From the current perspective, the emergence of DeepSeek has broken the inherent constraints of computing power and some links in the model, but there are still many problems to be solved, such as the directional distillation of the model, the construction of the data system, and the cross-coordination of the interests of all parties in the ecosystem, etc. This is no longer just a technical proposition, but also an industry proposition for the industry to move forward. However, what is certain is that by 2025, the industrial tide of China's AI big model will be surging and unstoppable.
The emergence of DeepSeek seems to have gradually outlined a definite future blueprint for the practical application of AI.
In the past few years, the entry threshold for large AI models has long been clearly marked – trillions of parameters, super computing power support, and massive and high-quality data resources, all of which mean a high “entry price”.
During the 2025 Spring Festival, DeepSeek was like a dark horse, breaking the original rules of the Chinese and foreign AI large model arena.
This team, originally from a quantitative institution, has drastically reduced the parameters of the large model to 1/10 of the original. With the help of reinforcement learning and model distillation technology, a small model has surpassed GPT-4o in solving math problems. In addition, DeepSeek has also open-sourced its code and API, showing its powerful capabilities comparable to OpenAI at a super low price, which has made netizens at home and abroad marvel at this “mysterious oriental power”.
In a sense, these performances on the surface have certainly shocked the AI industry, but several issues that should be considered over a longer timeline are still the core propositions hovering over the AI industry in 2024: How far are we from the big industrial model? On the three nodes of data, computing power, and models, and on AI applications that almost reached a consensus last year, what impact will this “phenomenal event” of DeepSeek have?
In 2025, the curtain of industrial digitalization has quietly been opened.
1. The technology paradigm has changed, and the model has entered the era of “low price and high quality”
In the process of implementing traditional AI models, there are many problems that limit their widespread application. Among them, “burning money without seeing any hope” ranks first.
Taking GPT-4 as an example, its training data volume is as high as 13 trillion tokens, covering texts in all fields of the Internet. Such a large amount of data labeling is not only costly, but also time-consuming and laborious. At the same time, its demand for computing power is also extremely large, relying on tens of thousands of A100 GPU clusters, and the cost of a single training is more than 100 million US dollars. Such high costs and resource requirements make it difficult to implement the technology.
This is also why DeepSeek is so highly praised, that is, it can achieve “self-evolution” through pure reinforcement learning (RL), giving it a significant advantage in data preparation.
In other words, it does not require labeled data, which greatly reduces the cost and difficulty of data preparation, saving developers a lot of time and energy, allowing them to focus more on model training and optimization.
At the same time, DeepSeek’s reward design is extremely simple, using only “correct answer” and “format specification” as reward signals. This simple reward mechanism avoids the risk of “cheating” that may be caused by complex reward models, making model training more efficient and stable.
This minimalist reward design can also better guide the model in the right direction, improve the model training effect, and avoid some unexpected situations that may lead to deviations in model training.
In addition, DeepSeek uses the GRPO algorithm, replacing the traditional Critic model with group scoring, reducing computing power consumption by more than 30%, further reducing the demand for hardware resources, which is commonly known as dependence on “cards”.
It is worth noting that the model capability has not been greatly reduced due to the reduction in computing power.
In the paper published by DeepSeek, a set of data showed that DeepSeek-R1 achieved a Pass@1 score of 79.8% in the AIME 2024 test, slightly higher than OpenAI-o1-1217. On MATH-500, it achieves a score of 97.3%, performing on par with OpenAI-o1-1217 and significantly outperforming other models.
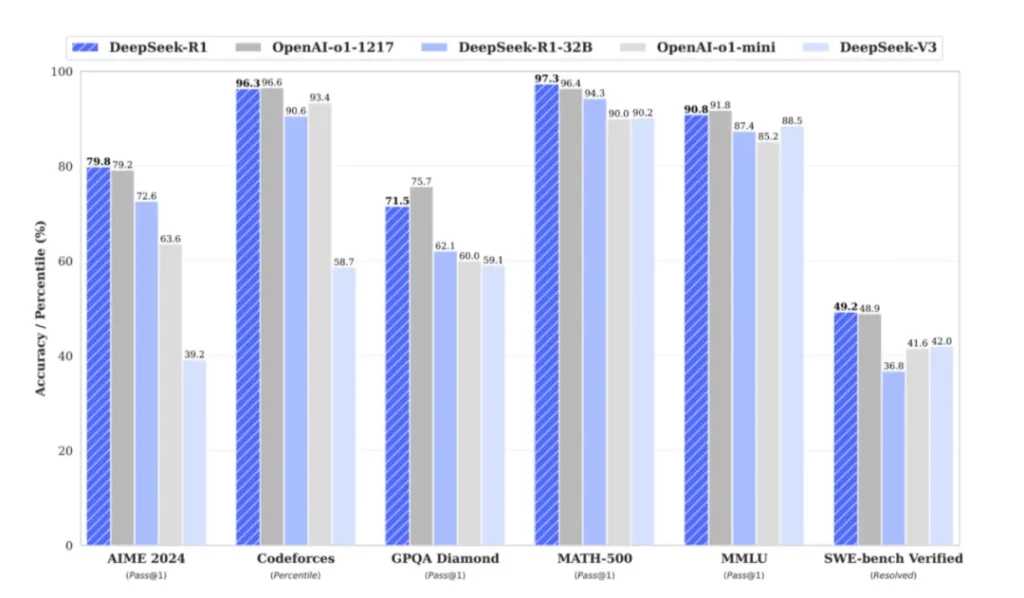
In DeepSeek, the outside world seems to have discovered that computing power and parameters are no longer the entry threshold for AI. Or, to be more precise, in DeepSeek, the outside world has seen a low-threshold, low-cost method that is more suitable for the implementation of AI, which is more conducive to implementation from the cost side.
From the industry perspective, the biggest beneficiaries of this change are medium and large manufacturers. In the past two years, large state-owned enterprises, universities, and people’s livelihood departments have all publicly tendered for projects based on large models. A large part of these projects are pre-training projects, and the unit price of these projects often exceeds tens of millions or even hundreds of millions, as targeted investments by enterprises.
But after DeepSeek, it can be predicted that the targets of this year’s medium and large-scale large-model projects will change significantly. For medium and large enterprises and even central state-owned enterprises, they can deploy large-model projects at a lower cost, or shift their focus more to data governance to further improve the final model effect.
Small technology companies also benefited from this. In the past, they may not have been able to get involved in the AI field due to funding and technical limitations. However, the emergence of DeepSeek has made it possible for them to develop AI applications that suit their business needs based on DeepSeek at a relatively low cost, thus promoting the development and innovation of their business.
In general, with the change of the reinforcement learning (RL) technology paradigm, not only will the threshold and cost of implementing large AI models be reduced, but it will also provide more companies and developers with opportunities to participate in AI innovation. This will not only help promote the development of AI technology, but also provide new impetus for the digital transformation and upgrading of various industries.
2. Open source acceleration: the era of vertical small models has arrived
In the paper published by DeepSeek, in addition to the transformation of the RL technology paradigm, there is another highlight, which is the construction of a cross-dimensional knowledge distillation system.
A set of data shows that DeepSeek-R1-Distill-Qwen-7B surpassed the original QwQ-32B-Preview with a score of 55.5% in the AIME 2024 evaluation, and the performance was improved by 23% while the parameter scale was reduced by 81%. Its 32B version even achieved an amazing accuracy of 94.3% in the MATH-500 test, which is nearly 40 percentage points higher than the traditional training method.
It deconstructs the reasoning logic of the 32B large model into a transferable cognitive pattern, and then injects it into the 7B small model through a dynamic weight allocation mechanism, thus realizing the transmission of “thinking paradigm” rather than simply “knowledge memory”.
Under this technical path, the small model not only inherits the problem-solving ability of the large model, but also acquires the meta-ability of problem decomposition and logical deduction. This also means that the reasoning mode of the large model can be distilled into the small model, and its performance is better than the result of direct intensive training on the small model.
In the field of artificial intelligence, the perception that “the bigger the model, the better the performance” has long been dominant. The evolutionary trajectory from GPT-3 to GPT-4 seems to confirm the law that “parameter size determines model capabilities.”
With the emergence of this combined training method of “distillation + reinforcement learning”, the era of small models seems to be finally coming.
You should know that for many companies, especially small and medium-sized enterprises and vertical professional companies, when pursuing model performance, they are often limited by the huge computing resource costs required for large models.
After DeepSeek proved that small models can also play a big role, these companies can reduce their spending on purchasing and renting hardware equipment (such as high-performance servers, GPUs, etc.) and reduce energy consumption costs.
For example, a small company focusing on medical image analysis may need to build an expensive computing cluster if it wants to use large models to process image data. Now, with the help of optimized small models, it can complete the task on ordinary computing devices, greatly reducing costs.
Among them, under the trend of small model effectiveness, companies with industry awareness usually have a deep understanding of their own business processes and data characteristics, and they are often able to integrate models into existing business systems more quickly.
Because small models generally have simpler architectures and fewer parameters, developers can more easily customize them to meet the needs of specific industries. For example, a financial risk control company, based on its know-how on risk assessment in the financial industry, can quickly embed an adapted small model into its risk control system, shorten the development cycle, and achieve model launch and business optimization more quickly.
In a highly competitive market, this advantage can enable certain companies to quickly overtake others in the field of AI and become the makers and leaders of AI rules in vertical tracks.
3. Efficiency and scenario breakthroughs: the explosive growth of end-side applications is coming
As we all know, in practical applications, especially in scenarios such as edge computing and real-time decision-making, traditional AI models often face many limitations.
In edge computing scenarios, due to limited device resources, such as mobile phones and glasses, it is difficult to run large AI models, which limits the application of AI technology in these fields.
In addition, in real-time decision-making scenarios, such as financial transactions and industrial production, the reasoning speed and accuracy of traditional AI models often cannot meet the requirements.
The emergence of DeepSeek provides a new idea. Its breakthroughs in model compression, inference efficiency, and training cost optimization provide strong support for its application in multiple scenarios, bringing about huge breakthroughs in efficiency and scenarios.
DeepSeek uses model compression technology to make its optimized model better adaptable to devices with limited resources, such as edge computing devices such as smart glasses. This enables edge computing devices to have stronger AI capabilities and provide users with a more convenient and intelligent experience.
For example, in smart glasses, DeepSeek can achieve faster and more accurate image recognition and voice interaction functions. Users can use smart glasses to more efficiently obtain information, navigate, identify objects, etc., greatly improving the practicality and application scenarios of smart glasses.
Its efficient reasoning capabilities also play an important role in real-time decision-making scenarios.
Taking financial transactions as an example, financial institutions need to analyze and process a large amount of market data in a very short time to make accurate investment decisions. It can quickly analyze and predict data, provide real-time decision support for financial transactions, and help financial institutions improve transaction efficiency and profitability.
In industrial production, real-time quality inspection and fault diagnosis are also crucial. It is also possible to quickly analyze the data in the production process, discover quality problems and equipment failures in a timely manner, thereby improving production efficiency and product quality and reducing production costs.
It can be said that in 2025, the emergence of DeepSeek may lead to a new round of terminal application explosion, providing strong technical support for the digital transformation and upgrading of various industries. The application breakthroughs of DeepSeek in multiple scenarios not only demonstrate its technical advantages, but also provide new solutions for the digital transformation and upgrading of various industries.
4. Ecological transformation: large factories refine models, small and medium-sized factories develop applications
DeepSeek also brings about changes in the AI ecosystem, and this change will also bring more possibilities for the implementation of AI in the industry.
The fact is that the current AI industry presents a “pyramid structure”, with giants such as OpenAI and Google controlling the basic models, mid-level companies relying on API calls and falling into “data hollowing out”, and bottom-level small and medium-sized developers lacking customization capabilities and becoming ecological vassals.
The fatal flaw of this structure is the stagnation of innovation. In order to maintain their monopoly, the giants will inevitably limit the openness of the model.
DeepSeek open-sources its core model and opens API customization capabilities, a move that breaks the “pyramid-style” ecosystem previously dominated by giants such as OpenAI.
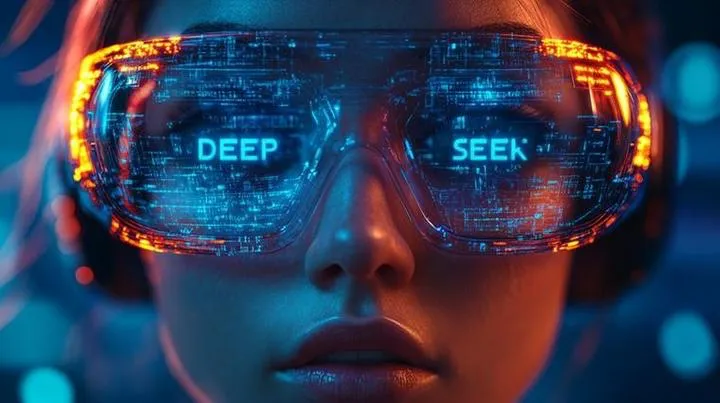
Under the new ecological model, large companies can focus on refining models and use their strong technical strength and resource advantages to continuously optimize and improve the performance and capabilities of the models.
For example, platforms such as Alibaba Cloud and Tencent Cloud can become “model supermarkets” that provide hundreds of small models in vertical fields to meet the needs of different industries and users. These large companies can promote the development and progress of AI technology by launching more advanced model architectures and algorithms through continuous research and innovation.
Small and medium-sized companies can focus on making applications and quickly develop specialized AI tools based on open source models, without relying on giants to provide “black box” capabilities. This provides more development space and opportunities for small and medium-sized companies, allowing them to give full play to their flexibility and innovation capabilities and develop AI applications that are closer to user needs and industry characteristics.
For example, some small and medium-sized factories can fine-tune models on demand through APIs for deterministic needs such as industrial quality inspection and supply chain forecasting, develop efficient and accurate AI applications, and provide customized solutions for users. This ecological transformation also brings many benefits such as technology democratization, ecological positive cycle, and scenario customization.
The democratization of technology can enable non-tech companies such as manufacturing and agriculture to participate in the application and innovation of AI technology, promoting the digital transformation and upgrading of various industries. The positive cycle of the ecosystem can form a collaborative network of “data-model-application” through developers contributing industry data to optimize the model and sharing the model revenue, promoting the sustainable development of the AI industry.
It can be said that the ecological changes brought about by DeepSeek have not only brought new opportunities for the development of the AI industry, but also provided new impetus for the digital transformation and upgrading of various industries. In the future, with the continuous development and improvement of DeepSeek technology, its potential in ecological change will be further released, bringing more possibilities for the development of the AI industry.
5. 2025: New trends of AI
In 2025, the direction of AI application in the industry will become increasingly clear.
In 2025, the development of AI will gradually shift from the past pure worship of technology to a more pragmatic application that focuses on business. This change is reflected in many aspects such as technology research and development, commercialization path, and ecological alliance construction.
In the field of technology research and development, companies have gradually realized that blindly piling up model parameters is not a wise move. Models with a scale of hundreds of billions are not a panacea, and the success of DeepSeek – R1 has strongly proved that models with a scale of tens of billions can also be comparable to larger models through algorithm optimization.
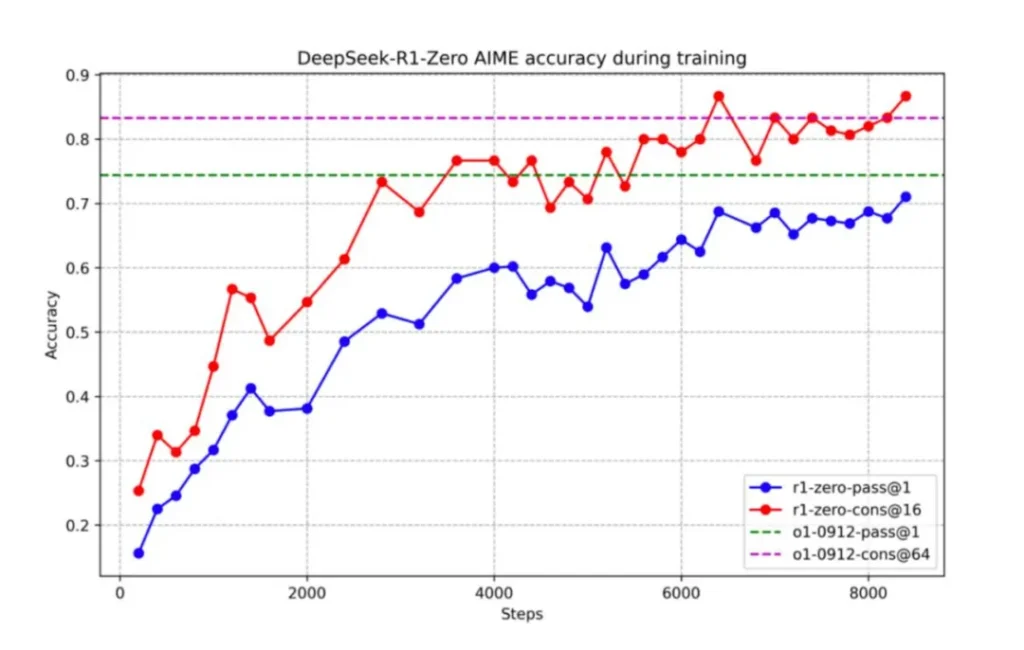
Therefore, future R&D investment directions will focus more on reinforcement learning (RL) and model distillation techniques.
Compared with simply increasing the amount of data, RL’s self-evolutionary capabilities and the ecological value of distillation technology show greater potential in commercial applications. Through these technologies, companies can improve model performance while reducing costs and expand their application scenarios, thus embarking on a cost-effective path of integrating AI with business.
In terms of choosing a commercialization path, the B-end market has become the priority focus.
Cooperate with leading companies in various industries, such as automobile companies, hospitals, banks, etc., to jointly build industry-specific models and adopt a pay-per-performance model. This will not only achieve a deep binding between enterprises and customers, but also promote collaborative cooperation between the two parties in value creation.
At the same time, companies should not ignore the potential market demand of small and medium-sized customer groups. By providing open source models and low-code platforms, and providing these customers with convenient “AI capability containers”, they can effectively reduce customization costs, meet the diverse needs of the long-tail market, and thus achieve comprehensive coverage of the entire market.
Building an ecological alliance is also crucial for the development of an enterprise.
On the one hand, open source core frameworks, such as DeepSeek’s open RL training toolchain, can attract developers to actively participate in ecosystem construction, pool the wisdom and resources of all parties, and form a powerful technical synergy.
On the other hand, the establishment of cross-border alliances is also essential. By uniting chip manufacturers (such as Huawei), cloud service providers (such as Alibaba Cloud) and professional companies in vertical fields to form a “computing power-model-scenario” iron triangle cooperation model, it can promote collaborative innovation in the upstream and downstream of the industrial chain and create a win-win industrial ecological environment.
Judging from the current industry situation, although it is difficult for China’s AI big models to surpass OpenAI in general capabilities for the time being, there is every opportunity to achieve a differentiated breakthrough through intensive work in vertical scenarios and open cooperation in the ecosystem.
Looking ahead to 2025, the development goal of China’s AI industry is to create a number of “small and beautiful” industry models. These models will have local advantages over the “big and complete” models of the West in specific fields, and through in-depth application and optimization in specific industries, they will gradually penetrate and expand into the field of general intelligence.
This development path can not only give full play to China’s industrial advantages in specific fields, but also provide an innovative model and solution with Chinese characteristics for the development of the global AI industry, and promote the diversified development and application of AI technology on a global scale.
In conclusion:
DeepSeek’s technological innovation and ecological openness have transformed AI from a “game for giants” to a “joint creation by all. ” With the mutual catalysis of digitalization and AI, a flywheel of “the more popular the technology, the richer the data, and the smarter the model” has been formed.
However, we should be more cautious about the implementation of industrial AI. Although the emergence of DeepSeek has broken the inherent constraints of computing power and some links in the model, there are still many problems to be solved, such as the directional distillation of the model, the construction of the data system, and the cross-coordination of the interests of all parties in the ecosystem. This is no longer just a technical proposition, but an industry proposition for the industry to move forward.
However, what is certain is that by 2025, the industrial tide of China’s AI big model will be surging and unstoppable.
Author:斗斗
Link:https://mp.weixin.qq.com/s/NBqLdUdbdmVCm54UULDYeQ
Source:产业家
The copyright belongs to the author. For commercial reprints, please contact the author for authorization. For non-commercial reprints, please indicate the source.