, improving complex task decomposition and AI reasoning capabilities.
- HGX B300 NVL16 : A high-performance server unit that increases inference speed for large language models by 11 times, increases computing power by 7 times, and increases memory capacity by 4 times compared to the previous generation Hopper GPU.
The current implementation plan is:
- Cloud service providers : AWS, Google Cloud, Microsoft Azure, Oracle Cloud, etc. will be the first to provide Blackwell Ultra instances.
- Server manufacturers : Dell, HP, Lenovo, Supermicro, etc. plan to launch AI infrastructure based on Blackwell Ultra by the end of 2025.
Blackwell Ultra is designed specifically for AI reasoning and supports the entire process of pre-training, post-training and reasoning. Huang Renxun called it “the biggest leap in the field of AI reasoning.”
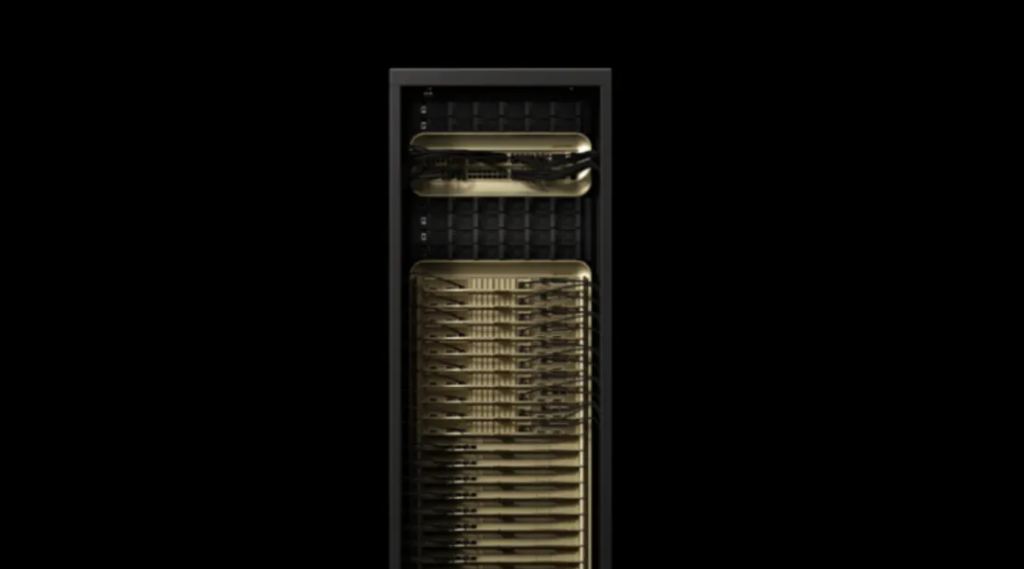
All the way to 2028: Rubin, Rubin Ultra, Feynman
NVIDIA will continue its “one flagship per year” strategy and the tradition of “naming after outstanding scientists”. In 2026, it will launch the ” Rubin ” architecture named after Vera Rubin, a female scientific pioneer who “confirmed the existence of dark matter” , and will update the Ultra version in 2027. In 2028, it will launch the “Feynman” architecture named after Richard Feynman, a well-known scientist, member of the National Academy of Sciences of the United States, and Nobel Prize winner in Physics.
The Vera Rubin NVL144 will be launched in the second half of 2026;
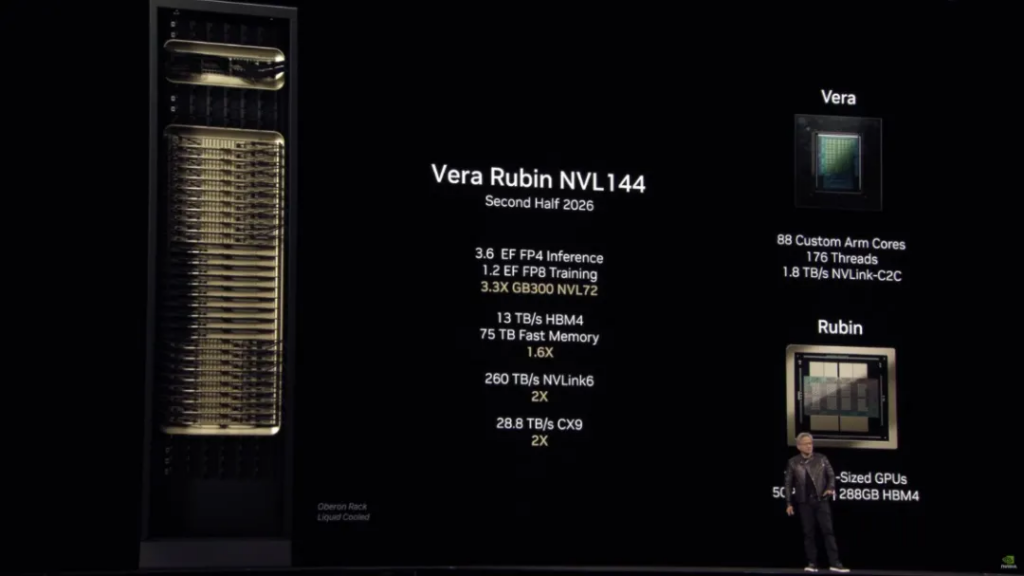
Rubin Ultra NVL576 will be launched in the second half of 2027 ;
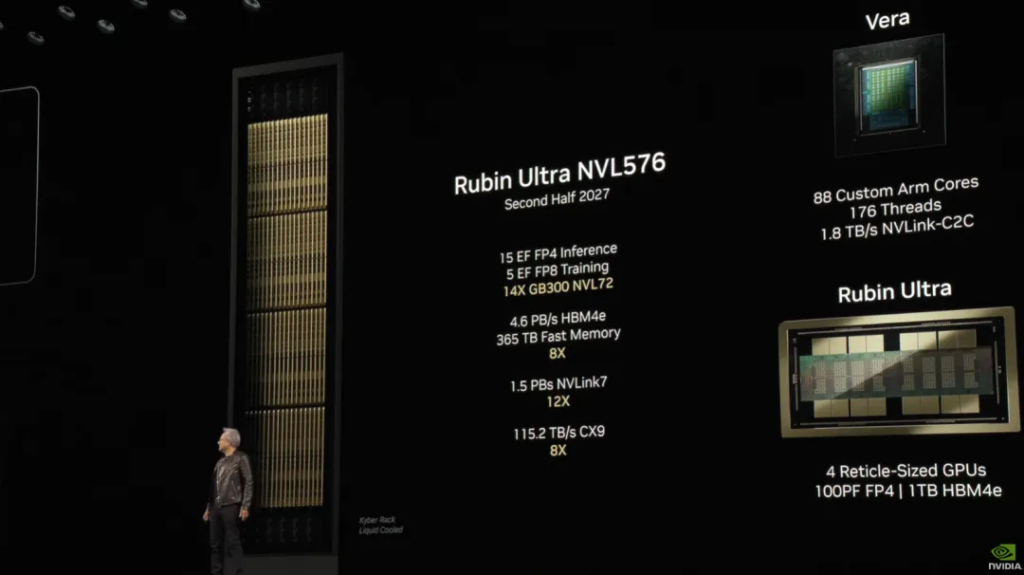
Huang said that Rubin’s performance can be 900 times that of Hopper, while Blackwell is 68 times that of Hopper.
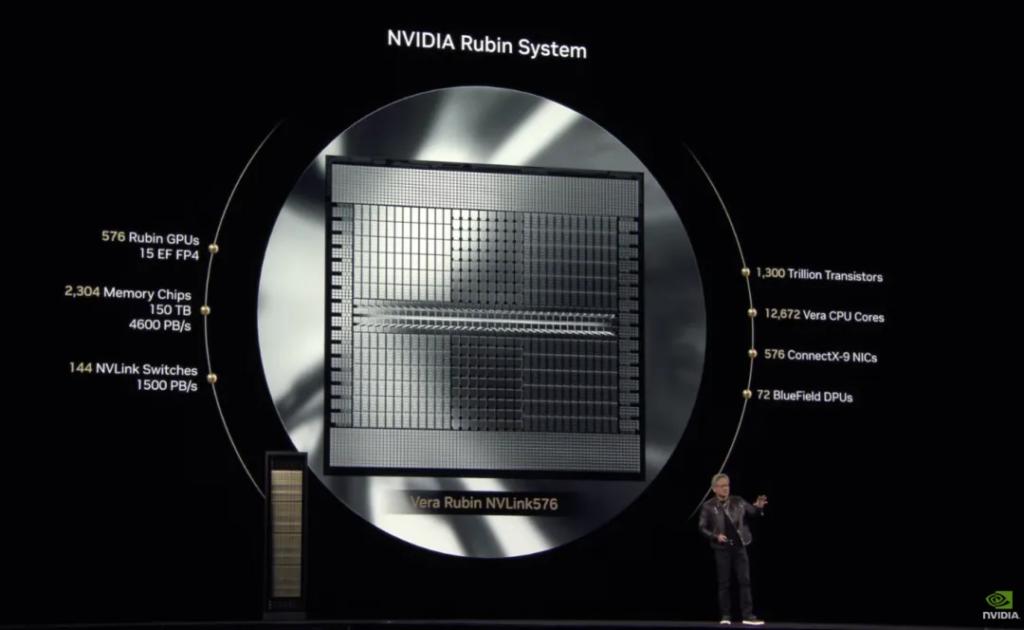
As for Feynman, it is still in the stage of creating a new folder.
Personal AI Supercomputer
NVIDIA launches DGX Spark , which will be equipped with GB10 Superchip (a simplified version of Blackwell), with a computing power of 100 trillion operations per second, suitable for model fine-tuning and reasoning. The starting price is about $3,000.
This is actually the ” mini supercomputer ” Project DIGITS that was unveiled at CES 2025 this year. Its design concept is a unit that can run high-end AI models and takes up the same space as a standard desktop computer.
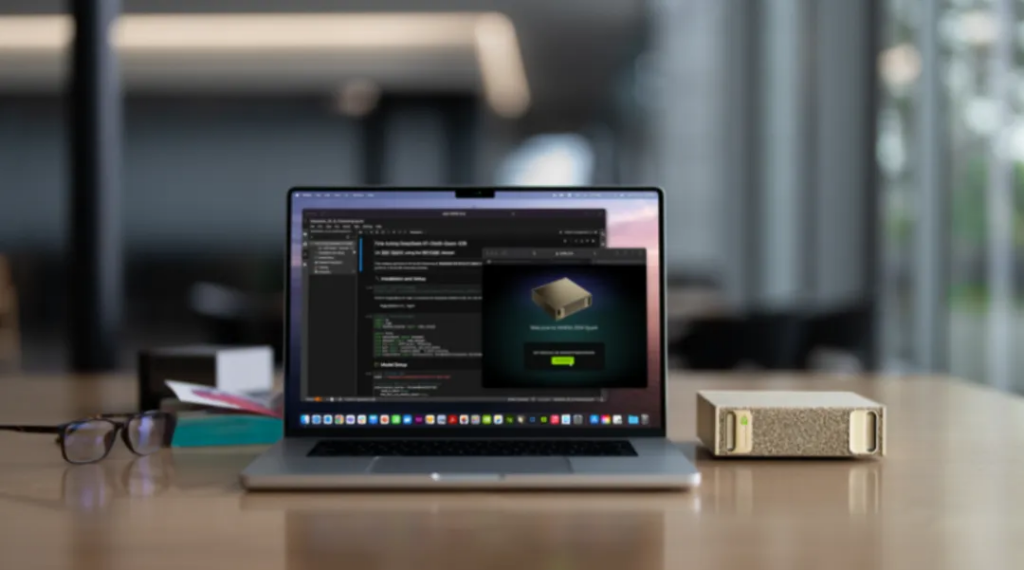
Another product, DGX Station , is an enhanced version of the mini computer above, which Huang Renxun calls a “desktop-class data center.” It is equipped with a B300 Grace Blackwell Ultra chip, 784GB of unified memory, and supports large-scale training and reasoning. It is expected to be launched by manufacturers such as Asus and Dell this year.
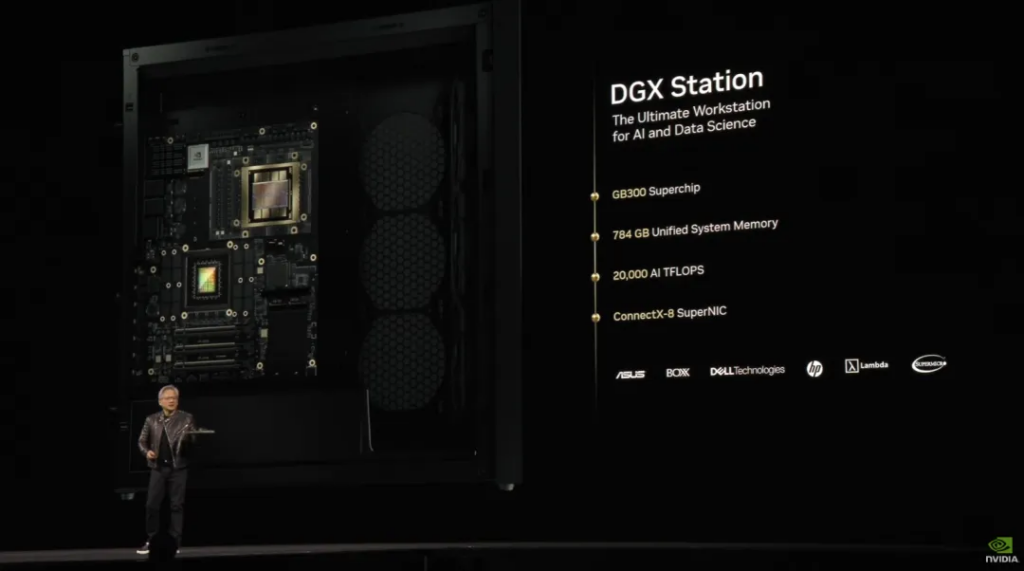
Preparing for quantum computing
Nvidia has attached great importance to quantum computing this year. This year, it established the National Accelerator Quantum Research Center (NVAQC) in Boston. Based on GB200 NVL72 hardware, the goal is to solve problems such as quantum bit noise and experimental chip design, and promote the integration of quantum computing and AI. At that time, the MIT (Massachusetts Institute of Technology) quantum engineering team will use the center to develop quantum error correction technology, which is expected to be launched within the year .
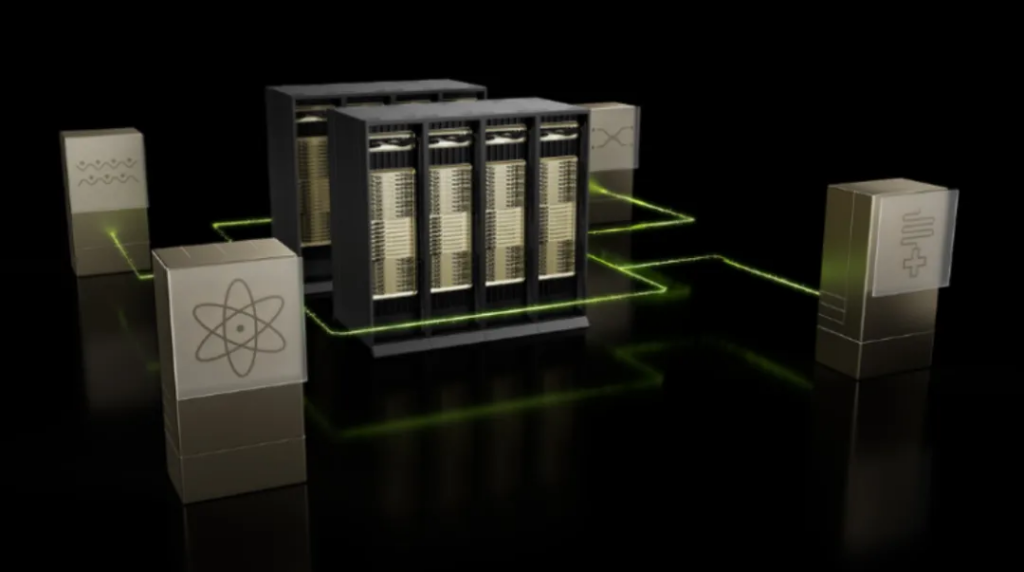
In addition, this GTC 2025 will end with a “Quantum Computing Forum”, where Huang Renxun will talk with 14 business leaders about topics related to quantum computing. This should be compared to last year’s ” Huang Renxun Dialogues with Transformer Seven ” forum, which shows how much Huang Renxun attaches importance to it.
At that time, the CSDN AI Technology Base Camp account will also follow up on this forum.
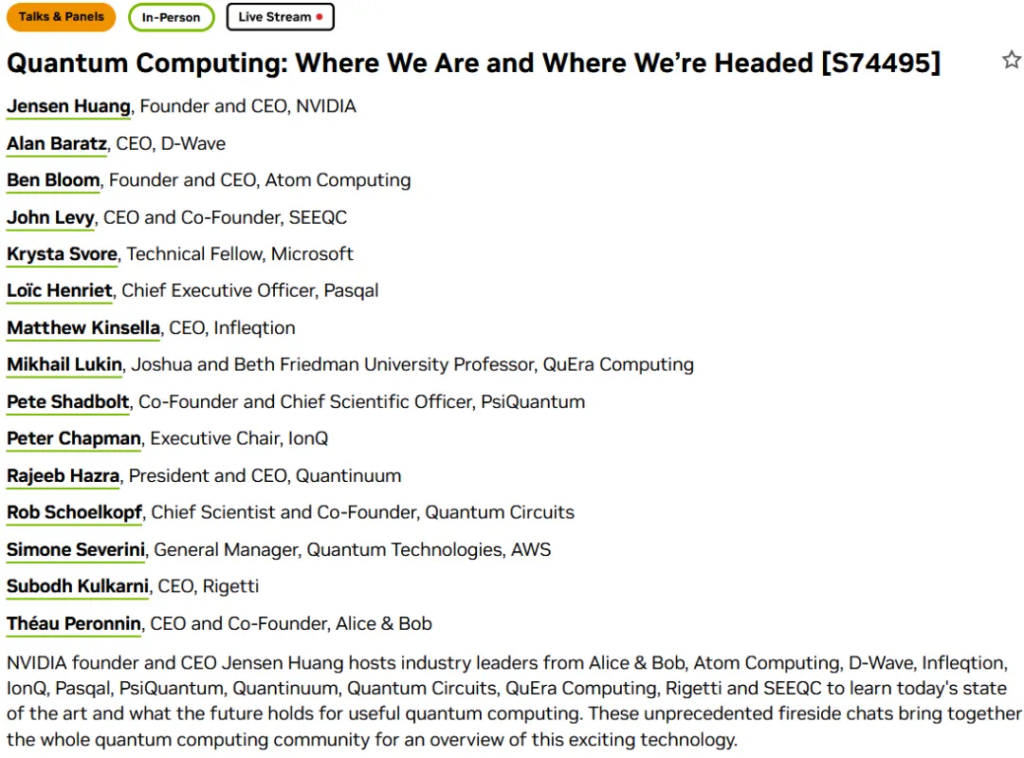
In addition, Huang Renxun also announced at the main conference that NVIDIA launched Spectrum-X and Quantum-X switches based on silicon photonics technology:
- Spectrum-X supports 128 ports of 800Gb/s or 512 ports of 200Gb/s, with energy efficiency improved by 3.5 times and signal stability improved by 63 times, making it suitable for ultra-large-scale GPU interconnection.
- Quantum-X is a liquid-cooled design that supports 144-port 800Gb/s InfiniBand, doubling the AI computing network speed and increasing scalability by 5 times.
Huang Renxun said it will “break the limitations of traditional networks and power million-GPU-level AI factories.”
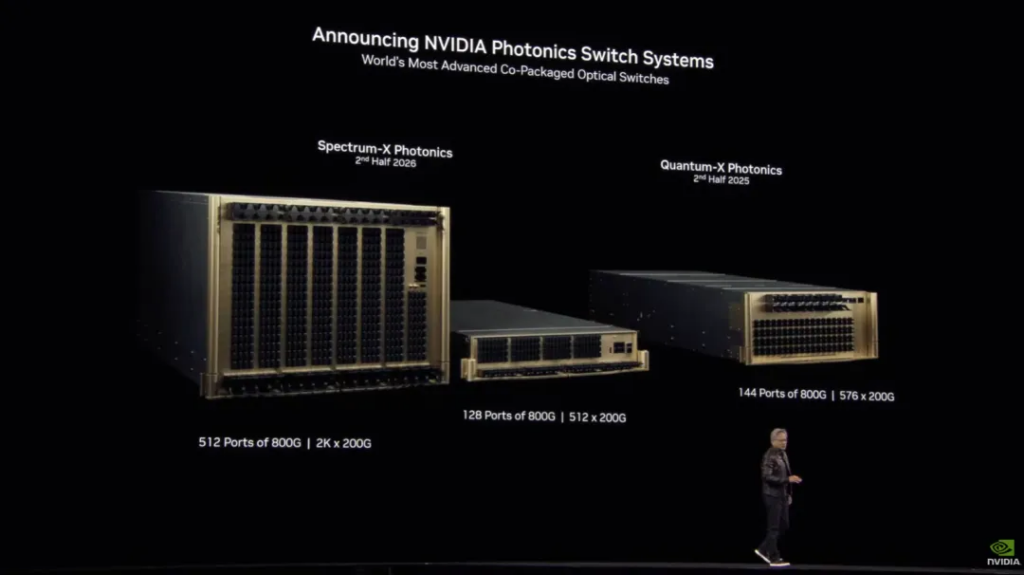
“The era of general-purpose robots has arrived”
At the end of his speech, Huang Renxun announced a bunch of technologies that can accelerate
The first is the long-standing NVIDIA Isaac GR00T N1 , the world’s first open and fully customizable base model for general humanoid reasoning and skills. It is also equipped with NVIDIA Isaac GR00T blueprint technology for generating synthetic data.
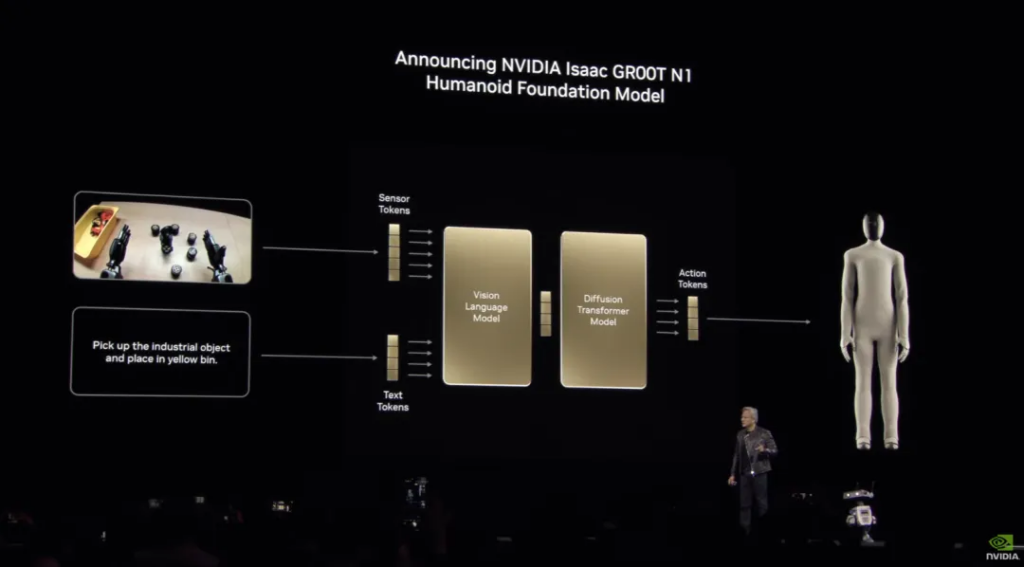
This time, the official name added half of “Isaac Newton’s name” to pay tribute to this well-known scientist.
The other half of the name is used for an open source physics engine Newton – it was jointly developed by Google DeepMind and Disney Research and is designed specifically for developing robots.
Huang Renxun finally emphasized: ” The era of general-purpose robots has arrived. With the help of NVIDIA Isaac GR00T N1 and new data generation and robot learning frameworks, robot developers around the world will open up the next frontier of the artificial intelligence era. “
Then came the final surprise: Nvidia’s little robot Blue made its debut.
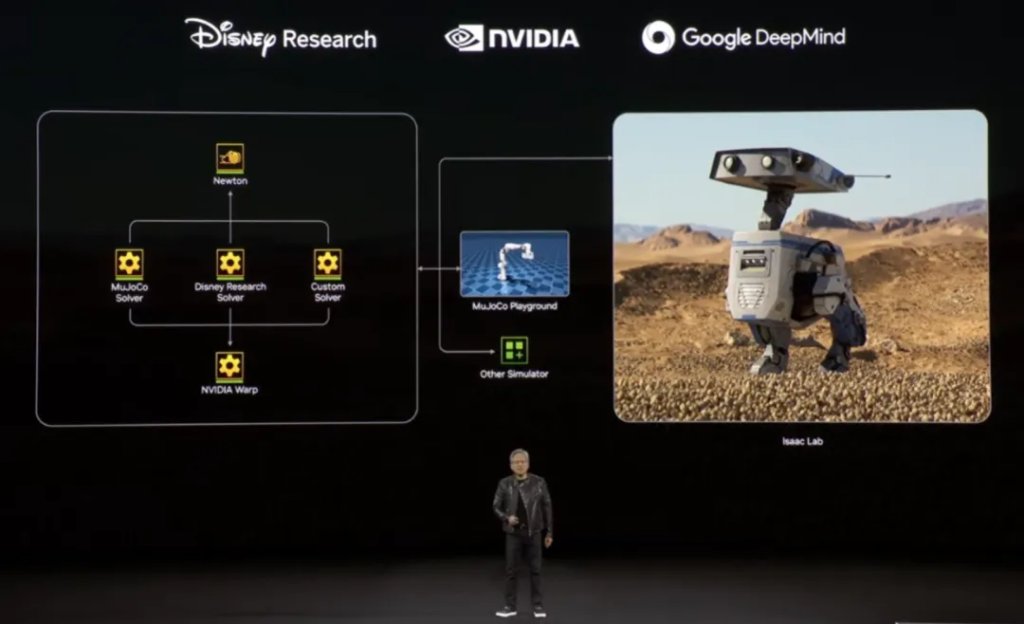
From the animation produced by Disney, to the AI technology provided by DeepMind, and the hardware capabilities of NVIDIA, this robot driven by the Newton physics engine has become a reality.
American netizens think it looks like a robot from Star Wars, and I personally think it looks more like the classic movie WALL·E. Blue is not a consumer product, so Huang Renxun really just wanted it to make an appearance to close the show.
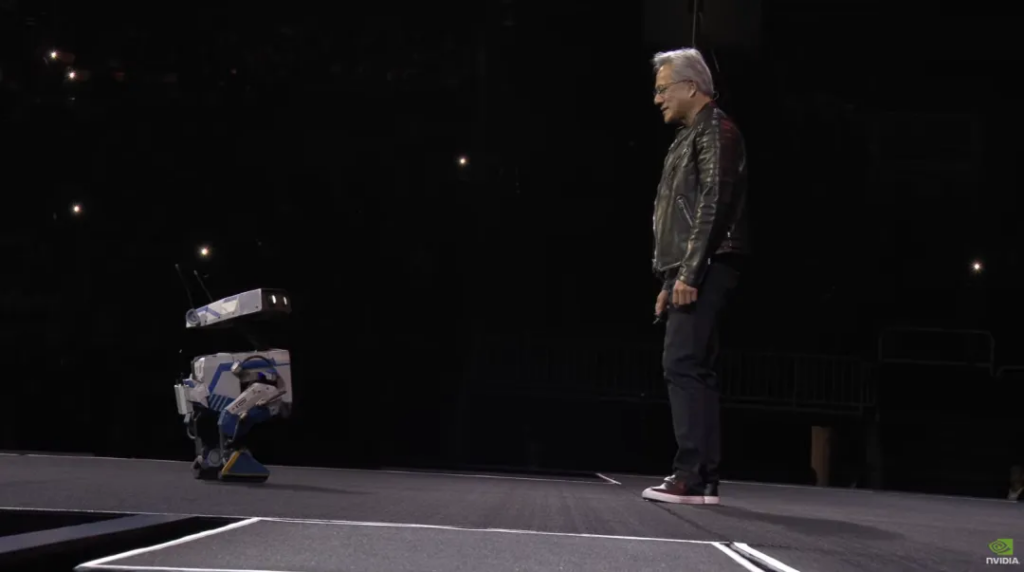
Next, let’s review the full content of this main speech in detail:
This year’s GTC conference was held at the SAP Center, a sports stadium in San Jose, California, USA . It was said that the original plan was to hold the main conference at another conference center in San Jose , but due to the large number of participants ( about 19,000 people ), the conference center could not accommodate all the audience, so NVIDIA could only hold it at the SAP Center sports stadium – and the rest of the GTC conference was still held in the conference center.
One hour before the main meeting, NVIDIA held a warm-up live broadcast , inviting many entrepreneurs to take turns to talk about their views on industry trends at a table, including Huang Renxun, who was about to take the stage to give a speech.
Even though he was wearing an apron, Huang wore a leather jacket to appear on camera, sticking to his character. He also brought out a pot of pancakes and talked about stories, comparing himself when he was young with himself now.
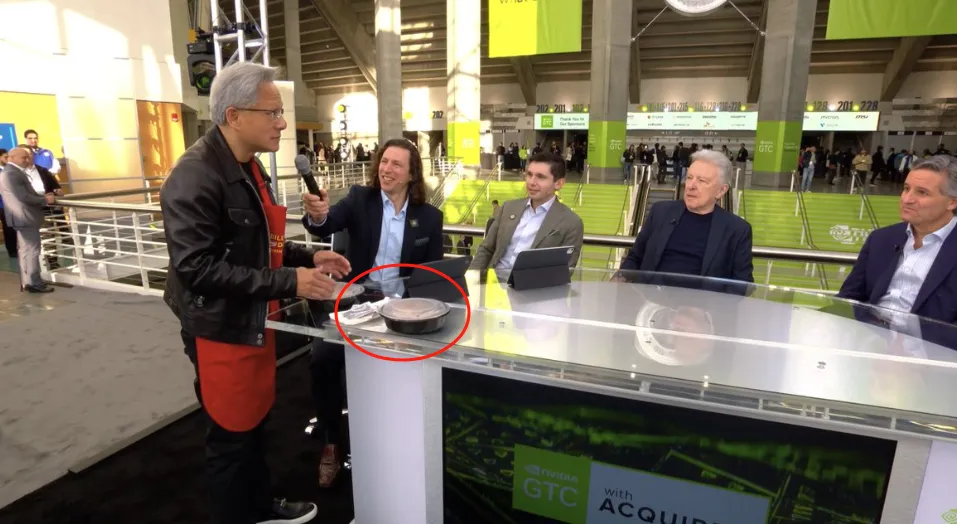
Huang and pancakes have a long history. For example, when he immigrated to the United States at the age of nine, he worked as a dishwasher in a Denny’s restaurant, serving customers signature pancakes and coffee all day long. Later, Huang Renxun himself also liked pancakes . He mentioned his love of eating pancakes when he commented on AI safety issues in 2023 and interviewed Zuckerberg in 2024. In another conversation with the CEO of HP, he ate pancakes directly on the show and demonstrated his way of eating pancakes .
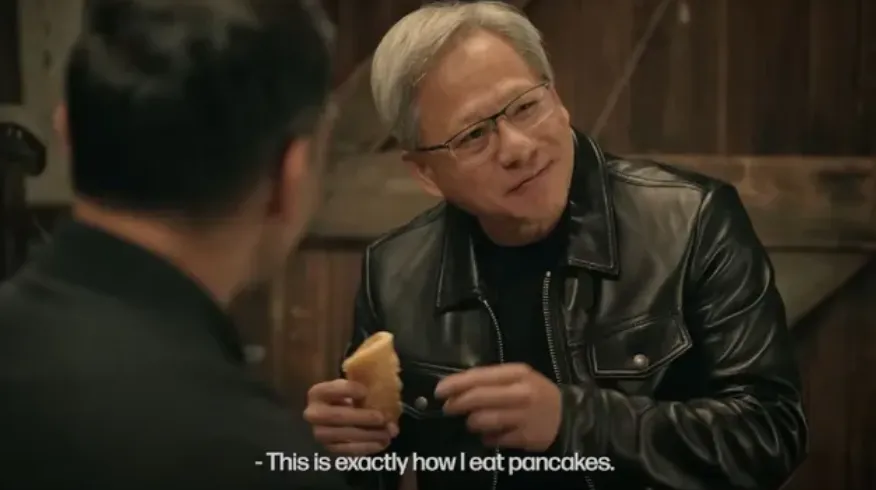
The time came to 1:10 in the morning and the late main meeting officially began.
01
Two key words: “token” and “AI factory”
“Last year the whole world got the Scaling Law wrong. Everyone thought the law was broken, but it was actually because the amount of computation required for inference was 100 times more than people thought last year.”
“The computing landscape has reached an inflection point, AI growth is accelerating, and data center capital spending is expected to exceed $1 trillion by 2028.”
“What is an AI factory: Computers have become tools for generating tokens, not tools for searching documents.”
First up is the official opening trailer for GTC 2025 this year: ” In NVIDIA’s world, tokens are the basic unit of AI computing. Tokens can not only teach robots how to move, but also teach them how to bring happiness… “
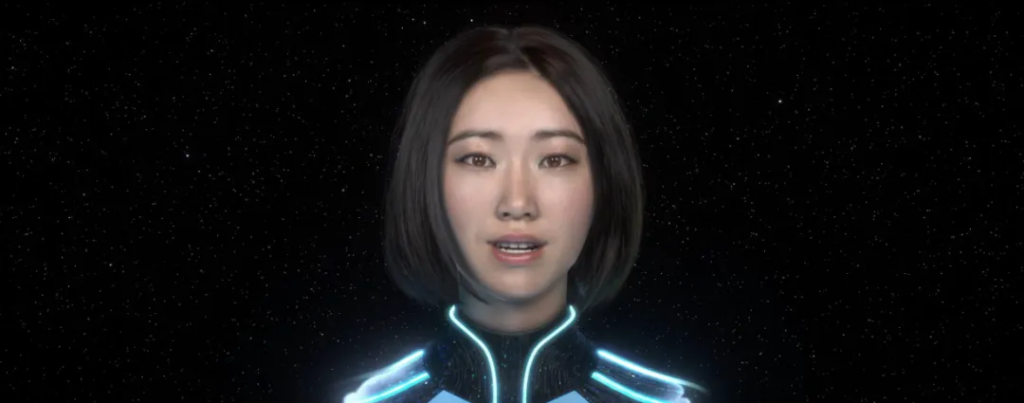
The idea that this promotional video wants to express is that one token gives birth to two tokens, two tokens give birth to three tokens, and then three give birth to everything . “Tokens connect all the dots, making life within reach, and taking us to take the next great leap forward, to where no one has been before.”
After the short film ended, Huang Renxun came on stage and exclaimed, “(2025 is) what an amazing year .”
He wanted to invite everyone to Nvidia headquarters through the magic of artificial intelligence, and then emphasized that his speech ” did not have any script or teleprompter “:
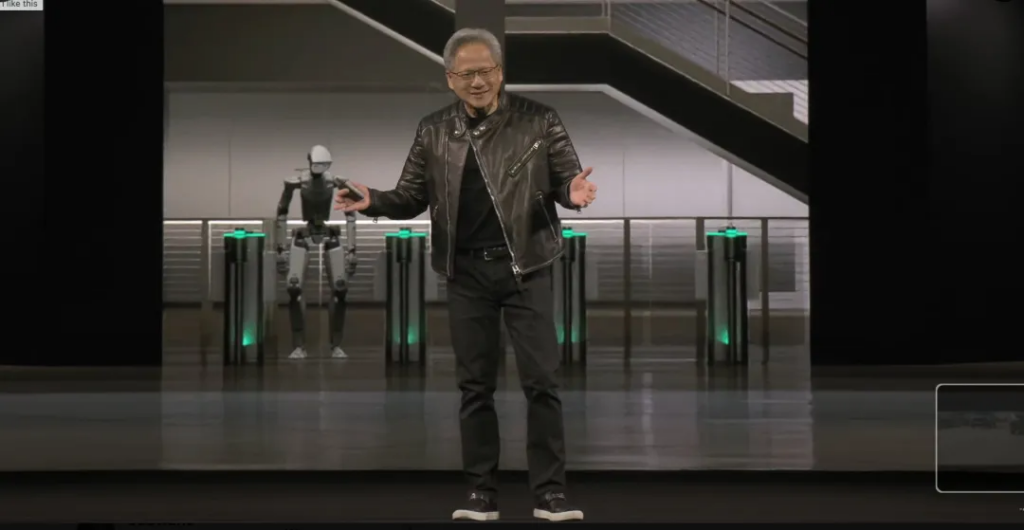
The easter egg here is that in the background animation, there is a humanoid robot trying to swipe his ID card to enter Nvidia headquarters, which hints at the final explosive point of this speech.
Before the speech, Huang thanked the sponsors as usual: from healthcare, transportation to retail… almost every industry was represented. Especially in the computer industry, almost every IT giant was on the list of sponsors.
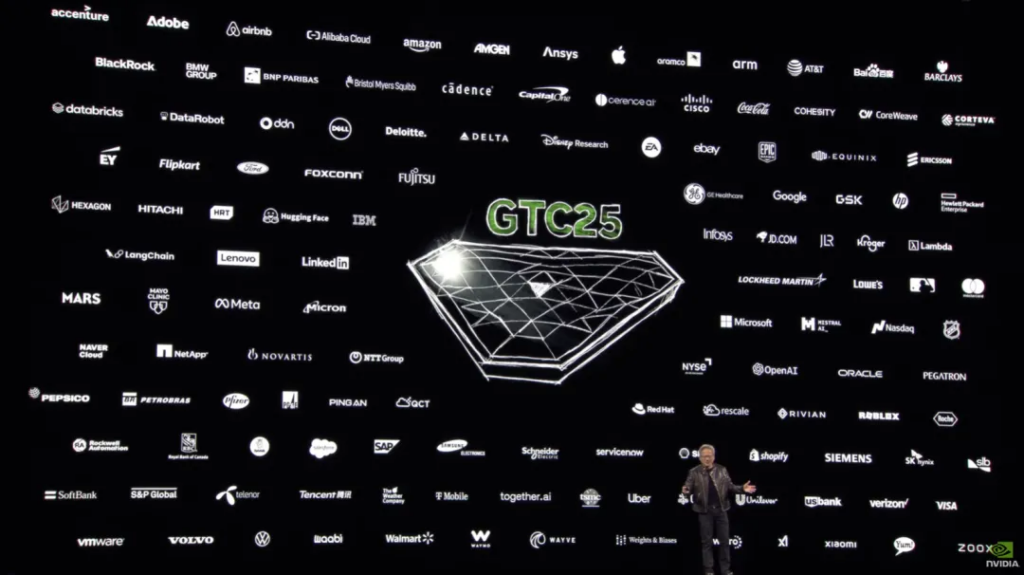
This was followed by another short film, emphasizing that “GTC originated from GeForce” to commemorate this graphics card series that was originally created for playing games:
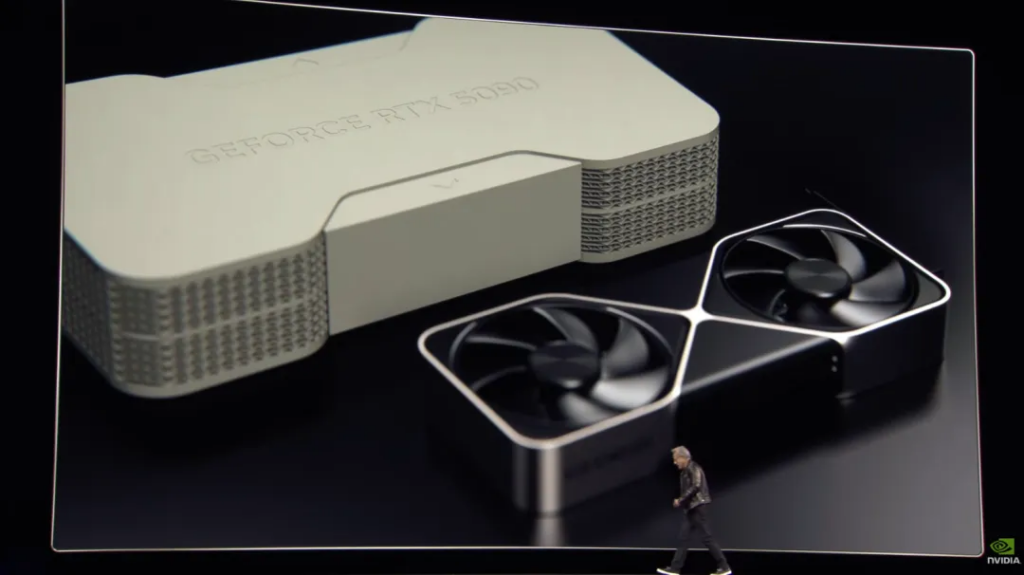
Huang Renxun then recalled the time when the G series graphics cards dominated the market, and then held up an RTX 5090 graphics card and an RTX 4090 graphics card in each hand: “You will find that its size is 30% smaller.”
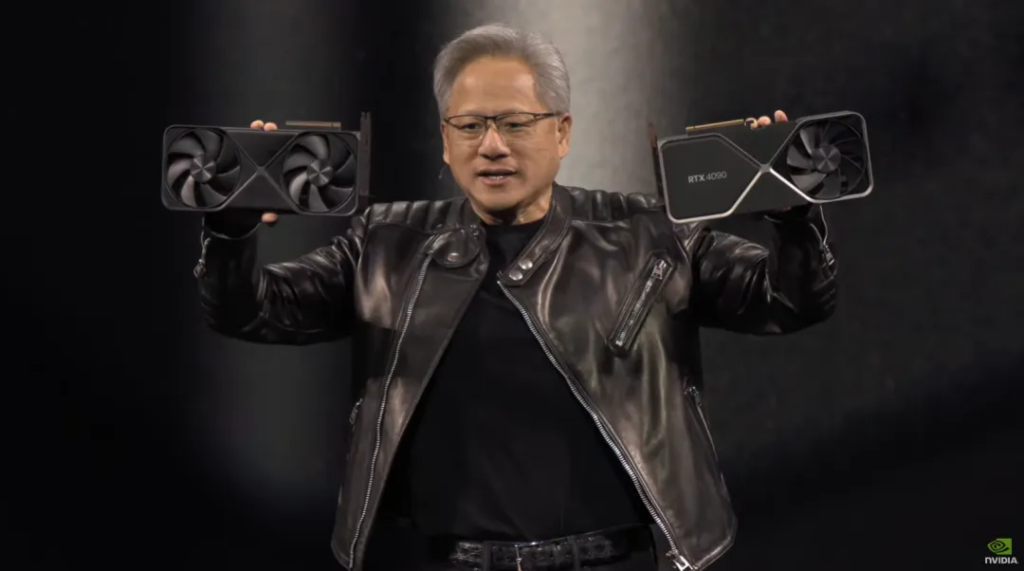
There is a wonderful sentence here: The greatest historical significance of NVIDIA’s GeForce series graphics cards is the introduction of CUDA technology to the world, and then CUDA promoted the development of artificial intelligence, and now artificial intelligence in turn has completely revolutionized computer graphics, and generative AI has fundamentally changed the way of computing .
The background below is a real-time rendering based entirely on path tracing:
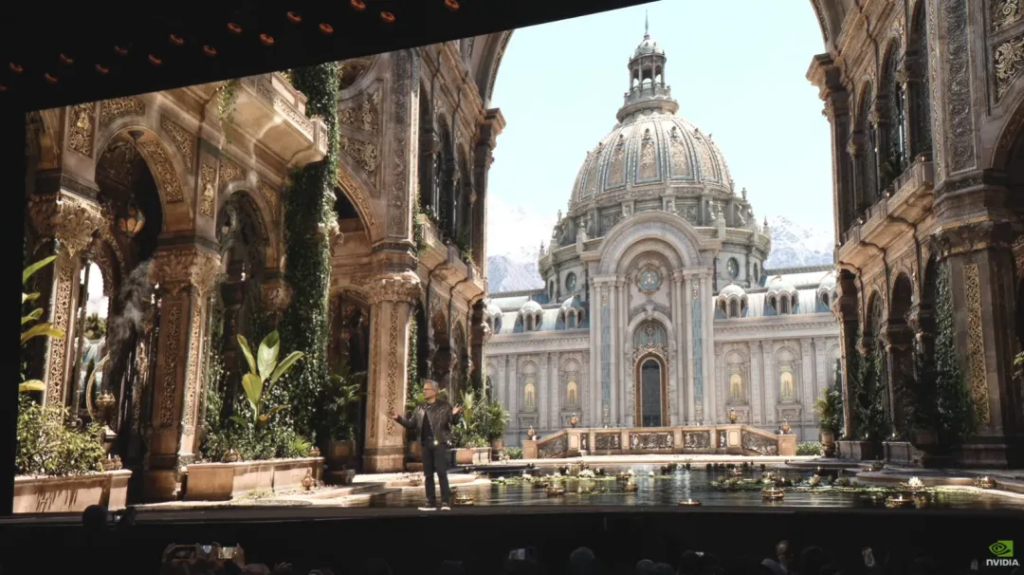
When talking about AI, we introduced the first growth chart of today.
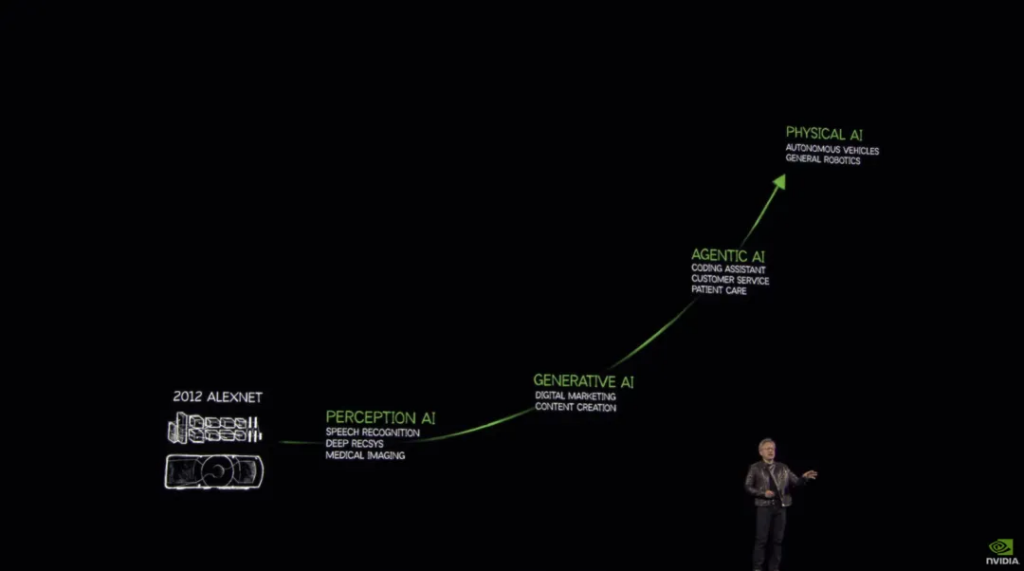
Since Alex Krizhevsky, Ilya Sutskever, and Geoffrey Hinton developed the AlexNet deep learning neural network in 2012, the initial perception AI (Perception AI) has been developed; then after the emergence of ChatGPT, the familiar generative AI (Generative AI) was born; in recent years, with the maturity of the idea of agents and the birth of reasoning models, autonomous AI (Agentic AI) has emerged; finally, with the rise of embodied intelligence and autonomous driving, there is a need for models that use motor skills to understand the real world and interact with it, namely physical AI (Physical AI).
Huang Renxun said that the ability to understand the physical world will usher in a new era of AI, making robots possible. Like every previous stage, it brings new market opportunities – and then brings more partners to the GTC conference.
Here he likened last year’s GTC 2024 conference to “the first live performance of AI”, which is the Woodstock Music Festival of AI (the Chinese metaphor is the Midi Music Festival). This year’s conference was described by him as the Super Bowl of AI (the Chinese metaphor is the Spring Festival Gala).
So, what factors make each stage of AI possible? Look at the following picture:
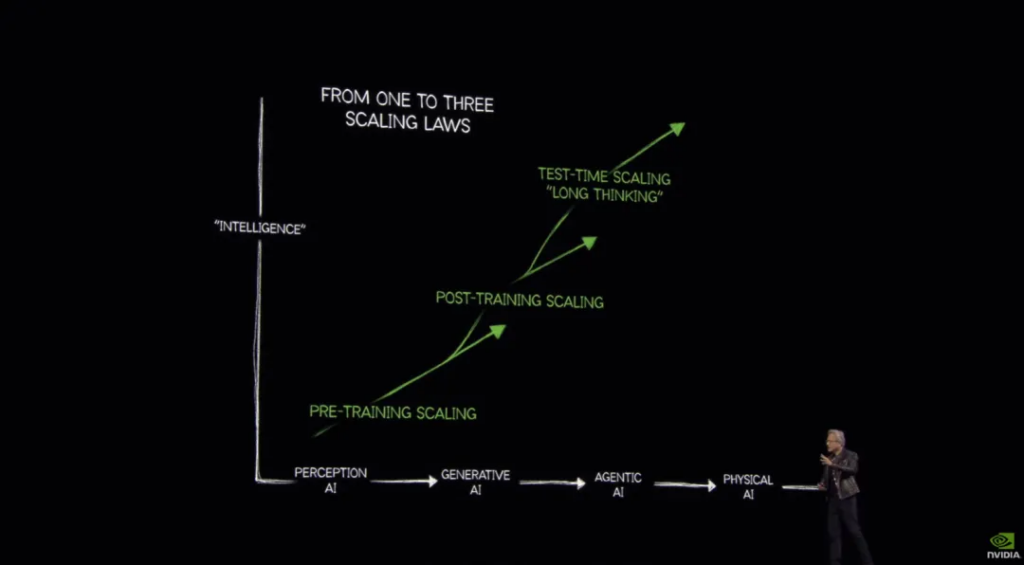
Huang Renxun mentioned “three basic issues”:
- 1. Data.
Artificial intelligence is a data-driven computer science approach that requires data to learn knowledge.
- 2. Training.
To train the AI, our ultimate goal is “hopefully no humans in the loop of training” because the reason why humans in the loop are fundamentally challenging is the lifespan. We want AI to be able to learn at superhuman speeds, faster than real time, and at a scale that no one can match.
- 3. Expansion.
This issue involves how we can find various algorithms so that artificial intelligence can become smarter as more data increases, rather than stopping when the data runs out.
The figure shows three types of extensions: the most important thing from perceptual AI to generative AI is the pre-training extension , such as GPT-3.5; and through post-training extension , we have obtained stronger generative AI, such as GPT-4; and then there is the test-time training extension . It is this step that gives us long-thinking models such as OpenAI o1 and DeepSeek-R1, allowing AI to learn reasoning.
Therefore, the number of expansion rules has actually increased from one to three.
Huang Renxun’s next words are very classic: ” Last year, almost the whole world got the scaling law wrong . Everyone thought that the scaling law was invalid, but in fact it was because the amount of computing required for autonomous AI reasoning was 100 times more than people thought last year. “
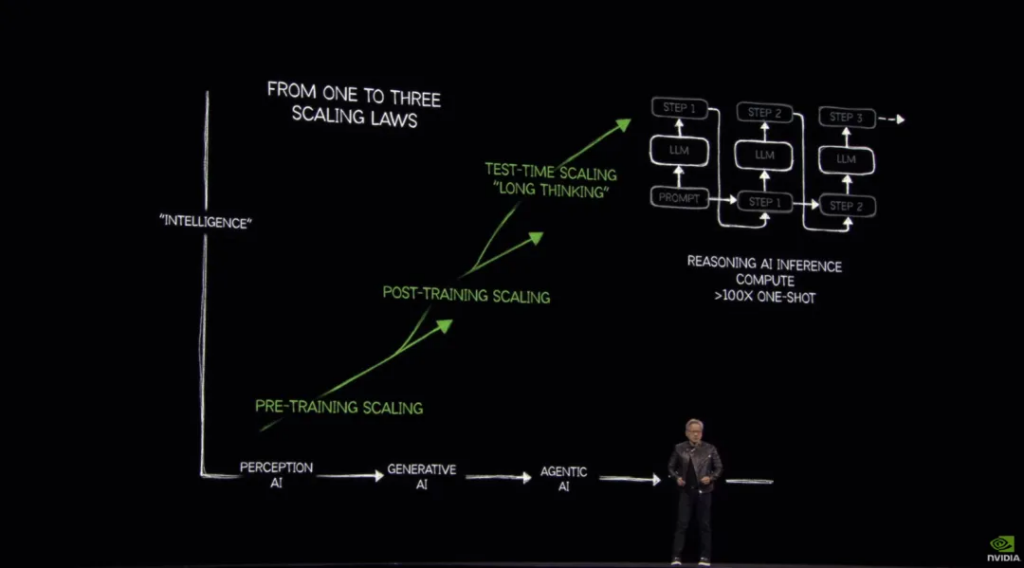
We now have AI that can reason step by step through thought chaining and other techniques, but the basic process of generating tokens has not changed. This reasoning requires more tokens, and the amount of computation required per second is still high in order to keep the model responsive.
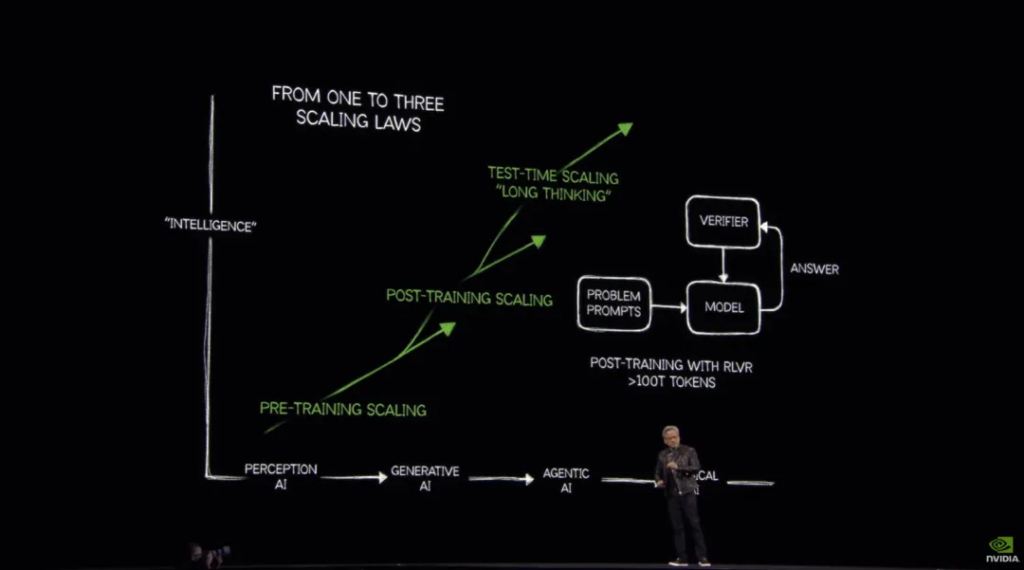
So Huang gave a solution: synthetic data . “Reinforcement learning is a major breakthrough in the past few years. Give AI thousands of different examples, let AI solve the problem step by step, and reward it (reinforce) when it does better. This means that trillions or even more tokens are needed to train the model. In other words: generate synthetic data to train AI.”
To prove his point, Huang directly brought out the data, saying that ” computing is facing huge challenges, and the industry is responding to this .” Last year, the shipments of Hopper chips ( the H100 series we often talk about, DeepSeek uses H800 ) ranked first among cloud service providers, which is a peak of the Hopper series. However, compared with the first year of the new series Blackwell, there is a three-fold gap:
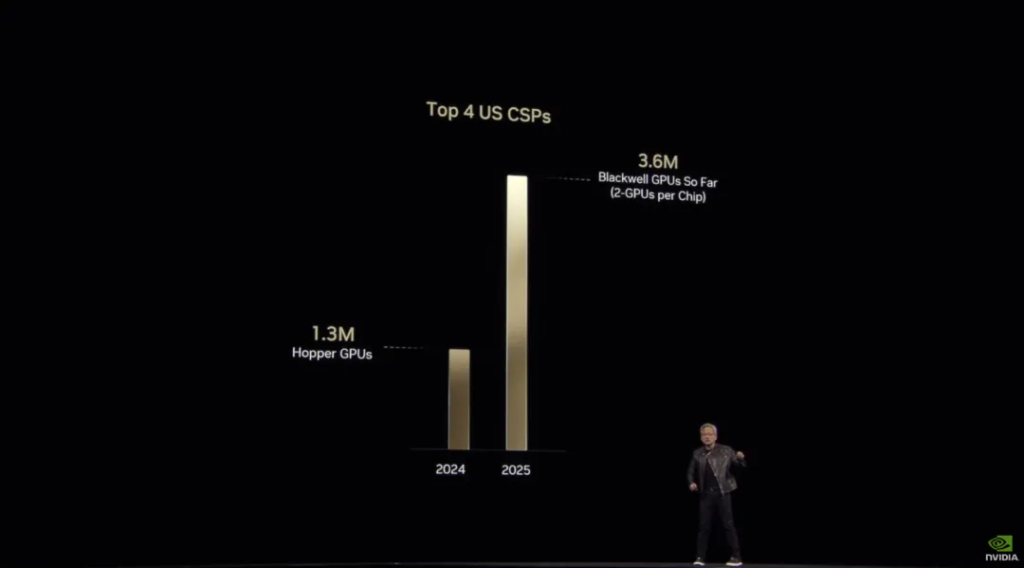
You have to know that Blackwell has only just started shipping products.
“We’ve known for a long time that general-purpose computing is obsolete, but of course, let it be, we need a new approach to computing, and the world is going through a platform shift,” Huang said.
Then came the first breaking news of the night: “Data center construction is turning towards accelerated computing (that is, using GPUs and other accelerators instead of just CPUs), and the computing field is turning. By 2028, data center capital expenditures are expected to exceed $1 trillion. “
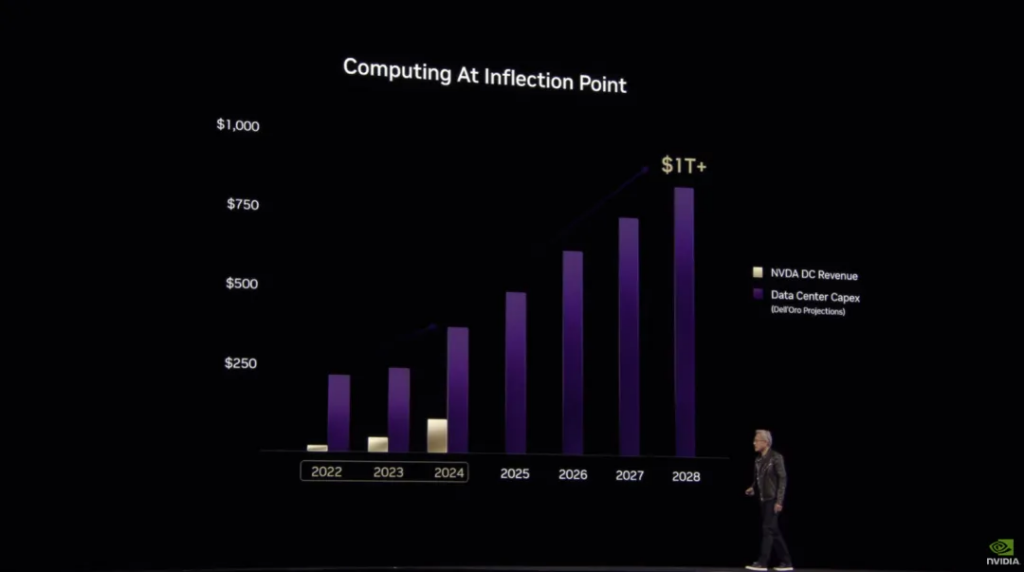
Finally, here is the summary of this paragraph, explaining what exactly an AI factory is: ” Computers have become tools for generating tokens, not files. The shift from retrieval-based computing to generation-based computing, from the old way of operating data centers to a new way of building these infrastructures. I call them AI Factories. “
02
From CUDA to edge computing and autonomous driving
“I love the work we do, and even more so what you (developers) build with it.”
“The era of autonomous driving has arrived!”
After introducing the concept of the AI factory, Huang Renxun changed the subject: ” Although everything in the data center will be accelerated, it is not all driven by artificial intelligence – frameworks from physics, biology and other scientific fields are also needed. “
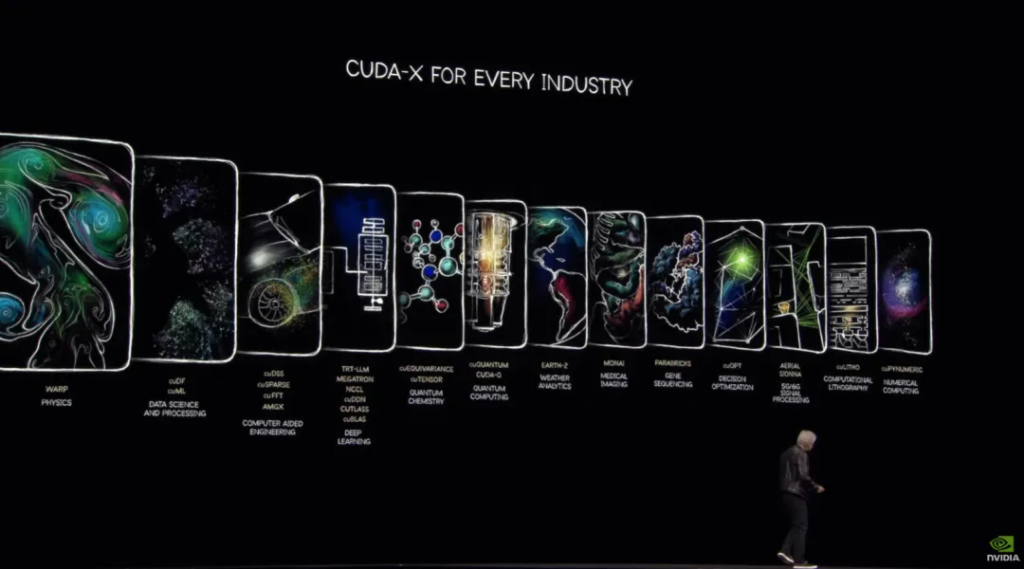
These frameworks have been provided by NVIDIA as part of its CUDA-X library . cuLitho is used for computational lithography, cuPynumeric is used for numerical computing, Aerial is used for signal processing, etc. This is also NVIDIA’s “moat” in the larger industry.Huang Renxun also mentioned that the 20th (our 21st) Eastern Time will be Nvidia’s first “Quantum Day”, which is actually the quantum computing forum mentioned earlier in this article. Huang Renxun will talk with many CEOs of quantum companies.Enter a short video again: Since the birth of CUDA, 6 million developers in more than 200 countries have used it and changed the way of computing… Developers use CUDA to accelerate scientific discovery, reshape industries, and empower machine vision, learning and reasoning. Today, NVIDIA Blackwell is 50,000 times faster than the first generation Cuda GPU.
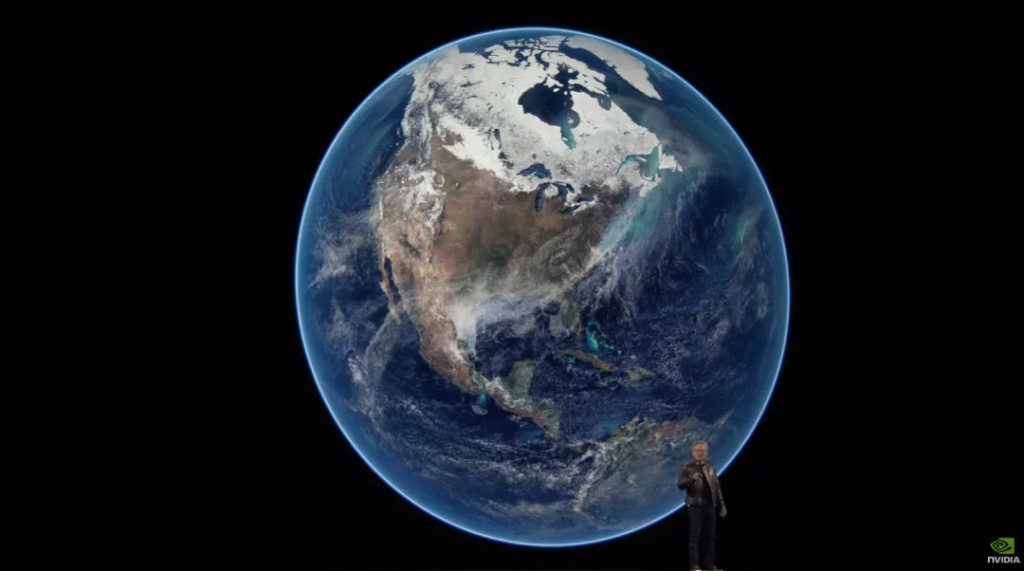
At the end of the video, Huang Renxun thanked all the developers: “I love the work we do, and I love everything you develop with it even more.”
Then we come to everyone’s favorite topic of AI.
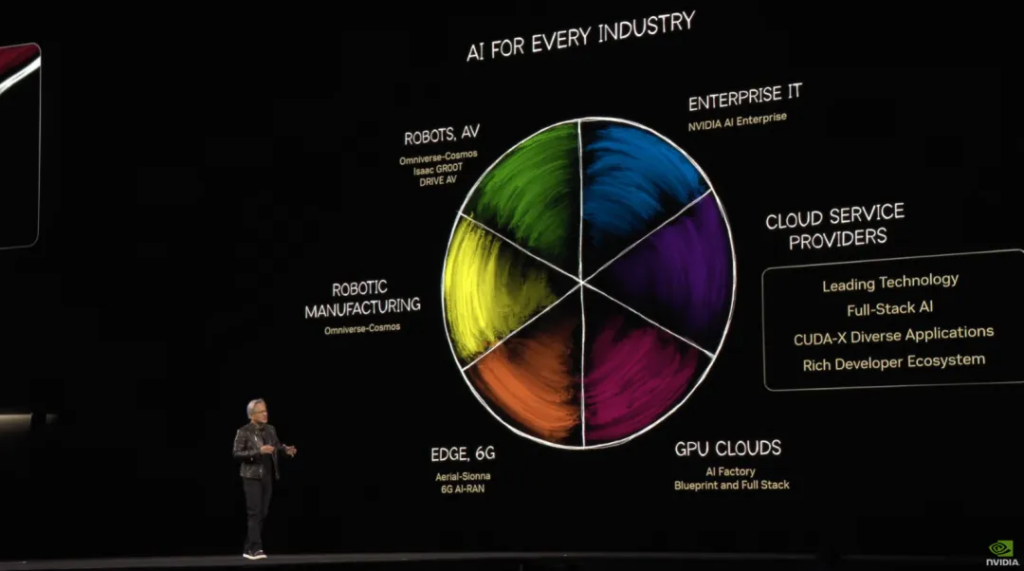
“ It’s no secret that AI originated in the cloud, and there’s a good reason why it originated in the cloud, because it turns out that AI requires infrastructure, and so-called ‘machine learning’, as the name implies, requires a machine to do the science. ”
“ And cloud data centers have the infrastructure, they have extraordinary computer science, extraordinary research, the perfect environment for AI to take off in the cloud, and the NVIDIA partner network of cloud service providers (CSPs), but that’s not where AI is limited to. AI is going to be everywhere, and we’re going to talk about AI in many different ways. ”
“ Of course, cloud service providers like our leading technology and they like that we have a complete stack. But now they want to bring AI to the whole world, and things are a little different. GPU cloud, edge computing, etc., all have their own requirements. ”
I have laid so much groundwork, all talking about the cloud, just to introduce this paragraph: ” We announced today that Cisco, T-Mobile, and NVIDIA will build a complete wireless network stack in the United States, targeting edge computing for artificial intelligence. “
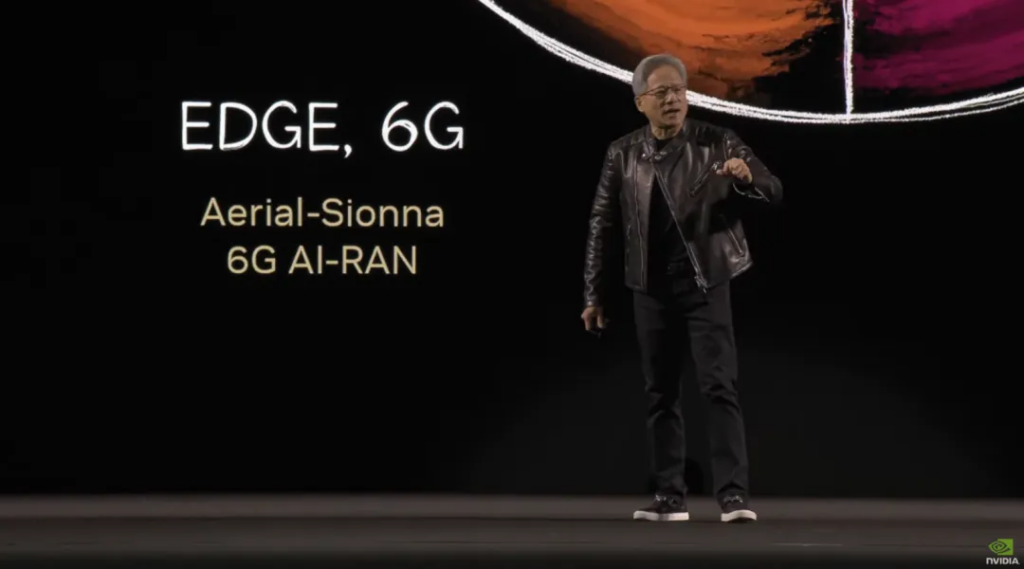
But this is just one industry, and AI will empower thousands of industries. ” And self-driving cars. It was AlexNet that got NVIDIA all-in on self-driving car technology. And now, their technology is being used all over the world. NVIDIA builds computers for training, simulation, and self-driving cars themselves, and today we are announcing that NVIDIA will work with General Motors (GM) to build its future self-driving fleet. “
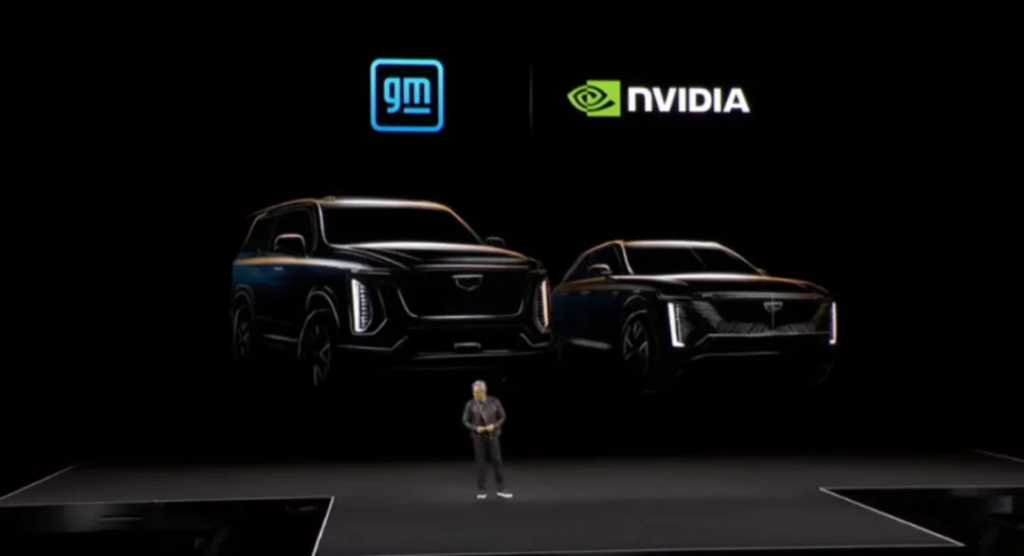
“The era of autonomous driving has arrived!” I don’t know if Huang has said this before. Perhaps the last person to say this was Musk.
When people discuss autonomous driving, the most important issue is safety, which is also the key to NVIDIA’s work in the automotive field this year. Now NVIDIA has had all 7 million lines of code evaluated for safety by a third party, and announced NVIDIA Halos, a chip-to-deploy autonomous driving safety system :
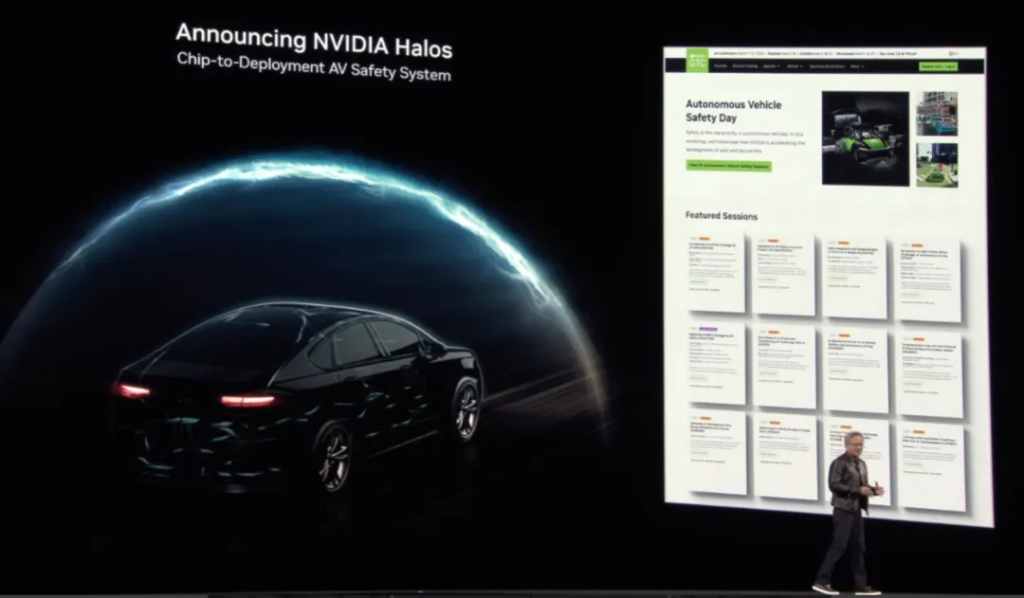
Next, we come to the short film session again, this time about NVIDIA’s technology and methods for creating self-driving vehicles. Digital twins, reinforcement learning, generating diverse scenarios, etc. will all be built on NVIDIA Cosmos to form a training loop: using AI to create more AI .
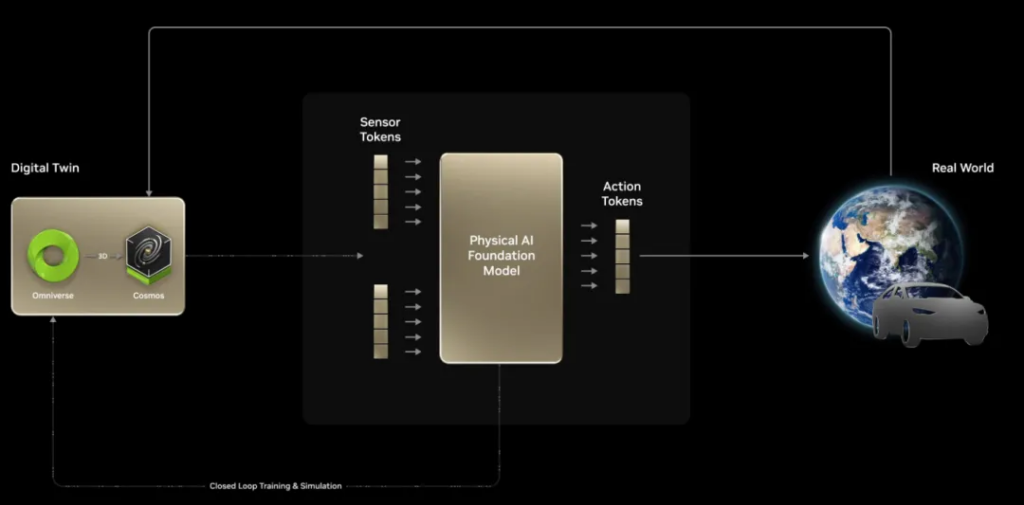
03
Data Centers and the Next N Generation of Chips
“You should gasp when you see this.”
“In the future, when NVIDIA discusses NVLink interconnect domains, it will no longer be based on the number of GPU chips, but on the number of GPU core dies.”
“Every future data center is going to be power constrained. We are a power constrained industry right now.”
After the short film ended, the topic turned directly to the data center.Huang Renxun made a major announcement: The Blackwell series has been fully put into production .
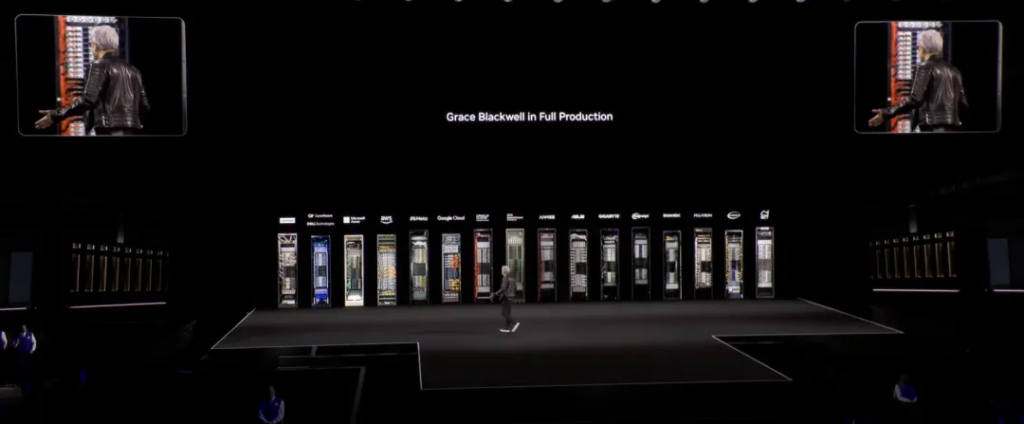
Then the real stuff came on stage. Huang first showed off the various rack systems provided by its partners. Nvidia has long been researching distributed computing – how to scale up and scale out.
Since horizontal scaling is difficult, NVIDIA first achieved vertical scaling through HGX and 8 GPU configurations.
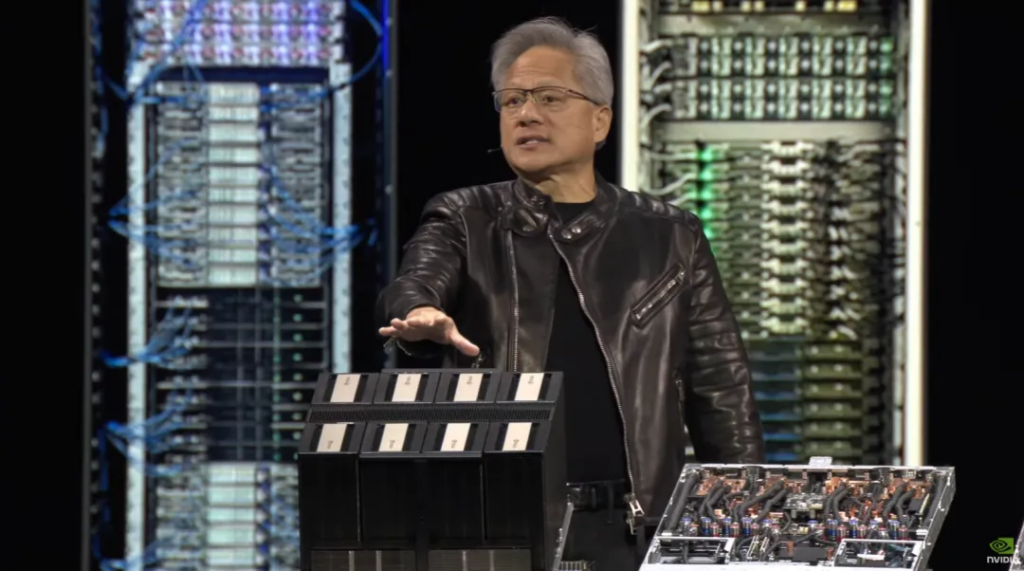
He then held up an H-series chip from the HGX and said it was a thing of the past:
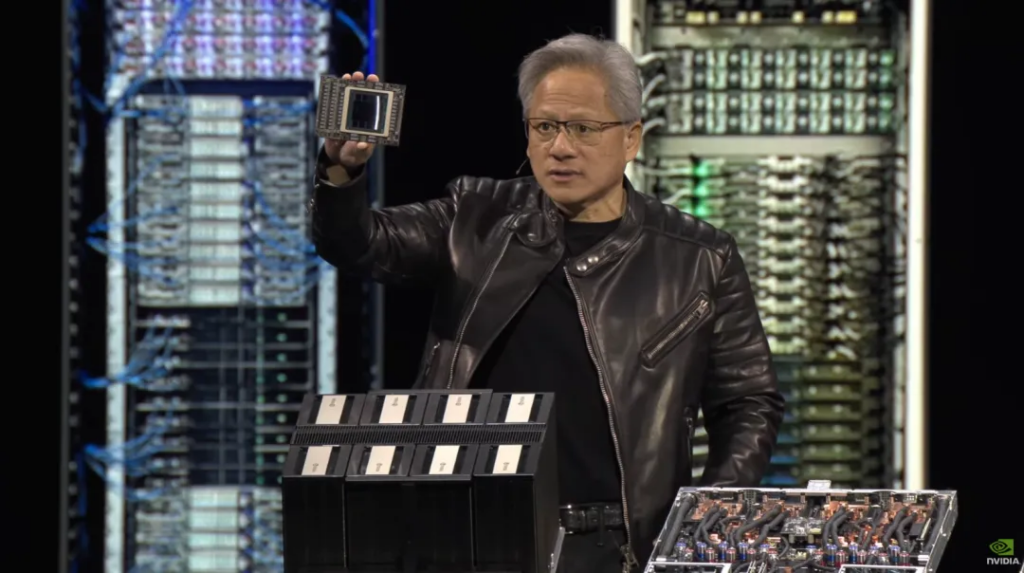
In the future, we need to build such an NVL8 system:
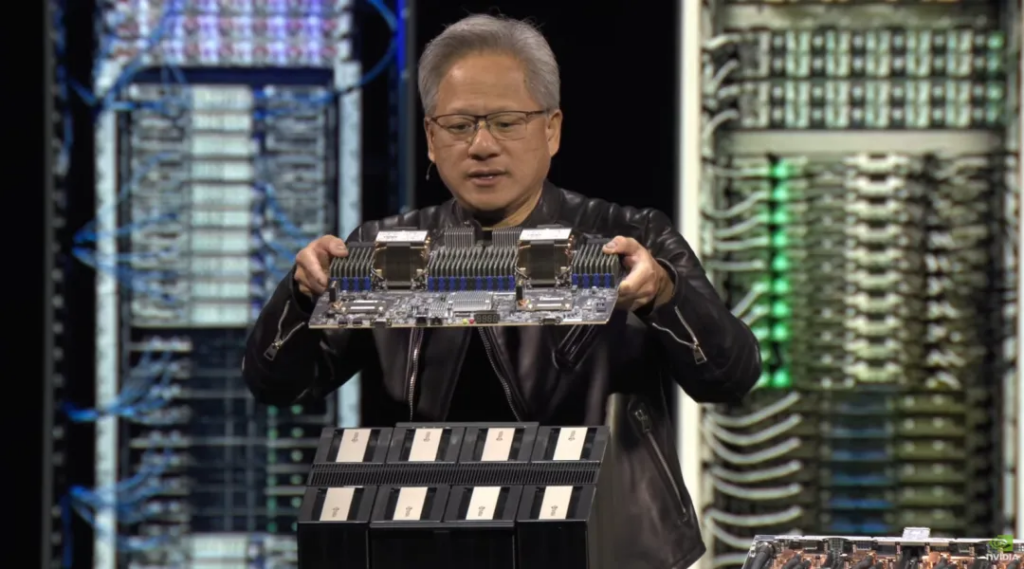
In order to surpass past designs and realize the AI revolution, NVIDIA must redesign the way the NVLink system works to further expand. Huang was very busy here, first showing the NVLink Switch, showing how it should be moved out of the chassis and moved to other rack unit devices. This process should be called “Disaggregated NVLInk”:
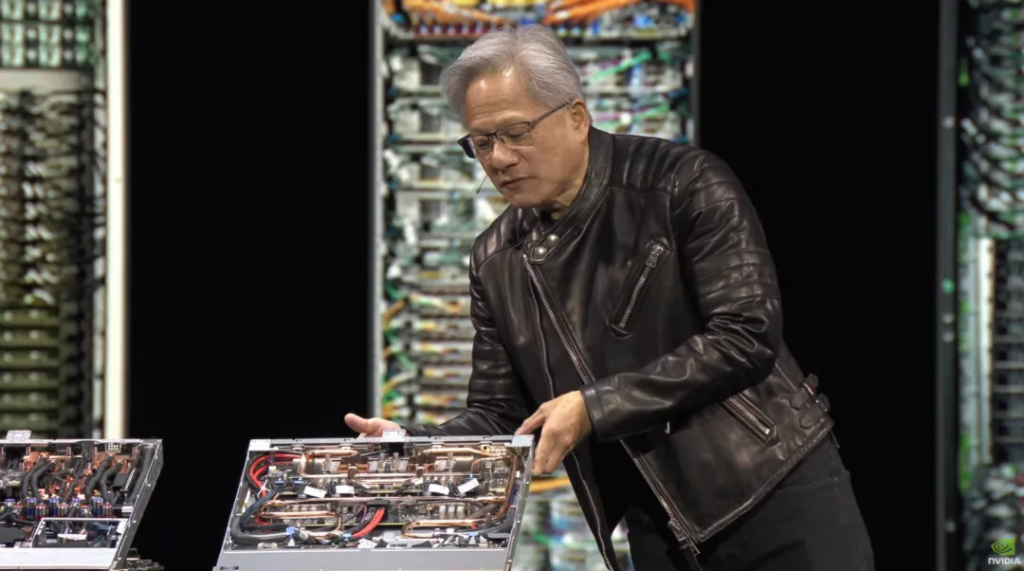
The end result is a single ExaFLOP in a single rack. “ This is the most extreme scaling the world has ever seen. ”
In short, this approach has its limits. As the chip size of the Blackwell GPU is close to the physical limits of the lithography process (reticle limits), Nvidia can no longer improve performance by simply “making a single chip bigger”, so it turns to “using the entire rack as a giant computing unit” to expand computing power, rather than relying on the traditional single server design.
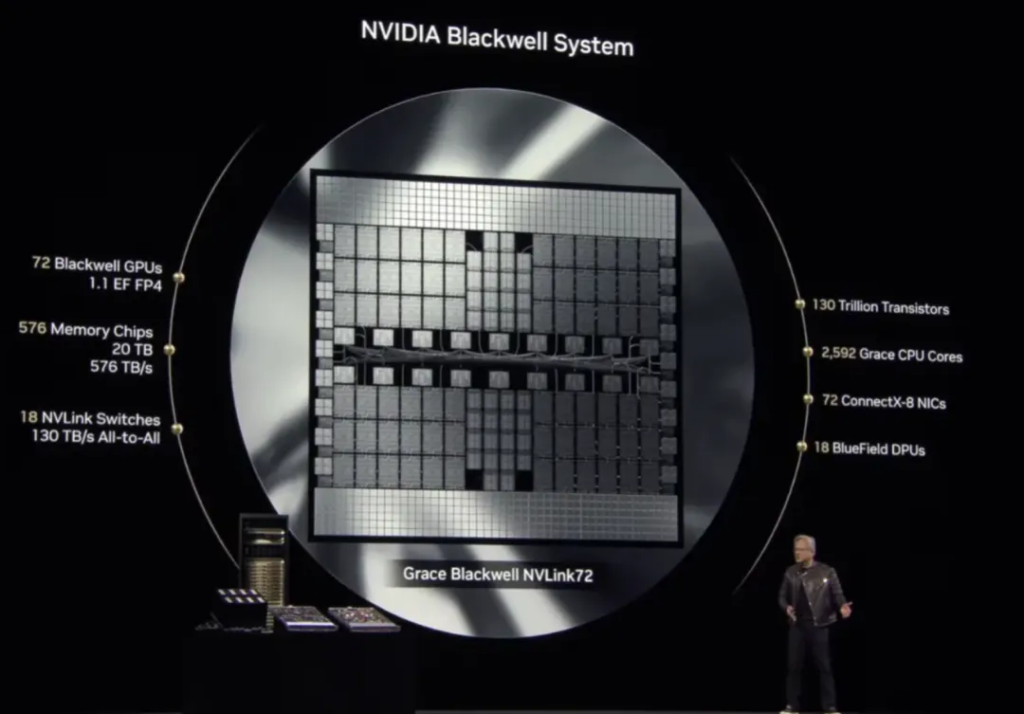
( This picture seems to have been used at CES 2025)
This shift also helps provide computing performance for AI. Not just for training, but also for inference – Huang Renxun showed an inference performance curve for large-scale computing. In short, it’s a balance between total throughput and responsiveness. Keeping the system saturated will maximize token throughput, but the time it takes to generate a single token will be long ( if it takes too long, users may turn to other places ):
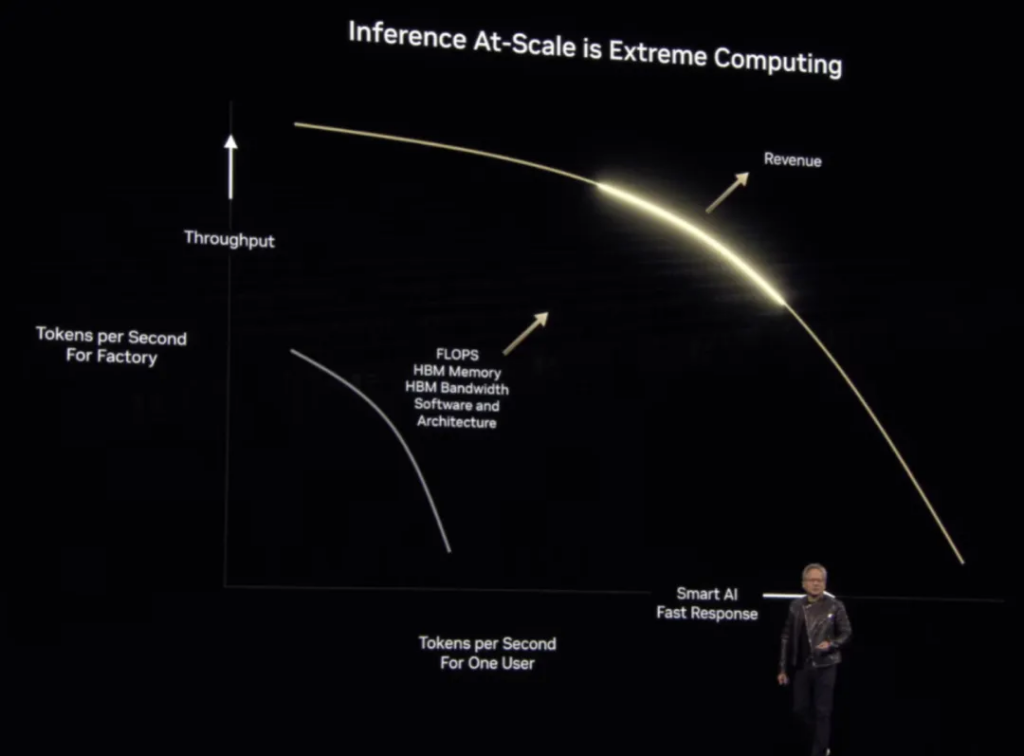
So for NVIDIA CSP partners and other customers using NVIDIA hardware for inference, they need to carefully choose a point on the curve in order to maximize their revenue. Typically, the ideal situation will be to move to the upper right – achieving maximum throughput and responsiveness without significantly sacrificing one side or the other. All of this requires floating point operations and memory bandwidth, so NVIDIA builds hardware to provide these capabilities.
Here is another short video, which is quite interesting and demonstrates the practicality and computational requirements of the inference model:
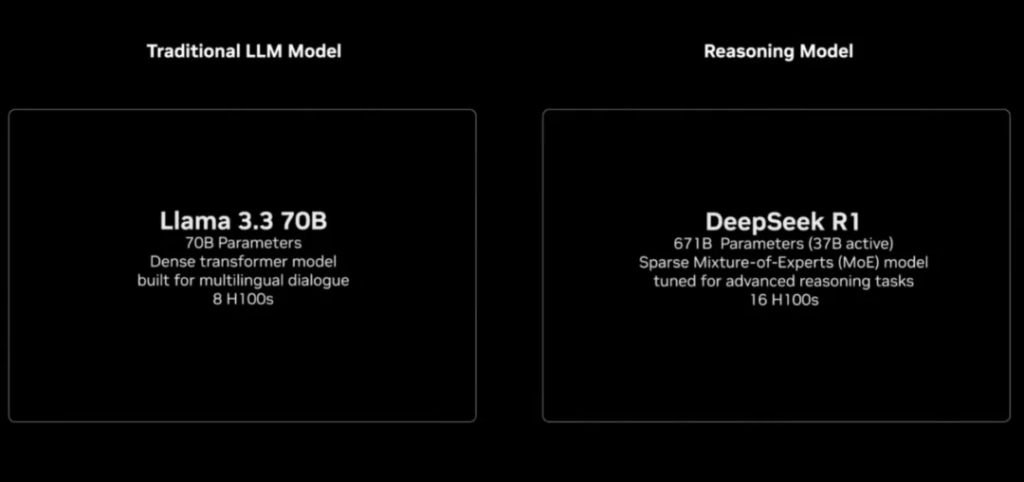
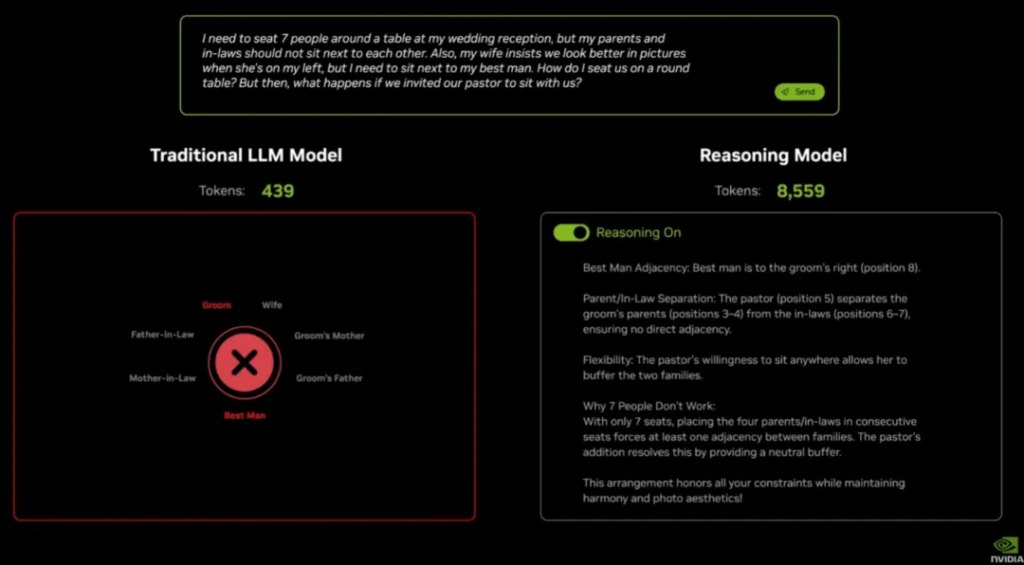
The case here is to let the large model arrange the seating for the wedding banquet.
A traditional text model can be arranged quickly and efficiently, but it is wrong. 439 tokens are wasted; the inference model can handle this problem, but it requires more than 8,000 tokens.
So making this all work efficiently requires not only a lot of hardware, but also a lot of software optimization, and even the operating system to handle basic optimizations like batch processing.
A single GPU cannot meet the extreme requirements of both stages at the same time – pre-filling requires high computing power, and decoding requires high bandwidth, so multi-GPU collaborative optimization is required. After NVLink completes the connection step, an operating system is still needed to perform dynamic task allocation or subsequent optimization to improve GPU utilization, which leads to Huang’s real focus – distributed inference service library NVIDIA Dynamo :
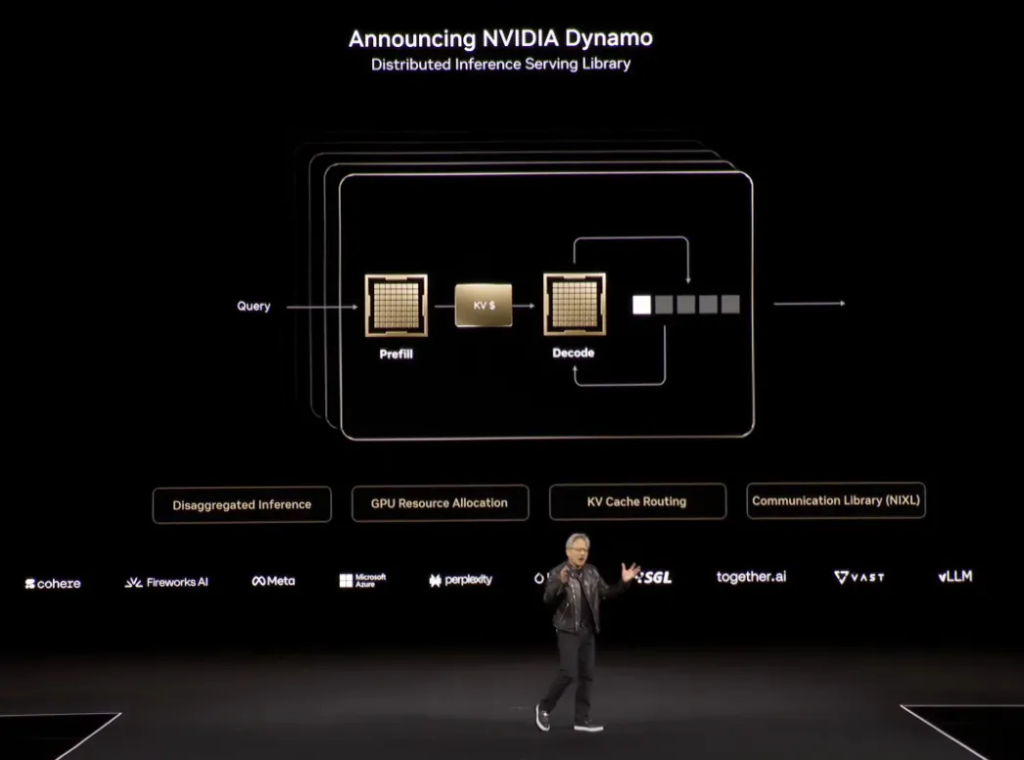
“It is the operating system of this AI factory,” said Huang Renxun.
Dynamo is compared with VMWare. VMWare is built on the CPU system, while Dynamo is built on the GPU system. It is worth mentioning that Dynamo is also open source .
Now back to the topic of hardware and performance. Huang compared the NVL8 Hopper configuration to Blackwell. The following chart shows the tokens per second per megawatt and tokens per second per user for the H series:
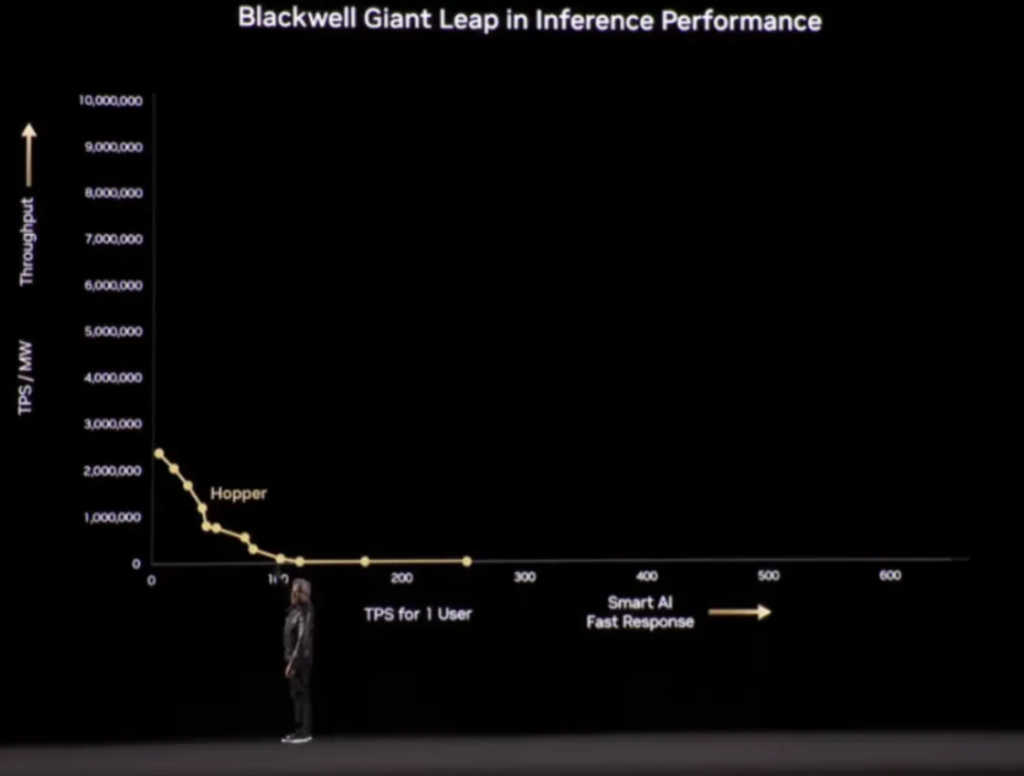
Since there are a lot of data in the charts , Huang wanted to prevent the audience from getting bored and joked, ” Only at NVIDIA will you be tortured by math. “
For service providers, generating a large number of tokens over a long period of time means a large amount of revenue, so when DeepSeek announced a model cost profit margin of 545%, many people were shocked. Blackwell has made some improvements in hardware and supports a lower-precision data format (FP4), so that more data can be processed with the same energy consumption:
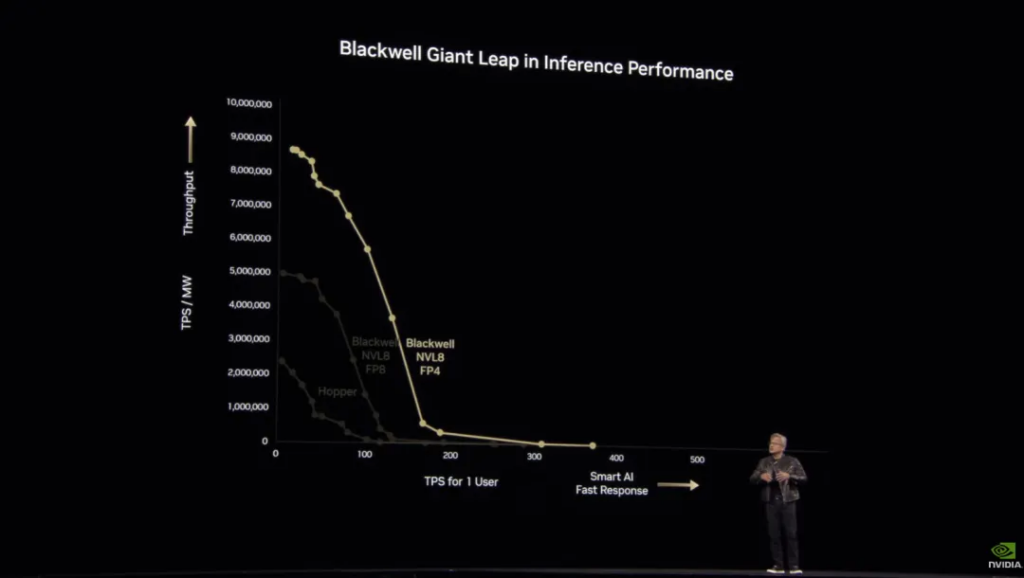
Dynamo can make Blackwell NVL72 faster – and this is at the same power, not the same chip, a generation of improvement of 25 times. The following figure marks the two highlights of the curve in this case, which Huang calls “Max Q” , which is the balance between the maximum throughput and the highest quality of artificial intelligence:
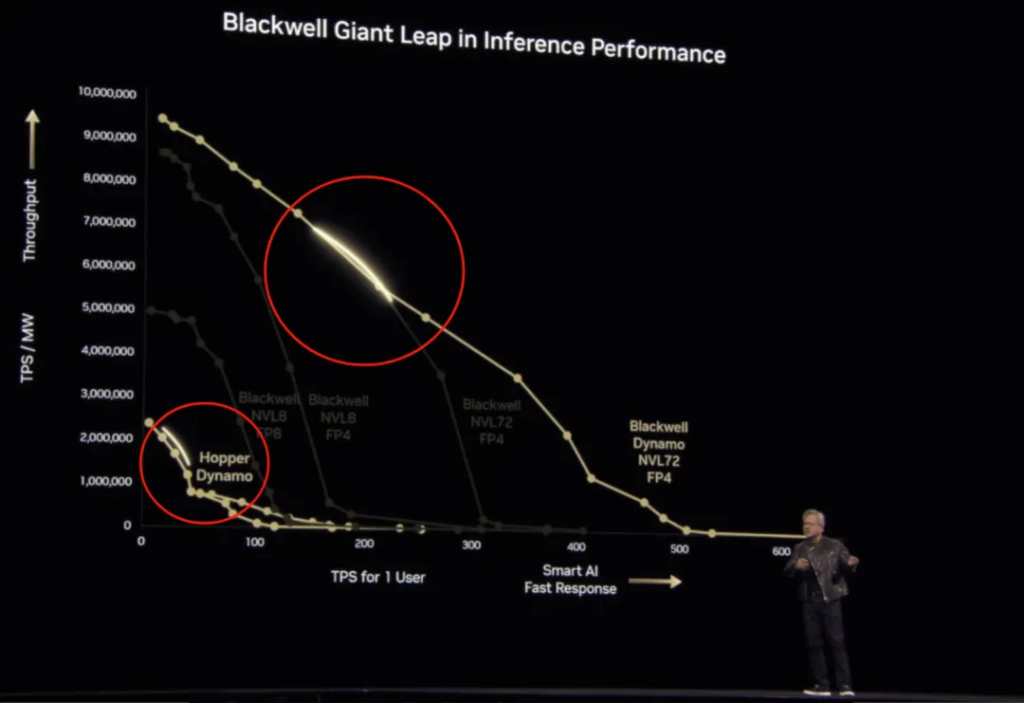
Finally, on the Pareto optimal frontier of this curve ( in a distribution system, there is no state in which it is possible to make one individual better off without making any other individual worse off through redistribution ), Blackwell can achieve 40 times (equivalent power) performance of Hooper:
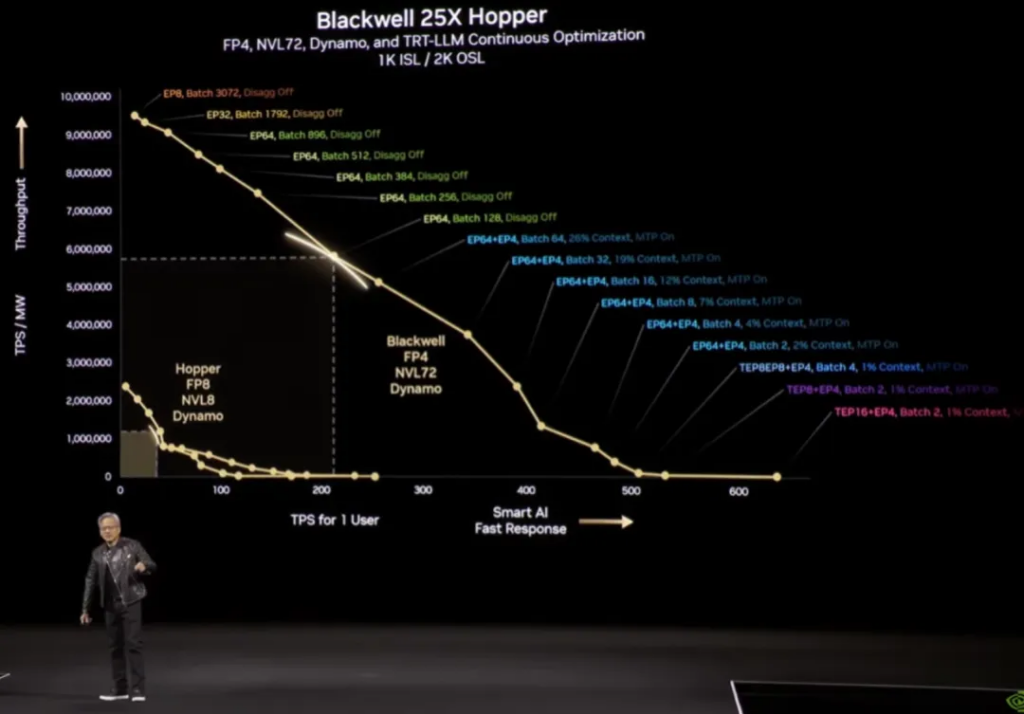
Huang’s sales pitch here is: “The more (Blackwell) you buy, the more you earn.”
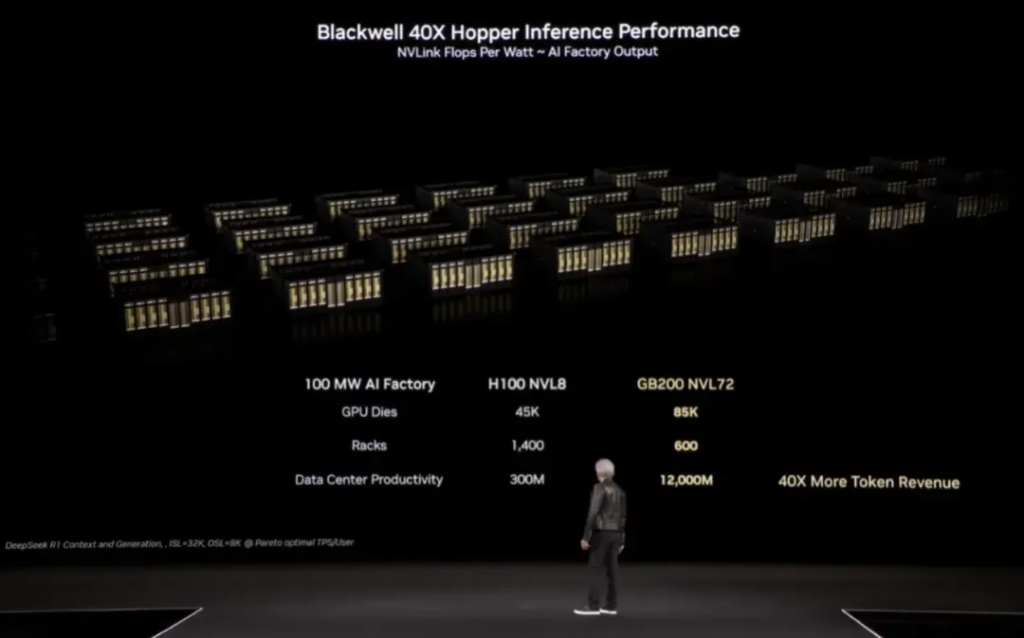
By the way, the audio equipment here popped, which affected Huang Renxun, and he paused awkwardly for about a second. It has to be said that even such a top technology speech occasion is bound to have some minor problems.
The next short video shows how NVIDIA builds digital twins for various data centers. This process is actually the so-called “AI factory”. The use of digital twins can plan and optimize factory processes in advance, and ultimately achieve a one-time build:
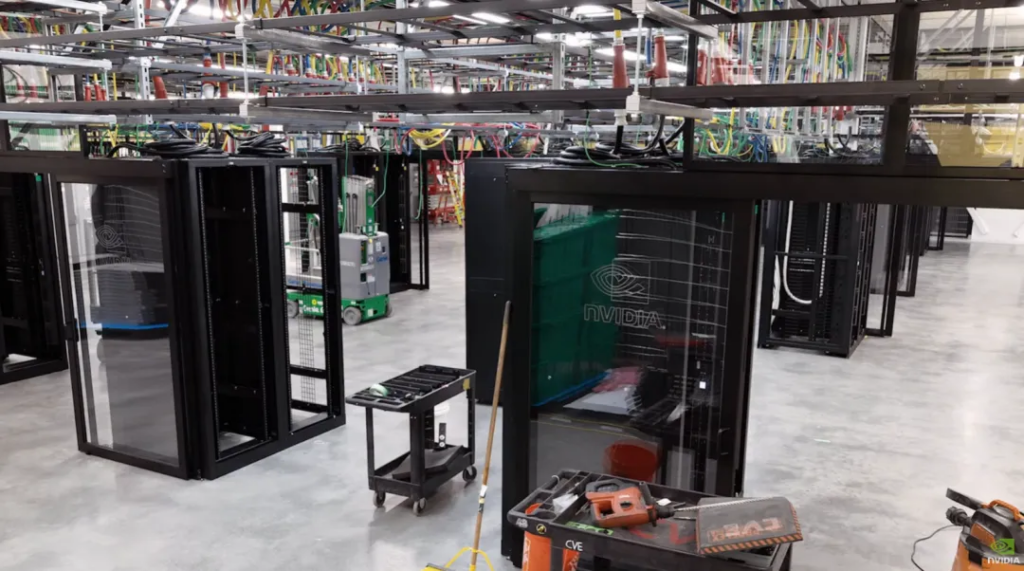
Next is the release of the next-generation chip architecture. Since we have already written about the parameters at the beginning of the article, we will only write about some details in the speech here.
Blackwell Ultra NVL72 will be shipped in the second half of this year. Now all industries are at a stage where they must plan their spending, that is, to place multi-year orders for Nvidia’s hardware, infrastructure and ecosystem. So Huang hopes to clarify Nvidia’s future roadmap and plan it directly to 2028.
Rubin in the second half of 2026 consists of Vera Rubin NVL144, which consists of Vera Arm CPU + Rubin GPU:
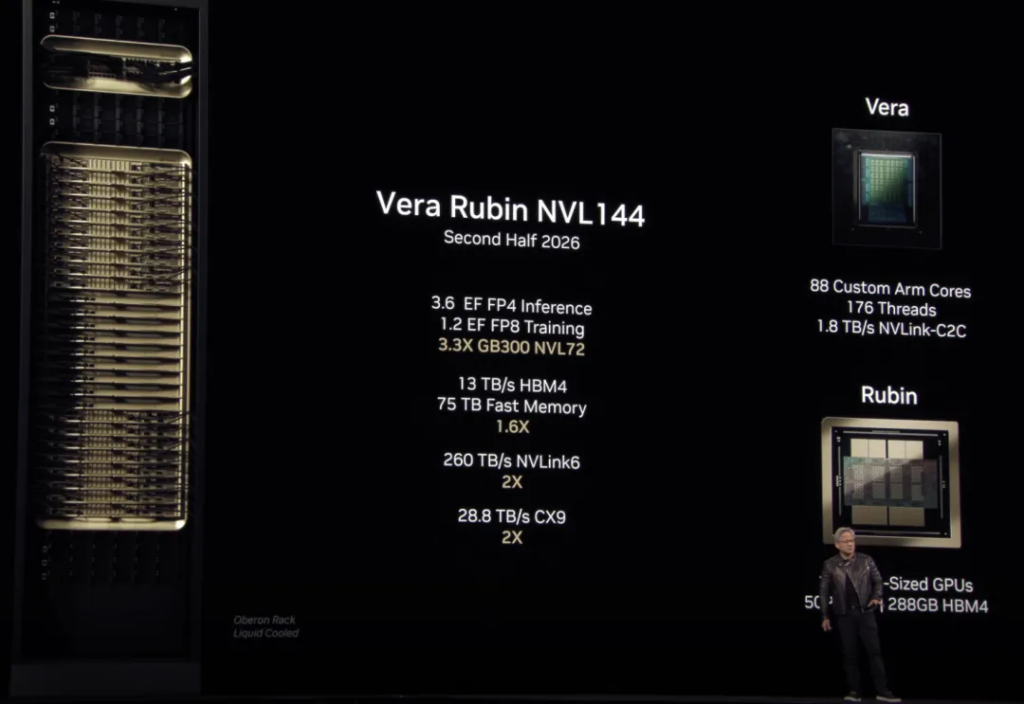
Huang Renxun emphasized that in the future, when NVIDIA discusses the NVLink interconnection domain, it will no longer use the “number of GPU chips ” as the unit, but will use the “number of GPU core dies” as the statistical standard.
For example, “NVL144” means that the NVLink domain contains 144 GPU core dies, not 144 independent GPU chips.
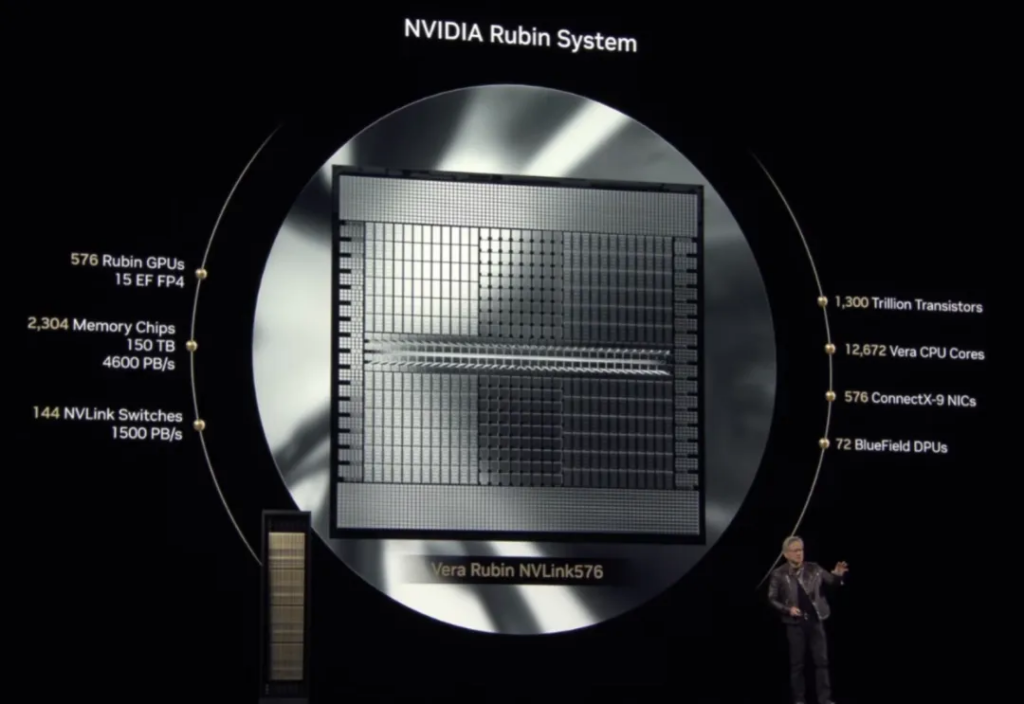
Then there’s the Rubin Ultra NVL576 in the second half of 2027. “ You should gasp when you see this. ”
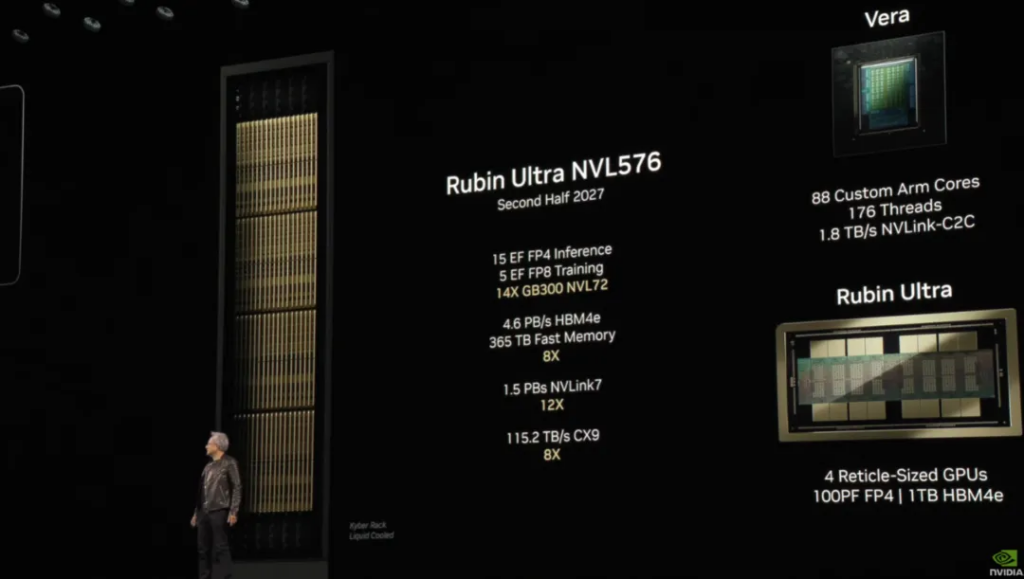
Rubin will dramatically reduce the cost of AI computing.
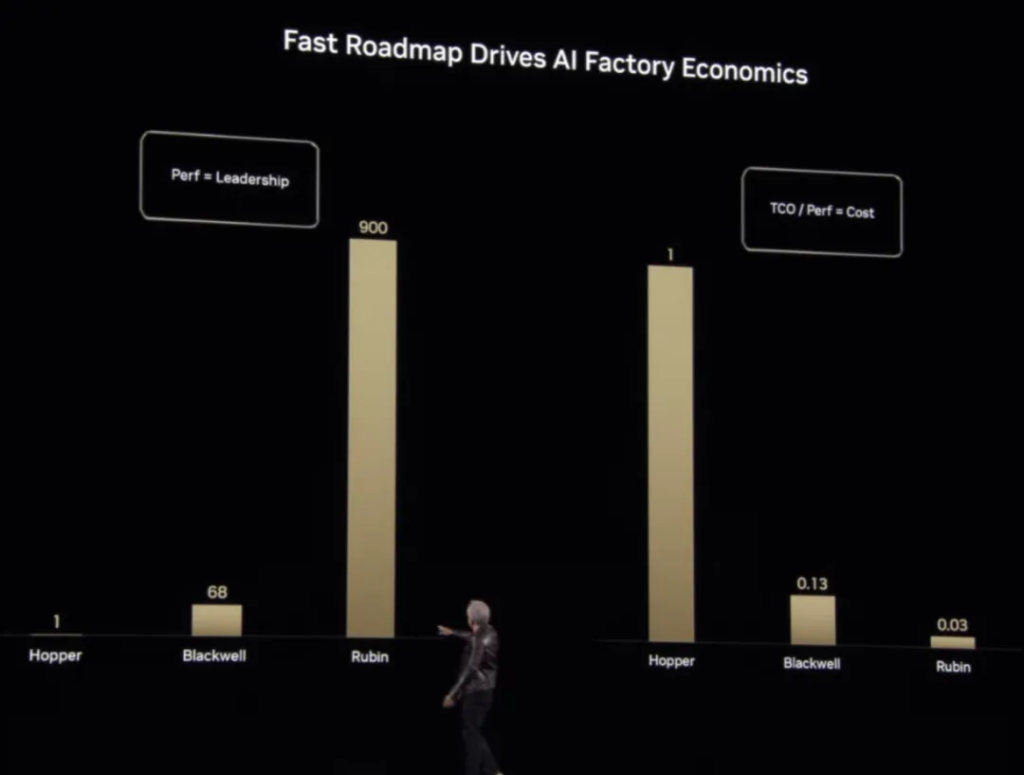
04
Further expanding the moat
At the beginning of this paragraph, Huang Renxun recalled that six years ago, NVIDIA acquired Mellanox for US$6.9 billion. This acquisition later created the industry’s first Ethernet network platform designed specifically for AI, NVIDIA Spectrum-X. In fact, it was for six words: to enter the network market.
So Huang also launched the latest NVIDIA network cards CX-8 and CX-9, hoping to expand GPUs to hundreds of thousands or even more in the Rubin era (2026).
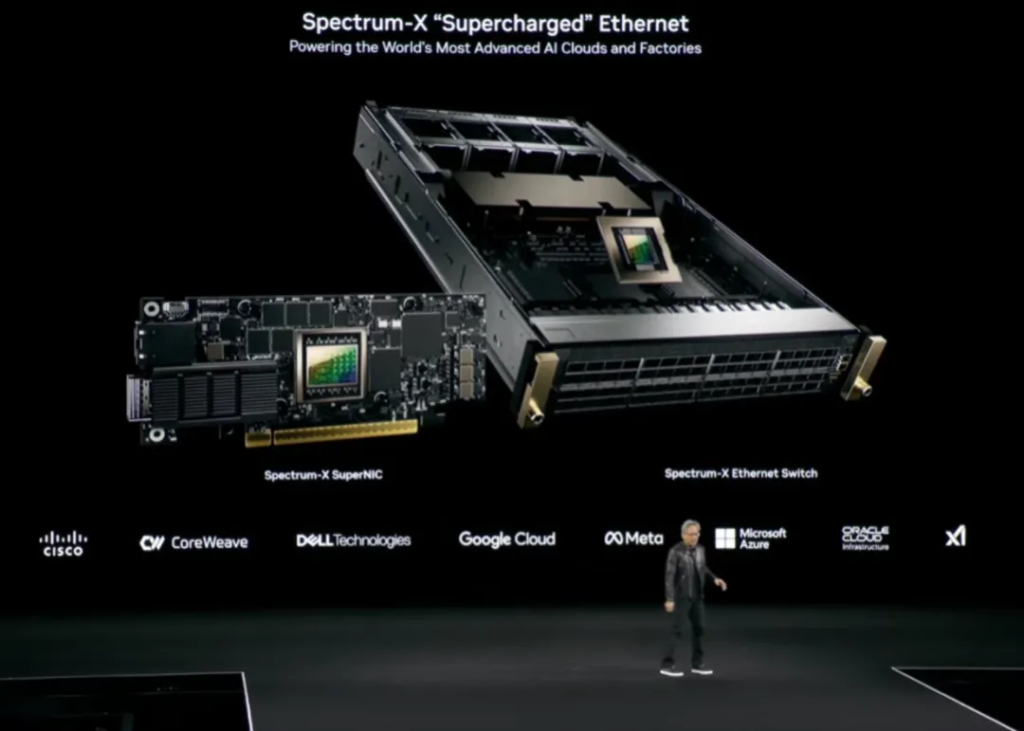
As Nvidia expands, its data centers will be as large as stadiums. At that point, copper connections won’t be enough, and optical connections will need to be used—and optical connections can be very energy-intensive. Therefore, Nvidia plans to make optical networks more efficient by co-packaging silicon photonics technology Photonics .
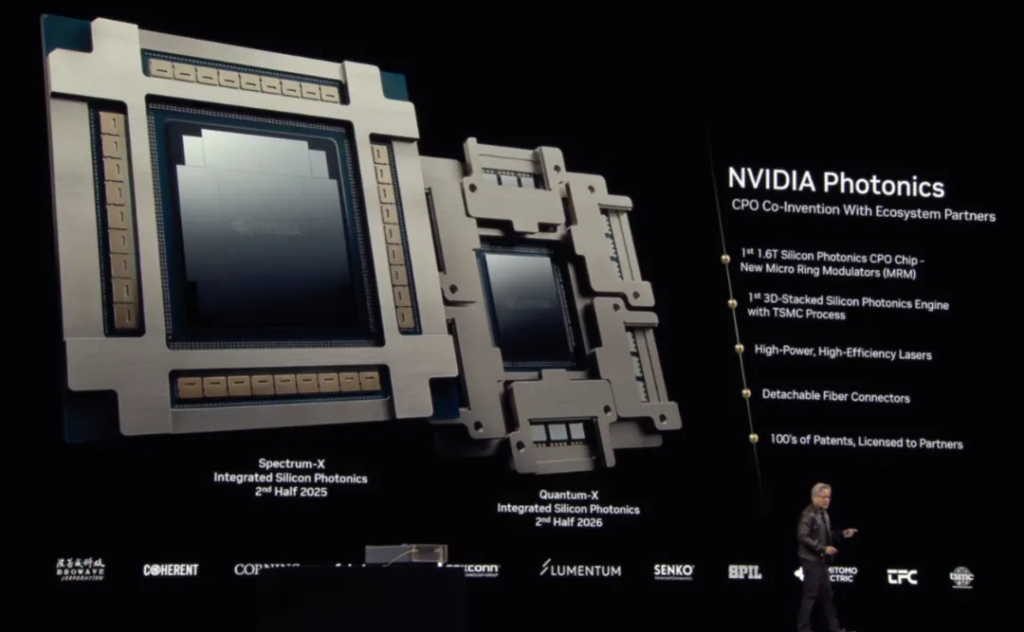
The new 3D stacking process Nvidia is developing in collaboration with foundries is based on a technology called micro-ring modulators (MRMs).
Here, Lao Huang brought a bunch of cables to the stage, and then found that he couldn’t untie them:
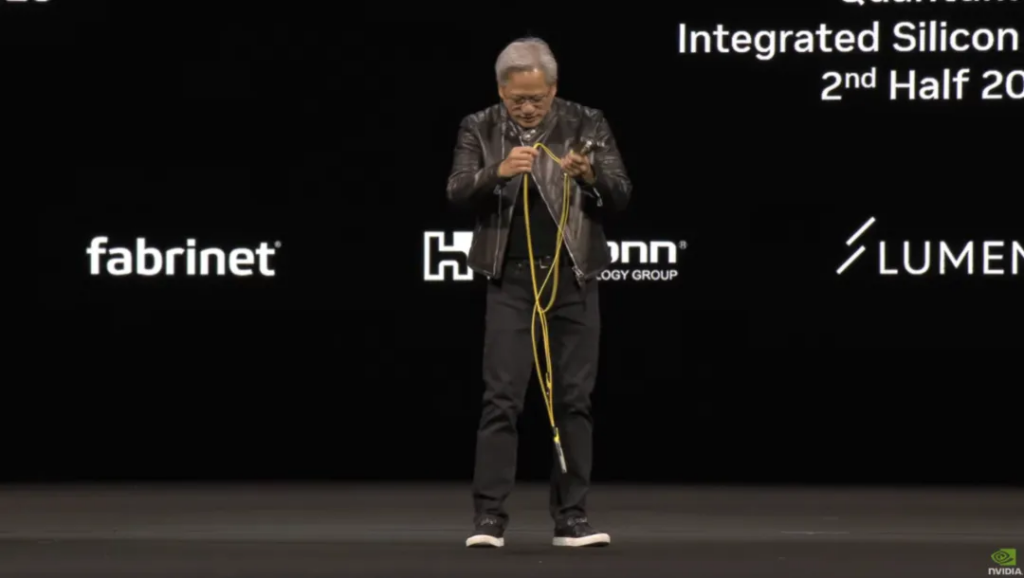
“Oh mother of god.”
After untying it, he showed a relieved smile:
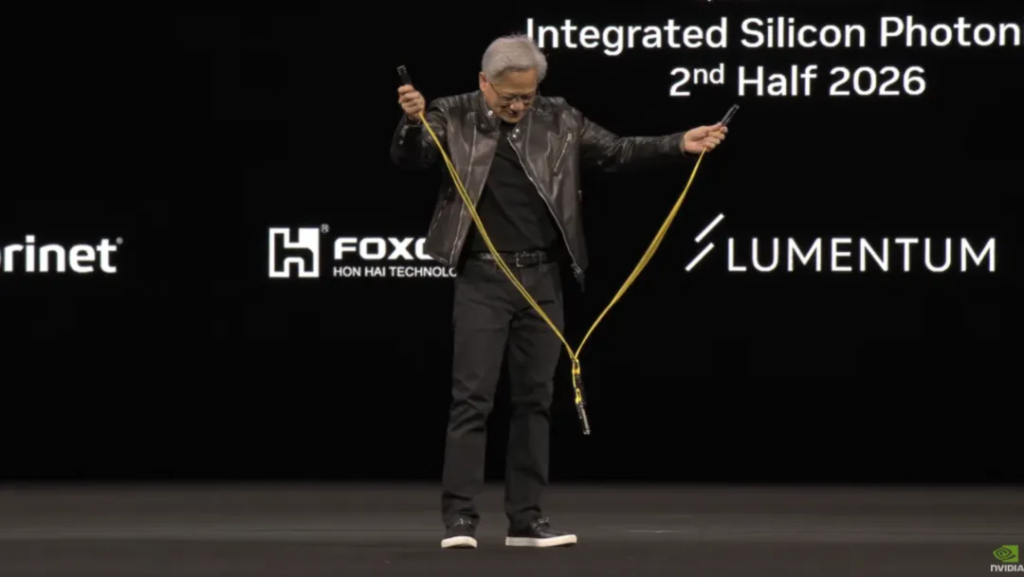
Huang explained how optical networks work today. First, each port on each side of the two wires has a separate transmitter. This is reliable and efficient, but the conversion of electricity to light (and back to electricity) consumes a lot of power.
And, “each GPU will have 6 transceivers.” This will consume 180 watts (30 watts more each) and require thousands of dollars in transceivers. All the power consumed by the transceivers is power that could be used for the GPU. This prevents Nvidia from selling more GPUs to customers.
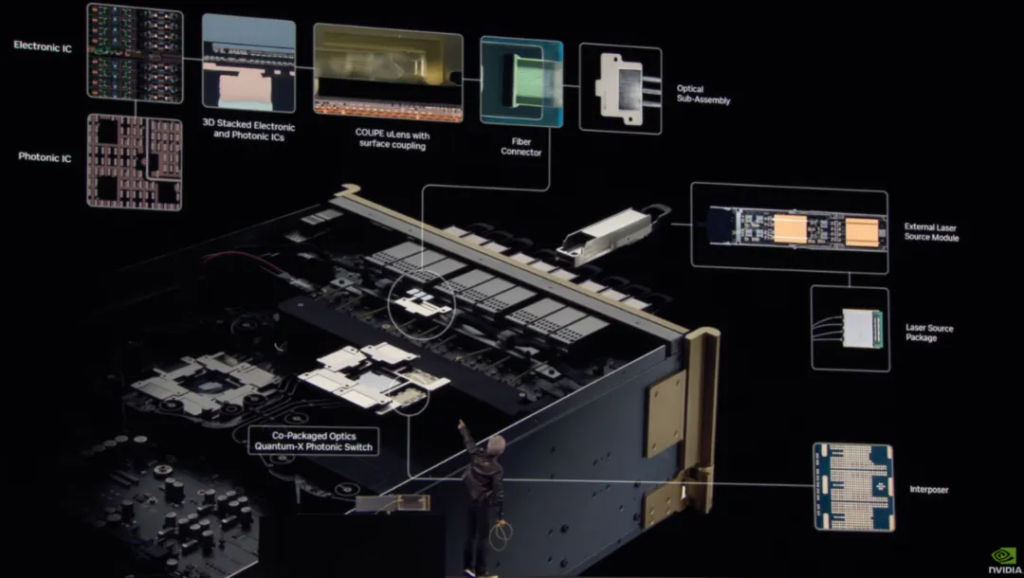
This speech certainly wouldn’t raise any unsolvable problems, so as expected, it was followed by another commercial break.
After a short video introducing the optical principles, the silicon photonics Quantum-X (InfiniBand) switch was officially unveiled and will be released in the second half of 2025. In the second half of 2026, another Spectrum-X switch will be released. It has no optoelectronic transceiver, direct optical fiber input, and can support up to 512 ports.
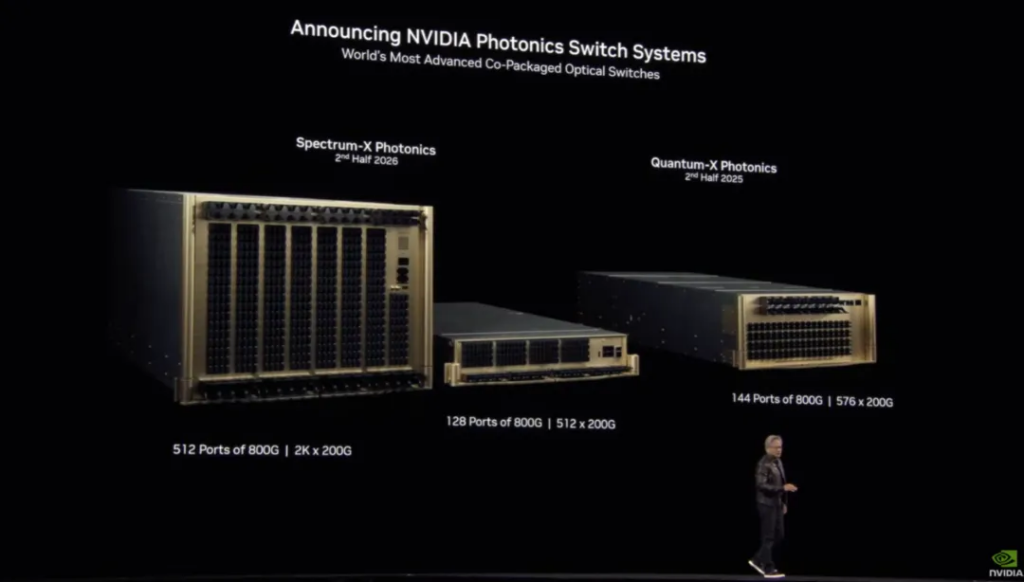
With this savings, the data center could add 10 Rubin Ultra racks.
Huang showed another roadmap here, saying that NVIDIA will now launch a new platform every year , and also announced the next-generation architecture – Feynman .
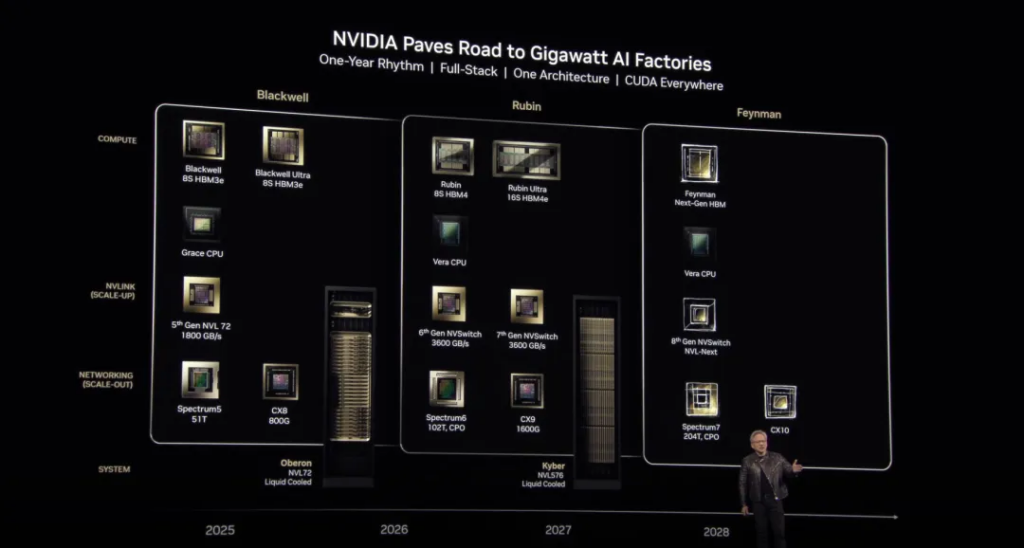
Now let’s talk about the enterprise. “ AI and machine learning have reinvented the entire computing stack. The processors are different, the operating systems are different, the applications on top of them are different, the way you orchestrate them is different, the way you run them is different. Let me give you an example: the way you access data in the future will be completely different than it was in the past. It’s no longer about retrieving exactly the data you want, but about humans generating confusion, asking a question, and letting the AI tell you the answer.”
“This is how businesses will work in the future. We have AI agents that are part of our digital workforce. There are a billion knowledge workers in the world — and in the future there will probably be 10 billion digital workers working alongside us, eventually 100 percent software engineers.”
“ I am convinced that by the end of this year, 100% of NVIDIA software engineers will be assisted by artificial intelligence. Agents will be everywhere, and what companies operate and how we operate will be fundamentally different. ”
“Therefore, we need a new computer production line.” – After saying this, the supply at the most important point was cut off , and this really caused a big problem.
The official website also briefly switched from live broadcast to recorded broadcast. I recorded this precious moment (the screen was stuck on this mini computer):
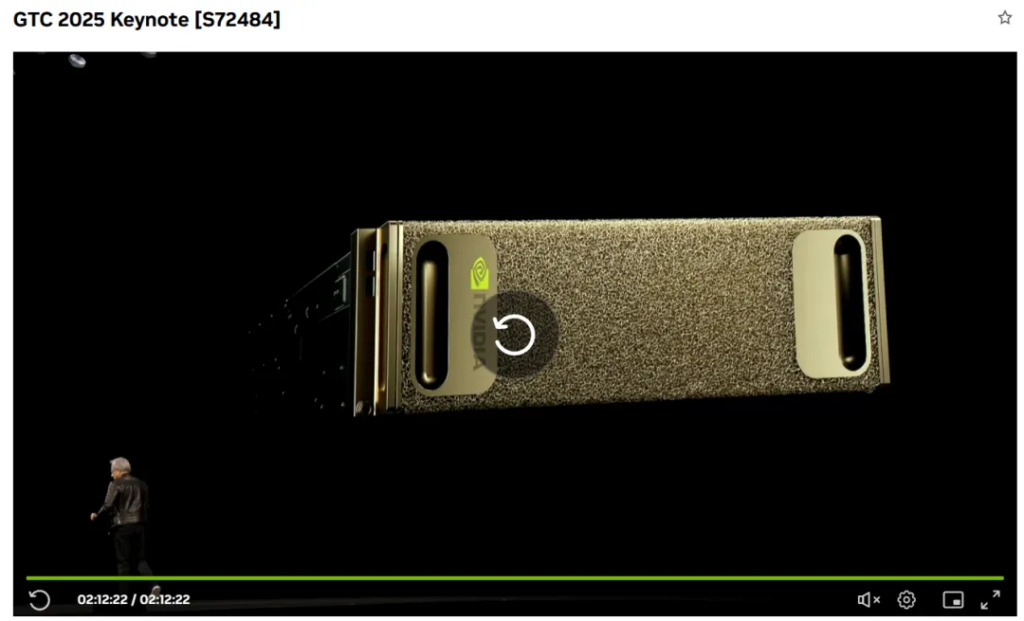
After resuming the live broadcast and replaying it, I found that the interrupted part in the middle was not filled in, and it jumped directly to the next part.
There’s a joke that goes, the whole world is made up of makeshift teams!
Fortunately, we know that DGX Spark is actually being released here . This is the final name of Project DIGITS mini computer announced by Nvidia at CES 2025. It will also have an enhanced version, the mini workstation DGX Station .
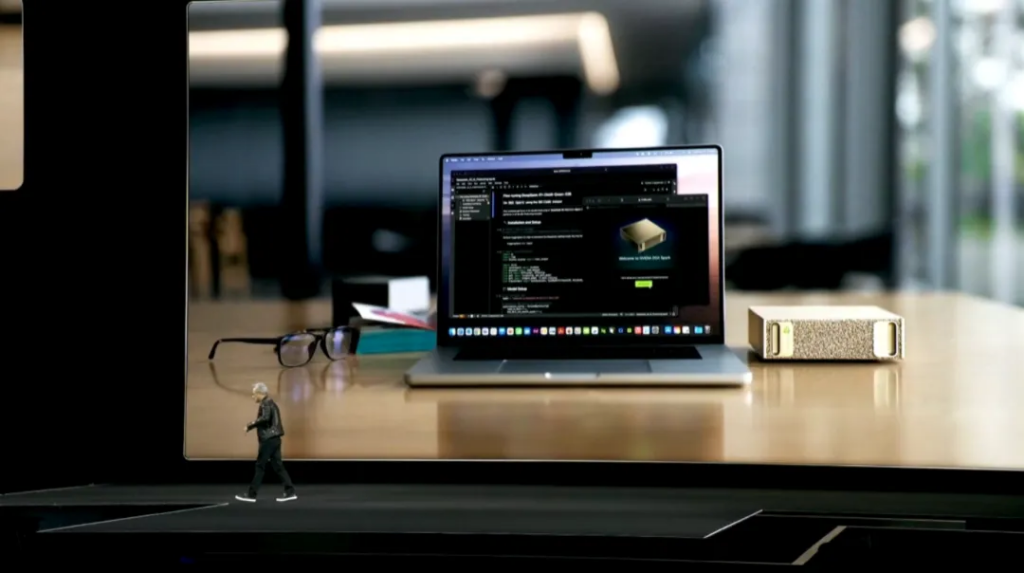
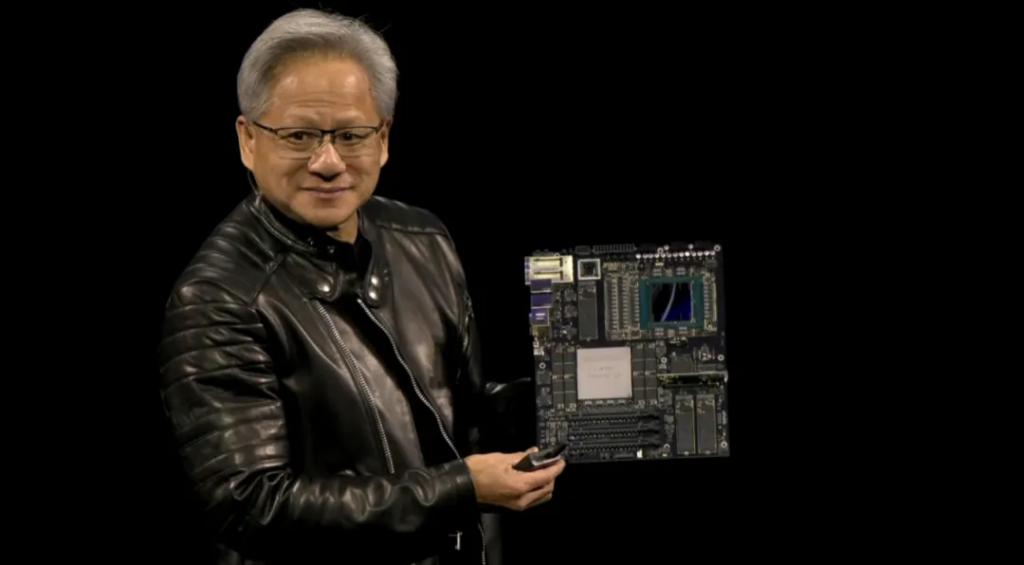
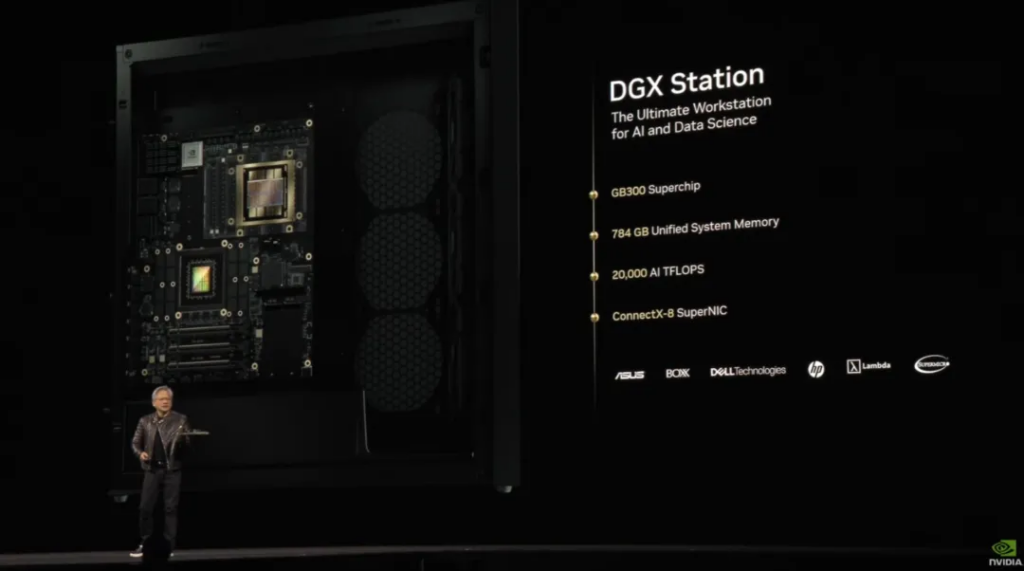
GPU-accelerated storage. NVIDIA has partnered with all major storage vendors.
Then there’s the new partnership, where Dell will offer a range of NVIDIA-based systems.
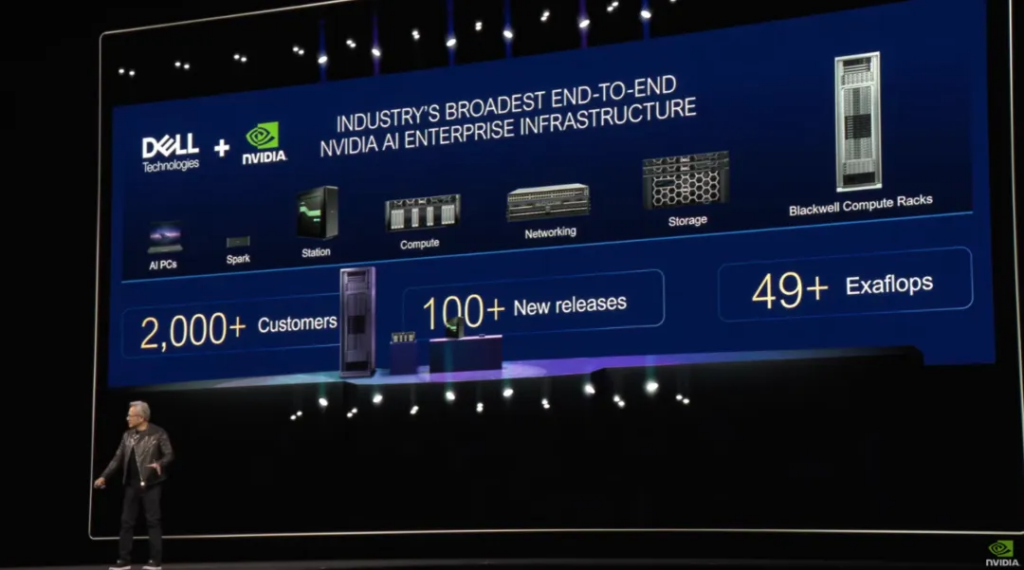
At the end of this paragraph, the NVIDIA Nemo Llame Nemotron model announced at CES 2025 is mentioned again – this time with a Reasoning suffix, which stands for reasoning. Because it is an open source model, the chart here also compares Llama 3.3 and DeepSeek R1 Llama 70B .
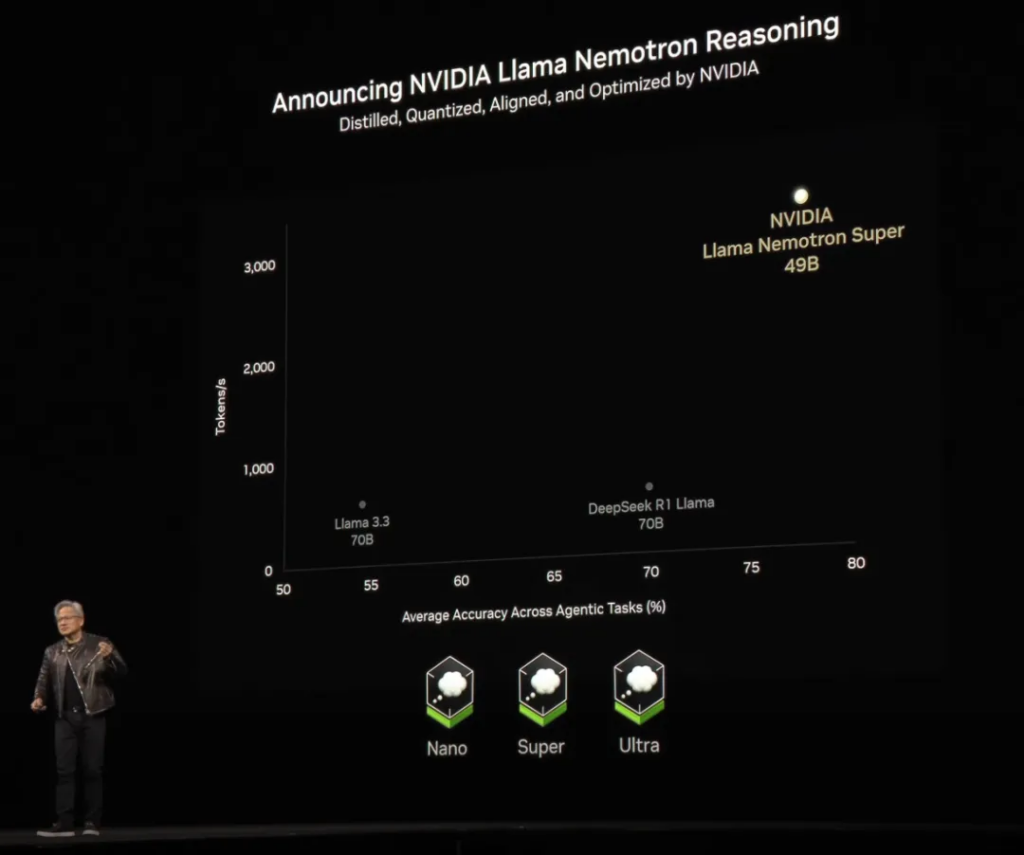
05
The era of general-purpose robots has arrived
The show started with a short film, and the much-anticipated robot segment finally arrived!
Let me start by telling you about the current situation: “ The world is seriously short of human workers. ”
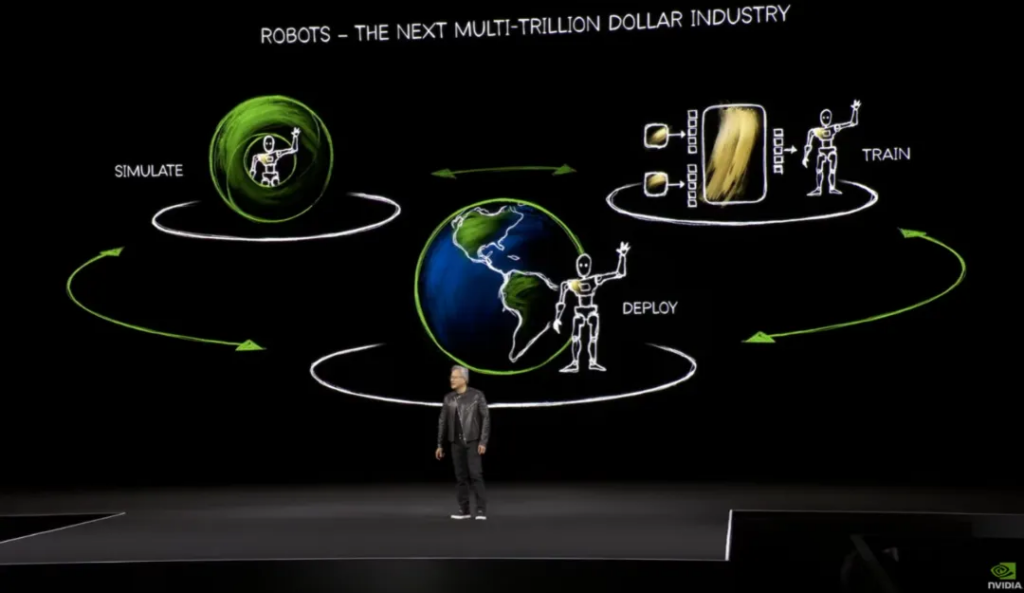
The short film content is mostly a review of Nvidia’s previous embodied intelligence videos . For example, using digital twins to create a virtual facility to help train robots. (When the robot makes a mistake in the virtual world, nothing will be damaged.) These robots will be trained through AI simulations of the physical world.
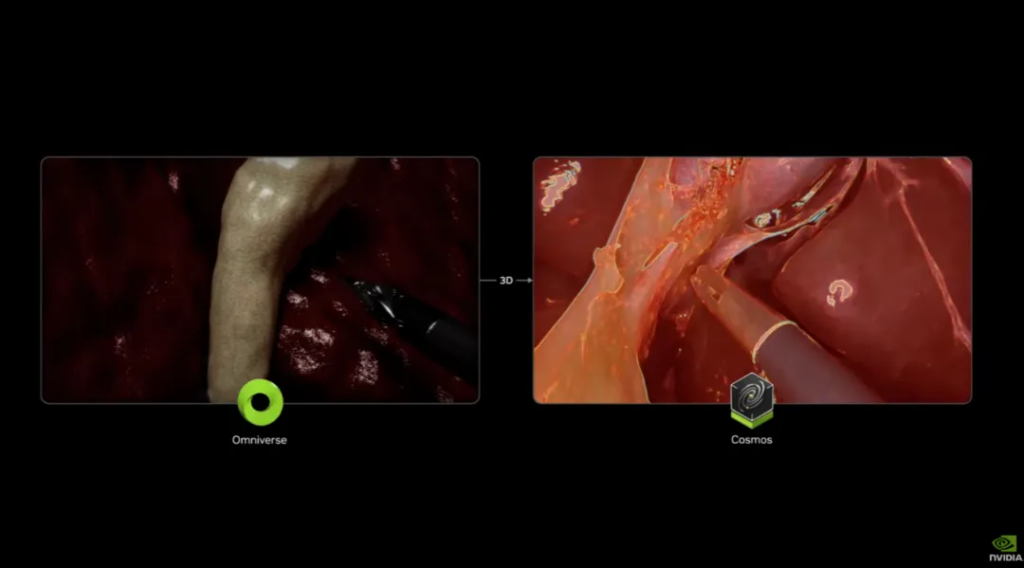
After a round of preparation, the protagonist officially appeared on the stage. NVIDIA released the universal basic model of humanoid robots NVIDIA Isaac GROOT N1 : As mentioned at the beginning, tokens can explain everything. Here, the perception token and text token are respectively input into the visual language model of slow thinking (System 2) and the diffusion Transformer model of fast thinking (System 1), and finally the action token is output to the robot to make it respond.
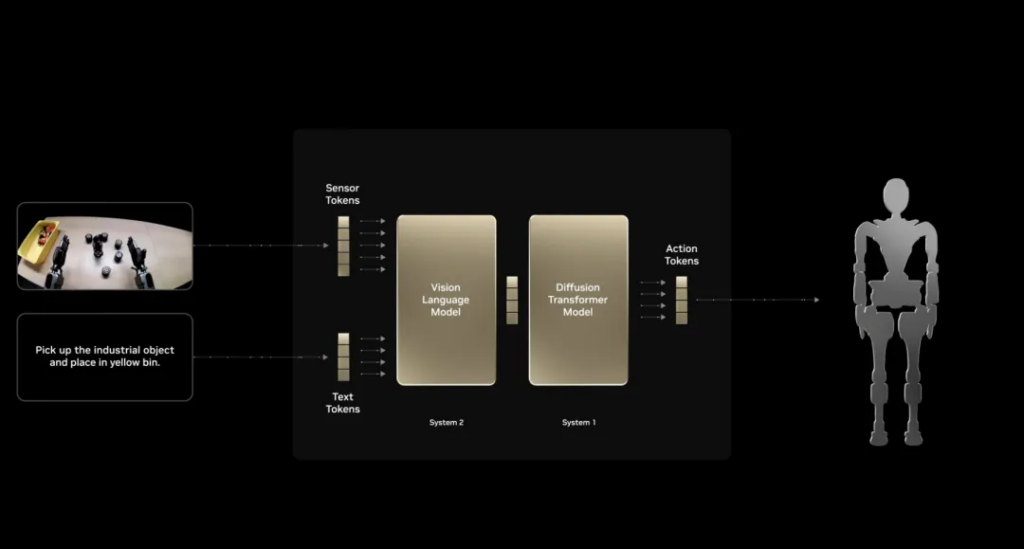
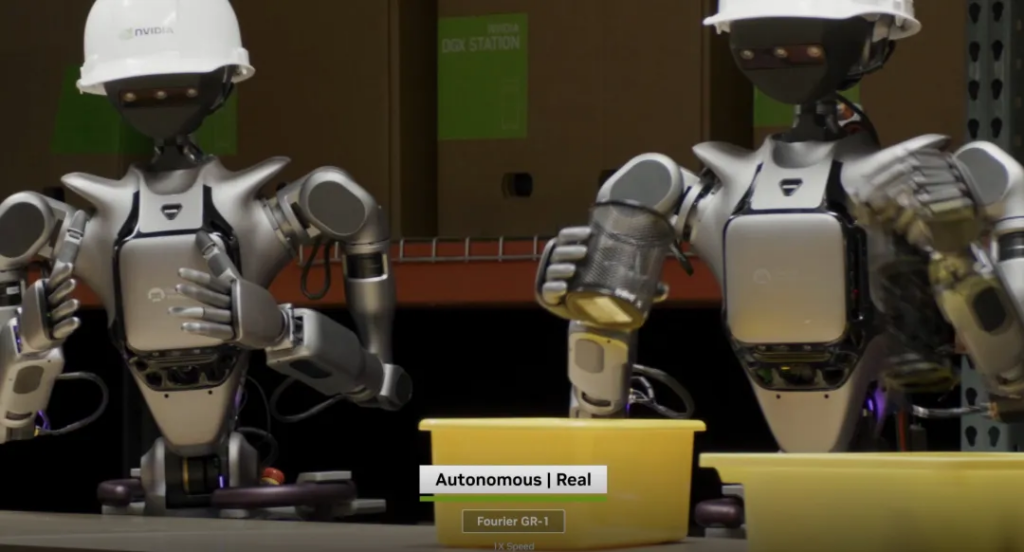
Huang made a prediction here: “Physical AI and robotics are developing so fast. Please pay attention to this field. This is likely to become one of the largest industries.”
Echoing the growth chart at the beginning:
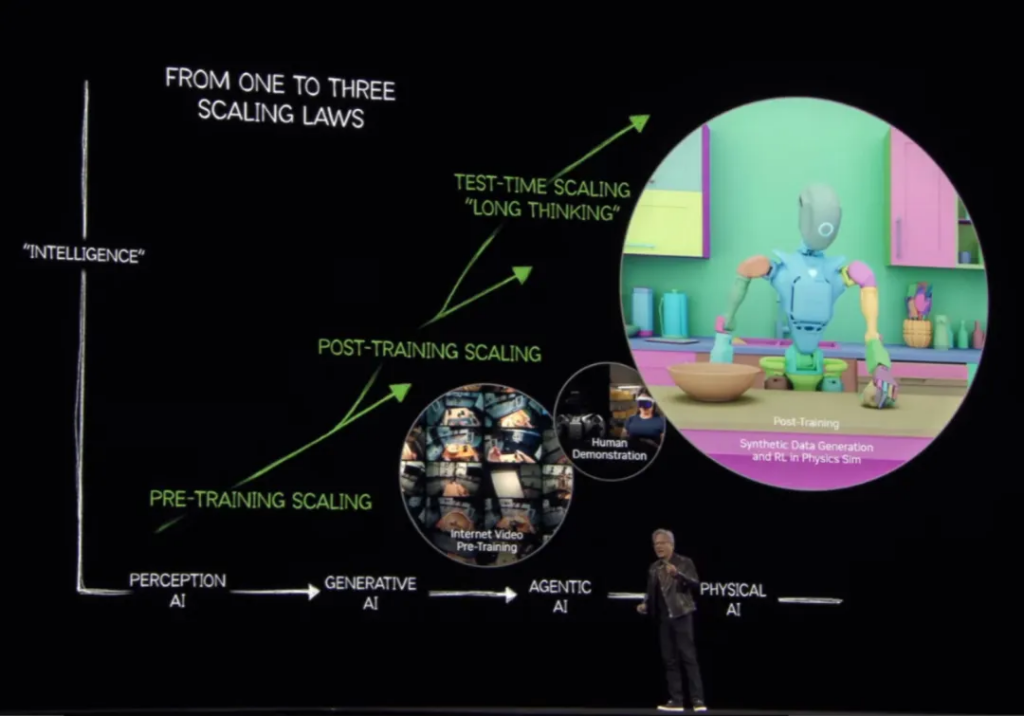
Huang also reviewed how the Omniverse + Cosmos simulation works. Using Cosmos to create various environments to help with training, this process is similar to the current reward model, and a verifiable reward needs to be set.
In robotics, the verifiable reward is physics. If the robot behaves in a physically correct way, then this can be verified to be accurate.
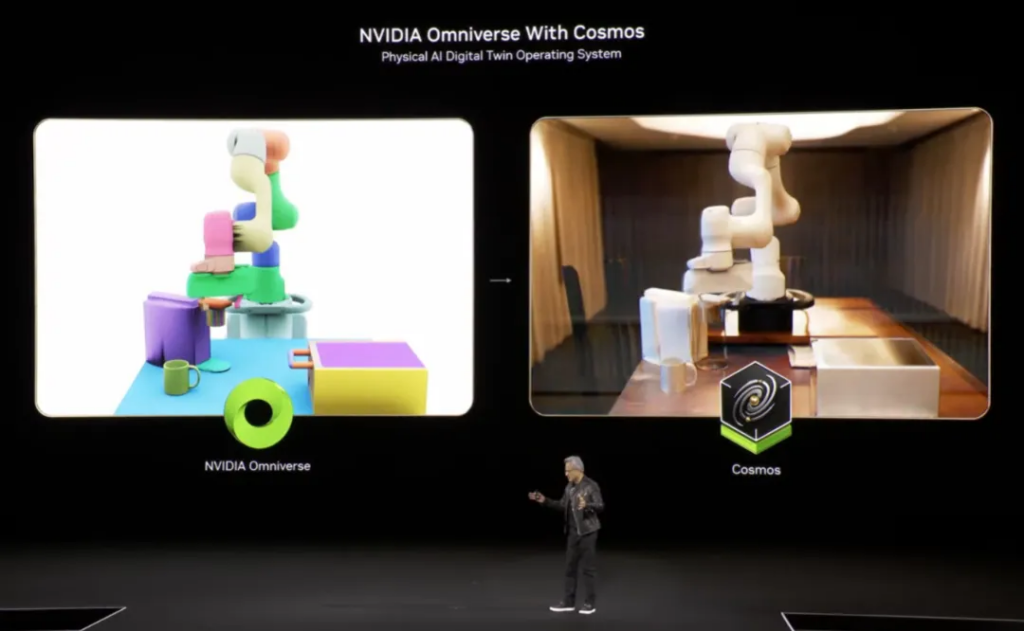
In the next short film, our protagonist , the Newton Physics Engine , officially debuts:
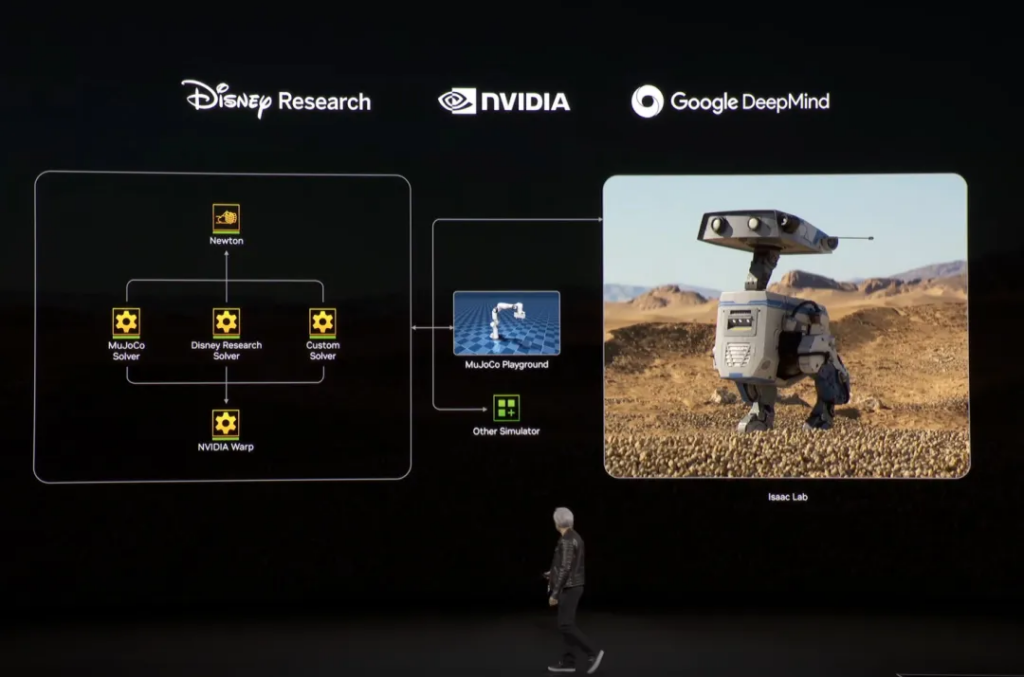
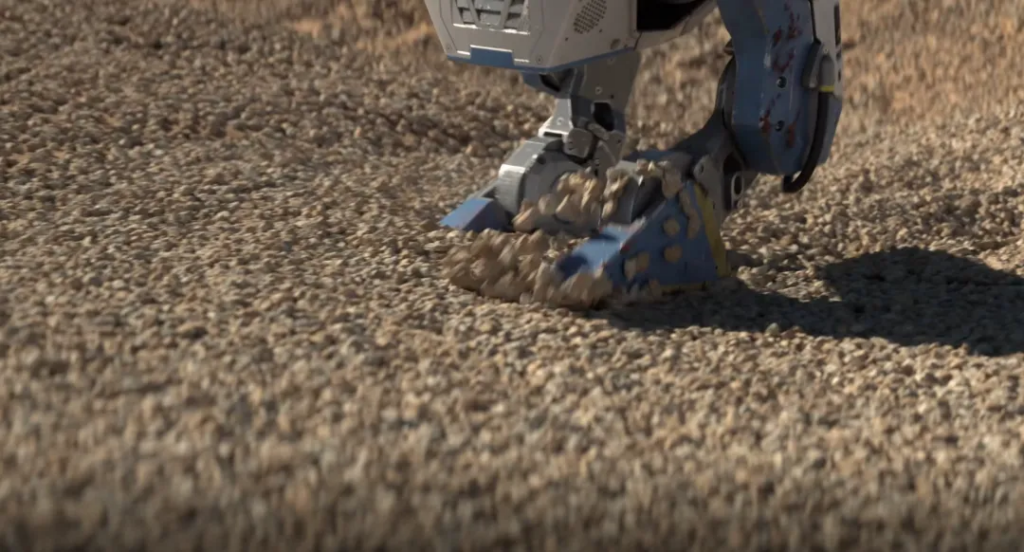
This time, the short film is no longer a short film. Through Newton, Disney’s short film has been transformed from digital to reality, allowing the robot Blue to stand in front of Huang Renxun.
“Let’s end this main meeting. It’s time for lunch.” It was also at this moment that Huang announced that GR00T-N1 was open source, which set off the scene.
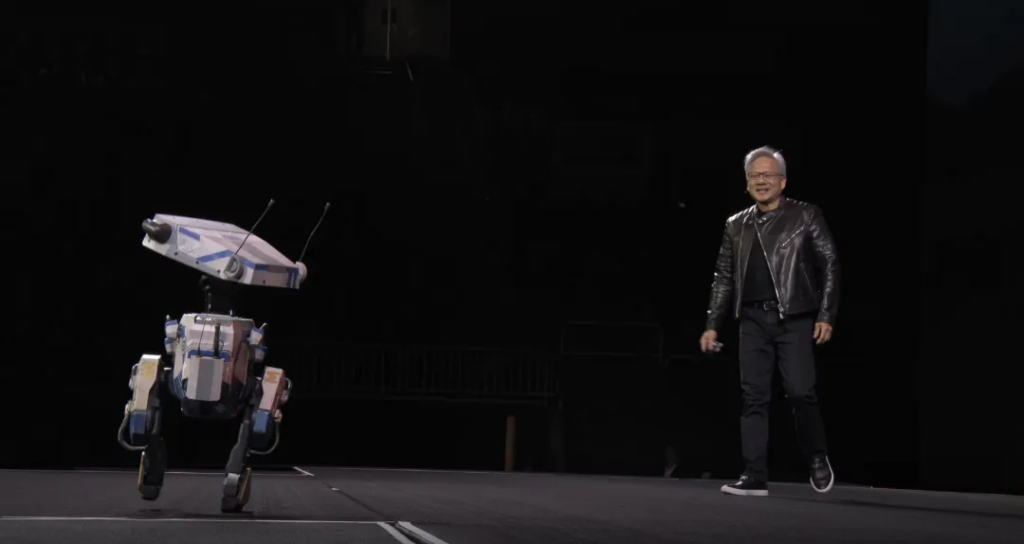
After a round of summary, it is natural to end with a short film.
Nvidia’s technology ranges from games to computer vision, to AI, autonomous driving, and humanoid robots. An “AI factory” has sprung up, which ultimately echoed the beginning of this speech. The Nvidia headquarters that Huang invited everyone to enter transformed into a spaceship and flew into the boundless universe.
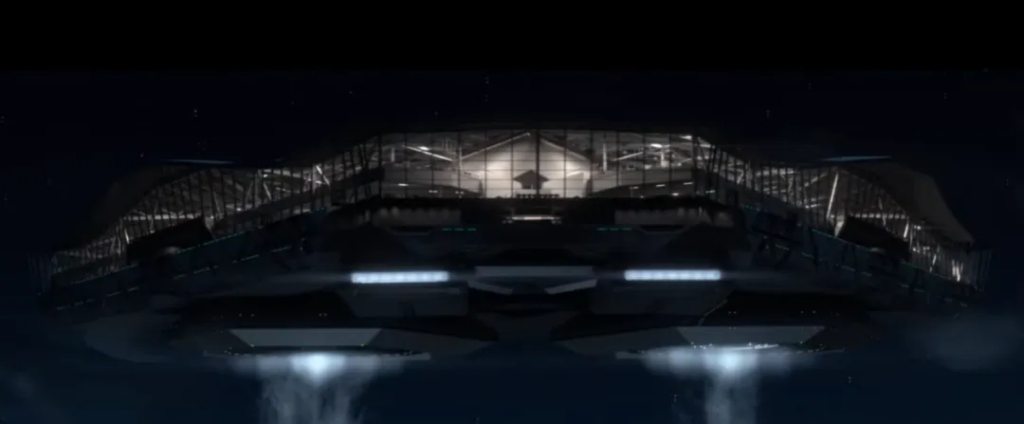
NVIDIA GTC 2025 redefines the boundaries of AI computing power with Blackwell Ultra GPUs, photonic network switches, and the open source robot model GR00T-N1. From chips approaching physical limits to rack-level “super GPUs”, from quantum computing laboratories to desktop-level AI supercomputers, Huang Renxun’s “AI Factory” is bringing science fiction scenes to life.
The final chapter of this technological carnival may be the starting point of the sea of stars.
Author:AI科技大本营
Source:https://mp.weixin.qq.com/s/f6G_p5Sw4gslMndY-oU0kQ
The copyright belongs to the author. For commercial reprints, please contact the author for authorization. For non-commercial reprints, please indicate the source.