Mercor is an AI recruitment startup founded by three 21-year-old Thiel Fellows. It is one of the fastest growing companies in the technology industry. In September last year, the monthly ARR growth rate reached 50%. So far, the company has achieved a breakthrough of $75 million in ARR in 2 years, with the main revenue coming from AI labs including OpenAI. Mercor recently completed a $100 million Series B financing, with a valuation of $2 billion.
Mercor is a platform that connects candidates and recruitment companies. After candidates upload their resumes, Mercor will screen suitable talents through AI interviews. After the recruitment company describes its requirements, Mercor will automatically recommend suitable candidates, automating the entire recruitment process. The product experience is very good and the recruitment success rate is high.
This compilation is an interview with Adarsh Hiremath, founder and CTO of Mercor, by 20VC. Adarsh believes that data annotation and talent assessment are actually the same thing, and the bottleneck for releasing the next generation of intelligence is expert human data, not synthetic data. In the future, powerful domain-specific models will emerge, unlocking a large number of application scenarios in different industries. With the development of Coding Agent, application construction will become simpler, and only companies with network effects will survive better.
01.
What is Mercor?
Entrepreneurship and Growth
• Origin of the business
Mercor’s three founders are Brendan Foody (CEO), Adarsh Hiremath (CTO) and Surya Midha (COO). The three knew each other for a long time before starting their business. Adarsh first met Surya when he was 10 years old. The two of them hit it off very well because they were the only two elementary school students who wanted to participate in high school debates. Eventually, the two entered the same high school and met Brendan in high school. The three of them became debate partners and participated in many national debate competitions.
Adarsh likes to think of the debate partnership as his first entrepreneurial venture, and believes that debate and entrepreneurship are similar in many ways, such as choosing the right partners and having a huge sense of responsibility.
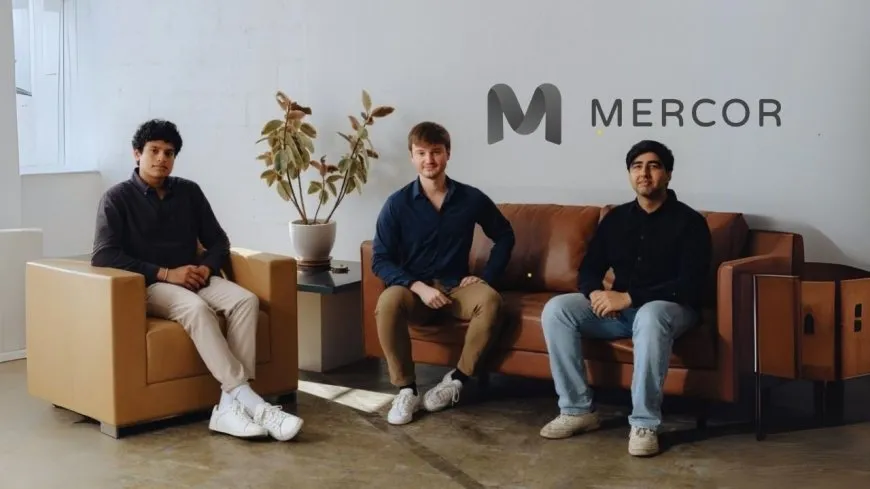
From left to right: Adarsh Hiremath, Brendan Foody, Surya Midha
When the three started working together, they didn’t have any business plan. They just opened a development studio together, wanted to quickly learn how to develop software to help startups, and eventually recruited some outstanding talents from India. But they soon realized that what really matters is people, not software. The three founders found these people manually, so they began to think about whether this process could be automated. This was the beginning of Mercor’s automation of candidate-facing functions. Then, they realized that if companies needed to manually screen candidates, this would not be scalable, so they had to automate company-facing functions as well. Thus, Mercor was born.
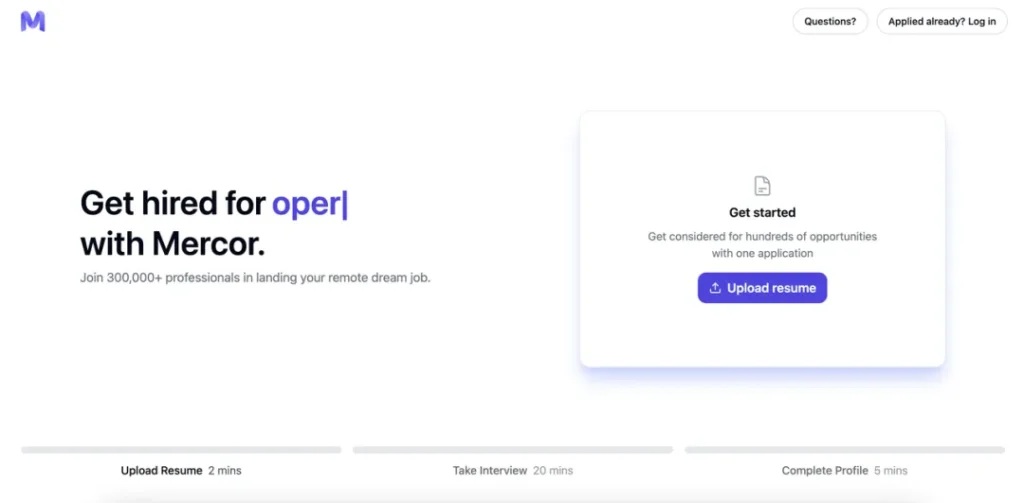
For candidates
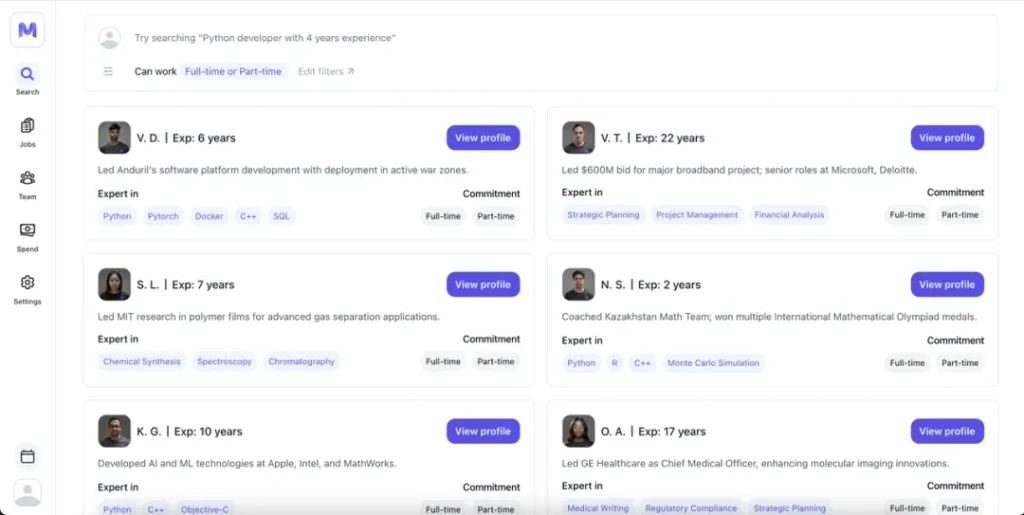
For recruiting companies
Adarsh believes that recruitment is the most prestigious position in a company, because recruiters control the inflow and outflow of talent in the company and can almost understand everything about a company through the flow of talent. Therefore, the recruitment function of a company is the most underestimated and neglected part, which is one of the reasons for creating Mercor. At the same time, the team believes that higher recruitment efficiency should be achieved with less manpower. This view is complementary to the importance of recruitment, because only when the right talents can we talk about improving efficiency. Solving the matching problem, that is, finding the right talents, is very difficult, especially with manual processes that cannot be scaled. As time goes by, Adarsh becomes more and more aware that the next generation of SaaS will replace end-to-end services. This realization is also one of the reasons for establishing Mercor to some extent.
• Dropped out of school to start a full-time business
Adarsh had basically avoided classes as much as possible while attending Harvard. Mercor hadn’t raised seed funding, had little revenue, and the founders didn’t have Thiel Fellowships, but Adarsh wanted to work with his best friends.
Adarsh believes that although you can try to rationally analyze whether you should drop out or start a business, it is often an emotional decision. Adarsh finally decided to drop out of school and start a business full-time when the three of them had an office in Palo Alto.
• 996 work
The Mercor team works 996 hours (9am to 9pm, 6 days a week). Adarsh tends to see 996 as a side effect of selecting team members, rather than a goal. When Mercor selects team members, it is very careful to select people who care deeply about Mercor’s mission. The side effect is that employees don’t want to wait until Monday to start moving the company forward. Everyone enjoys each other’s company and what they are doing.
To some extent, this is not unique to Mercor. All successful companies throughout history have had a fairly strong culture, which is a characteristic of startups, where you have to work harder than others in a sustainable way to succeed. This drive is very contagious, and everyone in the Mercor team feels energized.
• Rapid growth
Mercor has achieved 50% growth for several consecutive months, which Adarsh believes is a continuous stress test for Mercor. Many things will continue to go wrong during the growth process, including workflows, recruitment, etc. Most importantly, everyone in the team needs to constantly surpass themselves, constantly redefine what they can do, and take on new roles.
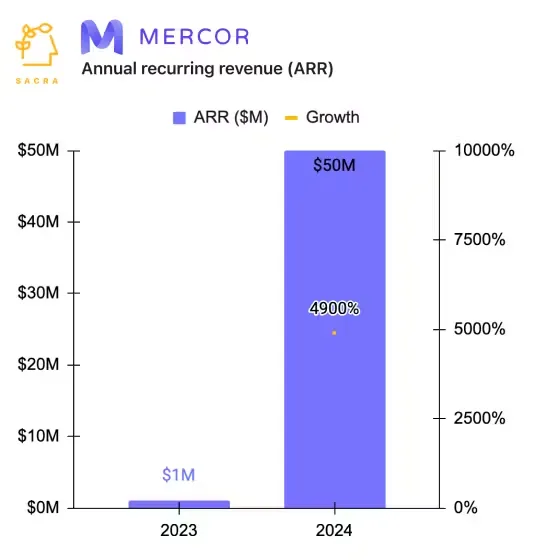
Mercor ARR
Adarsh believes that when a company is growing rapidly, it is more difficult to expand culture than software. The culture created by the founders in the first 20 people may be the strongest culture in some ways, but it is challenging to ensure that this culture remains strong as the company grows, does new things, and new members join. But in a way, this is also the most important part of building a legendary company.
When considering the ultimate market size of Mercor, you first have to consider that there are hundreds of millions of job seekers, and then consider how many jobs each person will change. Ignoring all the jobs Mercor has created for AI agents and focusing on jobs created only for humans, Mercor has created perhaps dozens of positions for each person. Mercor has created hundreds of billions of jobs and built a unified labor market, which means that any company that wants to recruit employees for a specific position or task can do so through Mercor. And any job seeker who wants to consider a specific position or task offered by a company can also do so through Mercor. Mercor can seamlessly solve matching problems across companies and positions.
Features
• Focus on product experience
Mercor’s entire recruitment process is automated, from the moment a candidate hears about Mercor and enters the Mercor platform through a job listing, Mercor pulls the candidate’s resume and salary expectations, conducts a personalized interview based on the candidate’s background and job requirements, until the candidate is paid for their work.
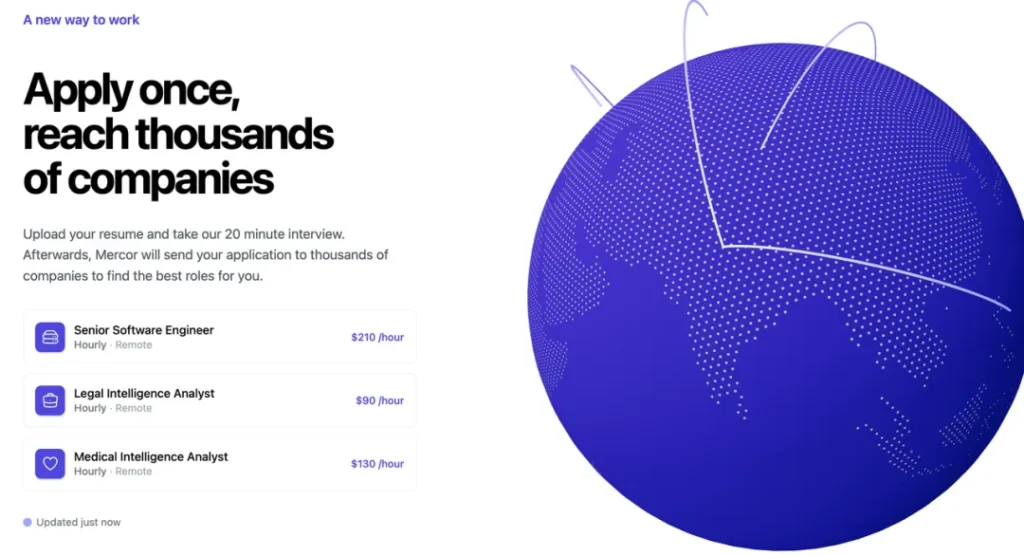
Mercor Automated Application
Adarsh believes that some of the most successful products or companies today have very sticky revenues because their products can create a “six-star” experience, which is one of the reasons why Mercor’s revenues are growing so quickly.
Previously, the Mercor team believed that chat was the core of all future UIs, so the initial version of Mercor was built around the chat interface. At that time, talent could only be hired through Mercor’s chatbot. Later, the team realized that although the chat interface was important, it also needed to be used in combination with other methods.For example, combining LMS (Learning Management System,Learning Management System) and other tools. The team believes thatIn the future, web applications will disappear, and all web application interactions will be done only through chat. Users don’t need to click a button to hire someone, but directly tell the chatbot to hire that person. Adarsh thinks this may be possible in the future, but the team chose a little too early at the time.
• High recruitment success rate
Adarsh believes that whether a recruitment is successful depends entirely on the talent required for the position, which is a very position-specific process. Different companies value different things, and based on the factors they value, Mercor can adjust the talent forecast.
Mercor provides companies with a wide range of talents, covering positions ranging from software engineers to lawyers, doctors, financial analysts, consultants, and more. An important feature of Mercor is that it is not tailored for a specific position, but rather builds technology that can be used universally. For example, in AI interviewers, the Mercor interviewer system can immediately process the candidate’s background information and then provide a customized interview. No matter what position the person wants to apply for, the interview system can be started in less than 10 seconds. Adarsh believes that it is possible for an agent to automatically collect information about a person and prepare an interview that exceeds the human level, and this can be done for almost all positions.
Currently, clients continue to expand their partnerships with Mercor, with a net retention rate well over 100%. As long as clients continue to expand their relationships, it means Mercor is doing a good job of finding the right talent.
• Improve products with AI
The landscape of AI underlying models is changing very quickly. Mercor has used many models and is very satisfied with OpenAI’s model. To some extent, he has been using OpenAI’s model.
If improvements can be made in some aspect of the underlying large model, it will bring improvements to the business and products. For example, in the case of AI interviewers, when the model improves, the applicant experience on the Mercor platform will also improve significantly.
Adarsh said Mercor’s focus has always been on whether Mercor can continue to improve its products as the model becomes more powerful.
Business Model
• Sales & Pricing
Mercor currently has no sales team. Apart from the founder, no one is dedicated to sales. The current customers who sign contracts are mainly contacted by customers. Someone has hired talents through Mercor, heard good things about Mercor, and then contacted Mercor, and then continued to cooperate.
Adarsh believes that clients are often most concerned with quality, not price. If Mercor can reliably find the top 0.1% or 1% of talent for the cost of the software and keep clients happy, then how much to charge is often a secondary concern for clients.
When a user gets into an Uber car, there isn’t much difference between a 4.8 star driver and a 4.9 star driver because their jobs aren’t that different. But in Mercor’s scenario, there’s a huge gap between the top 0.1% and the top 20%. When Mercor can find hundreds of times more talented people at the cost of the software, clients feel compelled to use Mercor. It’s usually when the first few candidates start working with a client that clients realize the value of Mercor.
Fees are determined on a case-by-case basis. For some clients, Mercor’s fee may be more than 30%, while for others, it may be less.
• Started in India, but the largest market is the US
Mercor started with Indian users because the parents of Mercor’s founders immigrated to the United States from India, and Mercor chose to start its recruitment activities from the schools they attended.
What prompted Mercor to start with Indian users was that Mercor once found one of the best engineers in the team through Facebook ads. But at that time, Adarsh personally interviewed him, but he did not pass the interview. However, he sent Adarsh a long message, telling Adarsh what went wrong in the interview and how to correct it, so Adarsh thought he met Mercor’s standards, and Mercor paid more attention to the employment problems of Indians in the labor market.
Currently, the largest proportion of workers who find jobs through Mercor are from the United States, accounting for about 60%. Customers are also mainly American customers.
• Collaboration with top AI labs
According to TechCrunch, Mercor has cooperated with the world’s top five AI labs, including OpenAI, and many large AI labs also recruit through the Mercor platform.
For many labs that Mercor works with, Mercor is able to identify people who are outstanding in specific fields and have them work with the labs, which is actually the driving force behind Mercor’s long-term goal. Mercor wants to build a global unified labor market, which requires a large number of smart people to join, and needs to be able to predict their work performance and find out what they should do.
Financing
• Seed round
💡 In 2023, Mercor completed a $3.6 million seed round of financing led by General Catalyst, a well-known American venture capital firm.
The three founders were only about 19 years old when they raised their seed round. They moved their office to New York before raising funds. For Adarsh, the moment that was more unbelievable than the arrival of the seed round funds was when they adjusted their salaries to $500 per month on Gusto (Note: a payroll company in the United States). Mercor then completed its seed round of financing, and the financing progress was very fast.
• Series A
💡 In September 2024, Mercor completed a $32 million Series A financing led by Benchmark, with a valuation of up to $250 million. Many heavyweight individual investors also participated in this financing, including "Silicon Valley Investment Godfather" Peter Thiel, Twitter co-founder Jack Dorsey, two directors of OpenAI, Quora CEO Adam D'Angelo, and former US Treasury Secretary Larry Summers. Benchmark's new partner Victor Lazarte became a member of Mercor's board of directors.
Mercor did not plan to raise funds at the beginning, and the arrival of funds seemed to happen naturally. In the cooperation with Benchmark, Victor persuaded Brendan to have a preliminary communication, and then Peter Fenton, one of the partners of Benchmark, invited Brendan to take a helicopter ride. Later, Brendan talked with Victor and the Benchmark team several times.
• Series B
💡 In February 2025, Mercor completed a $100 million Series B financing round led by Felicis, with a valuation of $2 billion.
The B round of financing was about six months after the A round. At that time, Mercor was not focused on financing, and its business revenue had reached tens of millions of dollars. The team wanted to continue to focus on work, but wanted to cooperate with Felicis, so they chose financing. In terms of dilution ratio, 5% of the equity was diluted to obtain 100 million in funds.
Although the financing went smoothly, the three founders of Mercor enjoyed driving the business forward the most. When the financing was raised, the team did not fully realize that the growth would be so rapid. The team knew there would be growth and was confident in the growth, but the growth rate still exceeded expectations.
Adarsh believes that when people raise money, it is dangerous to think that they have to spend it immediately. Mercor’s goal is not to spend the $100 million immediately. Mercor’s goal is to build a unified labor market, which takes a long time, so Mercor just wants to ensure that there are enough funds in reserve to cope with long-term development.
02.
What does Mercor think of AI?
Human Data Annotation vs Synthetic Data
Some investors in the market believe that Mercor is mainly doing data annotation for basic models, but Adarsh believes that data annotation and talent assessment have actually become the same thing. Five years ago, when it came to data annotation, it was basically a crowdsourcing problem. Suppose Waymo wanted some image annotations, it would ask people all over the world to draw frames and mark parking signs so that the model could better classify parking signs. But today, the nature of data annotation work has changed a lot. GPT-4 or other models may not work well in a specific field, so experts in a field are actually needed to make the model better in that field. Finding out who is an expert in this field is actually 100% a talent assessment problem, which is the perfect application for Mercor.
Adarsh believes that in the future, a lot of data will be human-annotated data rather than synthetic data. Take Eval as an example. Eval must be created with human data because in order to judge how well a model performs in a specific task, there must be a human-created test set that outperforms the model on that task. Humans play an important role in this process. In addition, methods such as SFT, RLHF, and RL, which train next-generation models, all require expert-level human data annotation to help improve the model.
Many people, including Jonathan, CEO of Groq, believe that synthetic data is of higher quality because it avoids the interference of low-quality data on the Internet, so using high-quality synthetic data instead of low-quality human data will exponentially improve model performance. But Adarsh believes that this view is wrong. Synthetic data and human data are not a zero-sum game. Even in a world where human data is critical to the next generation of models, it does not mean that synthetic data is not important. But in many cases, the bottleneck to unlocking and releasing the next generation of intelligence is still expert human data. Low-quality human data will definitely not make the model better, but high-quality human data can do it. This is exactly a talent assessment problem. The key to improving data quality is to find the right people, which is very difficult to do.
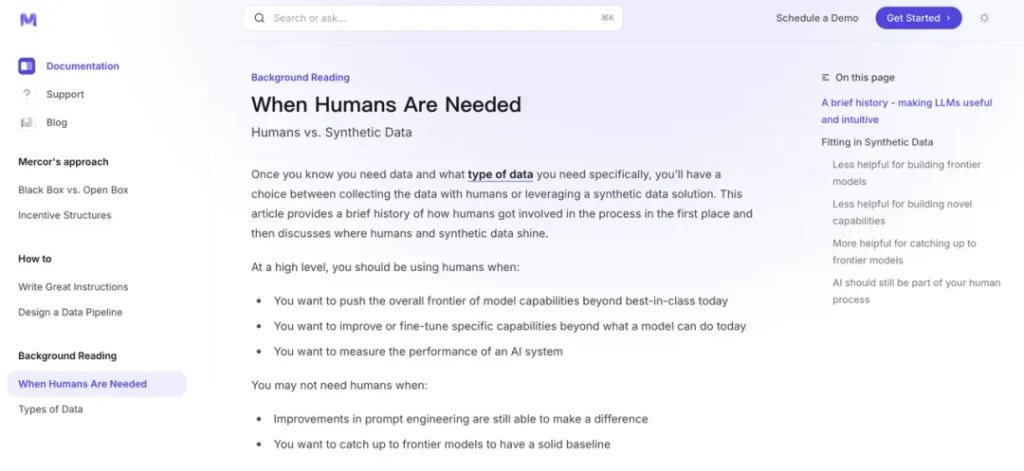
Humansvs SyntheticData
Adarsh believes that data is a bigger bottleneck limiting model improvement than computing or algorithms, but computing and algorithms are also part of the puzzle. Computing, data, and algorithms will all play a role in driving AI progress and unlocking the next generation of intelligence. But we are entering an era where expert human data is really needed to make models better in specific application scenarios. This situation will continue for a long time because there are still many tasks that models cannot complete.
AI Model &Agent
• FoundationModel
In the next generation of models, the entire market is turning to reinforcement learning, and the Mercor team has seen this trend in the models of o1, o3 and DeepSeek. Adarsh believes that in the future, there will be very powerful models in specific fields that can perform extremely good reasoning, which will unlock a large number of use cases in different industries and fields.
Adarsh believes that the future will enter a world where multiple models serve different use cases. There are already many application-layer companies that are using these specialized models to solve their own problems. For Mercor, the focus area is recruitment, and Mercor’s capabilities in recruitment far exceed those of traditional expert recruitment managers. For other companies, it may be financial analysis in a specific field. So, in these different application scenarios, each company needs to improve the performance of the model according to its own needs. In the end, there will be a few companies that can build basic models for other companies to develop based on the basic models, such as OpenAI. There will not be 20 companies that can build basic models and be widely used like OpenAI.
• Coding
There is a view that many young talents are told that they should not study computer science because computer science is becoming more and more automated. Now 41% of the code is written by AI. In five years, this proportion will increase significantly. Adarsh’s view is that programming is more important today than ever before. Programming will just happen in different forms. The jump from assembly language to Python may be greater than the jump from Python to natural language. The way programming is defined in the future will be very different. Perhaps people with only general skills in computer science today will be able to command thousands of super coding agents to do things that were once thought impossible. Programming in English will become very important.
Adarsh now uses a lot of AI tools when programming, such as Cursor. Many members of the Mercor team are using Cursor, which makes the work that originally took a lot of time to complete very simple and elegant. For example, in testing, with the help of a few prompts, a more thorough test suite can be generated for the application than anyone could imagine, which was not possible before. For example, if the user wants to bring the style of one part of the code base to another part, it can also be easily done in Cursor. Adarsh believes that as Coding Agent becomes more and more powerful, software will soon become commoditized.
The commoditization of software means that people will be able to build applications faster than at any time in history. It also means that in a world where the cost of software is close to zero, only those companies that can successfully build on network effects will survive, even if they give away their entire codebase for free, like Meta and Airbnb.
• AGI
Adarsh believes that when AGI is realized or thought about, more economically valuable work will be involved. When more and more economically valuable work is automated, research will also be automated to some extent, which also falls into the category of AGI.
Future Development
• The future labor market
If in one or two hundred years, the model can complete all the work and humans no longer need to work, then society will become completely different. All humans will live a life with universal basic income (Note: universal basic income means that there are no conditions, qualification restrictions, or qualification reviews. Every citizen or member can receive a certain amount of money on a regular basis, which is distributed to all members by the government or group to meet basic living conditions), play video games every day, or do other things.
But before that, there will be many tasks that the model cannot complete, which may be tasks with specific economic value, such as consulting work or some kind of engineering tasks, or even more niche tasks, such as making the model better at a specific hobby. Humans will need to fill these gaps, especially in the “long tail” areas. Many people have a one-way understanding of the relationship between humans and AI, that is, things that humans cannot do are left to AI to complete, but the more realistic situation is that for specific application scenarios, AI may be able to help humans complete 60%, 70% or 80% of the work, but the remaining 40%, 30%, and 20% still need to be completed by humans. Finding humans who can complete these remaining parts will become more and more difficult, and therefore more valuable.
The future labor market will move toward specialization and complexity, which means that the jobs we see in the next 50 years will be more specialized and generally require people with higher levels of expertise.
• The importance of network effects
As mentioned above, as Coding Agent becomes more powerful, the software will gradually become commoditized., only companies with network effects can survive better.
Today, Mercor’s network effects can be divided into two categories. The first is the network effect in the labor market, like Uber or Airbnb, where each additional company that recruits through Mercor or candidate on Mercor increases market power because there are more great people and companies to choose from. The second is the data flywheel around job prediction, where Mercor can see which people perform well in their jobs and know the specific reasons why they perform well, and use this end-to-end data to help Mercor find the best person for a position, even if they themselves may not know this.
Author:海外独角兽
Source:https://mp.weixin.qq.com/s/ftTh0SiVlYhacXvunl_HwQ
The copyright belongs to the author. For commercial reprints, please contact the author for authorization. For non-commercial reprints, please indicate the source.